Building Trustworthy AI with Alexandra Ebert, Chief Trust Officer at MOSTLY AI
Alexandra is an expert in data privacy and responsible AI. She works on public policy issues in the emerging field of synthetic data and ethical AI. In addition to her role as Chief Trust Officer at MOSTLY AI, Alexandra is the chair of the IEEE Synthetic Data IC expert group and the host of the Data Democratization podcast.
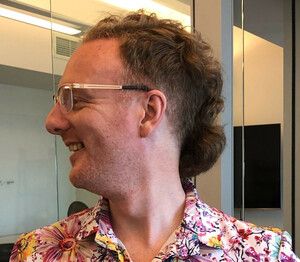
Richie helps individuals and organizations get better at using data and AI. He's been a data scientist since before it was called data science, and has written two books and created many DataCamp courses on the subject. He is a host of the DataFramed podcast, and runs DataCamp's webinar program.
Key Quotes
Many organizations go with, okay, again, more regulation. This is going to hurt our business. But particularly in the context of responsible AI, we have seen so many examples where organizations were simply wasting money because they haven't taken care of fairness or explainability right from the start of the project.
When we think about getting a wrong answer from AI,I think it's important to first ask the question, in which area for which purpose do I want to use the AI system? Is it an area where I can live with this wrong answer? And if the answer is yes, then you can proceed with putting AI into this context. If the answer is no, then don't use it. Also, I think it's very important that organizations educate the users of their AI systems about what to expect.
Key Takeaways
Incorporate Trust Early in AI Development: It's crucial to consider fairness, privacy, and explainability at the beginning of AI project development, not as an afterthought, to avoid costly mistakes and ensure trustworthiness.
Prioritize Transparency and Explainability: Develop AI systems with clear explanations of their decisions, understandable to both users and regulators. This involves showing what data goes in, how decisions are made, and the overall purpose of the AI system.
Educate All Organization Members on Responsible AI: Promote AI and data literacy across the organization, ensuring that everyone understands the ethical implications and challenges associated with AI, including fairness, privacy, and transparency.
Transcript
Richie Cotton: Hi there, Alexandra. Thank you for joining me on the show.
Alexandra Ebert: Thank you for having me, Richie. I'm very much looking forward, on being on DataFramed.
Richie Cotton: glad to have you here. So, to begin with I want to talk about why trust is important. So can you give me some examples when AI has been used and then trust has been breached?
Alexandra Ebert: There are a lot of examples. So maybe the first one that comes to mind was back then when Apple decided to publish a credit card together with Goldman Sachs. And then there were some accusations that this card was actually discriminating against women and granting, in the specific case, a wife less credit than the husband, even though she had more financial assets.
And in a kind of legal process, this was then deflected. But in the end, this was something that impacted the trust users had in the AI system that was used, or also Google, who used the labeling technology to label photos that users of Android took. And then suddenly some users figured out that their black skinned friends were labeled as gorillas, which is something that they couldn't resolve for plenty of years and therefore also heavily impacted the trust many had in brands like Google of not being able to solve something like that.
Richie Cotton: It does feel like there are far too many examples of this where AI seemed like a good idea and then something's gone wrong. It's caused like even the best, it's just like a public relations d... See more
Like why do people lose trust in AI? What are the common themes here?
Alexandra Ebert: The examples that I just named, we had discrimination, so one pattern is definitely biases and discrimination of AI. Another one, privacy infringements, if users suddenly figure out that some data was used to train an AI system that wasn't allowed to use. Or explainability aspects, when you as a user, for example, are denied credit and you can't get sufficient explanation of why this decision was made, which rids you of the chance to challenge the decision made by an AI.
Richie Cotton: Okay. So it's like, it's getting wrong answers or being unfair in some way, or there's some lack of transparency, which is causing a loss of trust. So it just seemed like there are a lot of sort of these important points that we're going to have to get to in more detail later in the episode, I think.
Alexandra Ebert: Absolutely. And maybe also to add to that, I would say how people lose already has the prerequisites that people trust AI. But I think today we're in this strange stage where one bunch of the population has this overinflated expectations and belief that AI can do everything. And the other one is rather mistrusting of AI.
So I think it's also still a process of building up. trust in AI, where AI deserves this trust, and then cautioning in the areas of where AI maybe shouldn't yet be used or just with the necessary precautions.
Richie Cotton: Okay. Oh, it's like, yeah, type one and type two errors. Like you're trusting it when you shouldn't, or you're not trusting it when you should. Interesting. Okay, cool. So, I think related to this what are the costs or the consequences when people do lose trust in AI?
Alexandra Ebert: So I'm going to answer this in two aspects because I work a lot on the regulatory side, advising regulators. And I would say on this macro level, we see that plenty of nations or economic unions like European Union have this objective of becoming a global leader in artificial intelligence. But this is something that will absolutely not happen without people trusting AI, because without trust, there's not enough adoption and not enough organizations developing, building or using AI.
And this is why we also see on a regulatory side, so many initiatives happening like the European AI Act, like the blueprint for responsible AI from the White House and so on, because Responsible AI or trustworthy AI, these two terms often times used synonymously, are seen as the basis to ensure that this trust is here and that we can reach this goals of becoming leaders and having more widespread adoption.
So I think this is the answer on the macro level. If we bring this down to the business side of things, of course, if people are not trusting AI, this could mean that you as an organization have. Problems with bad press or even legal repercussions if something goes wrong with your AI or on the employee side that you will never ever manage to get AI from this POC and piloting and innovation stage to actually having fully fledged AI in production because if people are skeptical and not trusting the way how you use AI, then I'm sure there will be big blockages on the people side.
And I think we all know that bringing AI to an organization requires a lot of change management, a lot of people involvement and approval. And here, again, trust is just simply essential.
Richie Cotton: That's actually a really fascinating point that you think regulation can increase adoptions. I think a lot of the times companies go, Oh, well, you know, regulations is something we comply with. We don't want more of it, but actually, if you have bit of regulation to encourage trust, that's going to help people be more confident.
They're going to adopt AI and, everyone's happy.
Alexandra Ebert: You're completely right. Many organizations go with, okay, again, more regulation. This is going to hurt our business. But particularly in the context of responsible AI, we have seen so many examples where organizations were simply wasting money because they haven't taken care of fairness or explainability.
to you right from the start of the project. One example was Amazon who was thinking of using AI to help them make their hiring decisions, whom to invite for an interview. And here it took them two years until they figured out, okay, this model is actually discriminating against female candidates. We can't bring this into production.
If they had thought about this right from the beginning and figured out ways to mitigate this discrimination, then not as much money would have been wasted and it would have been potentially much quicker in bringing out the product.
Richie Cotton: As soon as we had a recent episode where this same thing with Amazon was discussed and there the guest was sort of saying, okay, well, I'm doing quite well because I actually found out there was a problem and then they shut the system down. But you're saying that actually they should probably have thought about this from the start and built trust into the decision making from the beginning.
Alexandra Ebert: Absolutely, I think with everything in the realms of responsible AI, trustworthy AI, ethical AI, it really pays off and it's also necessary to think about these things right from the beginning before you even decide is AI suitable for this problem or not. So you really have to have it in there from the beginning throughout the entire life cycle.
Richie Cotton: I'd like to talk a bit about accuracy and what happens when AI gets the wrong answer because it's true sometimes AI does come up with a result that's either unexpected or just downright wrong. So, can you talk a bit about what are the consequences of when you get that wrong answer?
Alexandra Ebert: That's a good question because it depends on in which context, particularly, I would even say that you rarely get an answer from an AI where also from a fairness context, it's accurate and fair in all respects, because particularly with fairness, we have these different mathematical definitions, and you can't satisfy all of them at the same time.
So that's one thing to keep in mind. But particularly when you think about getting a wrong answer, I think it's important to first ask the question in which context. area for which purpose do I want to use the AI system? Is it an area where I can live with the wrong answer? And if the answer is yes, then you can proceed with putting AI into this context.
If the answer is no, then don't use it. Also, I think it's very important that organizations educate the users of their AI systems about what to expect. Let's take ChatGPT as an example. If I'm as a user who isn't well acquainted with the inner workings of ChatGPT, assume that this is some type of crystal ball, then potentially the outcome for the organization and also for the user will be quite surprising.
Because the user didn't expect that ChatGPT doesn't always tell you the truth. So I think it's really important to question in which context you can use an AI system and also whether the people using the system have the necessary understanding of what the limitations of the system are.
Richie Cotton: It's always a shame when it's like, oh, it's really context dependent. You actually have to think about what you're doing in order to come to a sensible
Alexandra Ebert: of course, would be more satisfying if I could give you the three easy areas and steps what to do.
Richie Cotton: Yeah. So you said something interesting that there are different metrics for fairness and you can't satisfy them all at once. Can you maybe elaborate on that? Tell me like what some of the different metrics for fairness are and when you might consider one over the other.
Alexandra Ebert: So, from a mathematical side, there are plenty of different definitions, and I think for this episode, it's not as important to go through the detailed metrics, but more give the audience a more general understanding of what the challenge is, and the problem with fairness is, It is not a black and white thing.
It's not either fair or not, but if you ask five people, you will get seven different understandings of what counts as fair. So what I oftentimes like to use as an example is my imaginary nephew and my imaginary niece. Let's assume he's six years old and my niece would be three years old. And if I have six pieces of chocolate, he could, might argue, well, I'm the bigger one.
I should get four pieces. My little sister only should get two. You could say that's fair to some point. She might say, well, I did the dishes yesterday while he was playing computer games. I should get four, he should get two. Also a valid point. And then there would be others who say, well, three pieces for you, three pieces for him.
That's fair. So you see there are already many different things and you can't satisfy all of them at once. And therefore, it's super, super important to keep this in mind, particularly because as a data scientist, you will always. stand in front of this challenge to satisfy two different general concepts of fairness.
Because if we look into anti discrimination laws, what we find there is on the one hand, the principle of trying and aiming to treat everybody equally, not discriminating, for example, based of gender. But then there's also this acknowledgement that historically speaking, we have this history of prejudices, biases, discriminating against people on gender, ethnicity, sexual orientation, and so on.
And here to not perpetuate these biases, it's sometimes important to make an effort of what is called positive discrimination. for example, giving preferential treatment to people from the African American ethnicity or to females or something like that. And as you can see, you can't have equal treatment of everybody and course correcting historical injustices, and therefore it's sometimes really tricky.
And this is also why I oftentimes make this point that this decision shouldn't rest on the shoulders of data scientists alone. It's really a super important, not only societal problem, but also problem from organization. And therefore I think it's important that not only the data scientists, but also legal experts, even ethnicities, social scientists come together to decide how can we approach this in a given scenario.\
Richie Cotton: Okay, so, it just sounded like it's going to be a real sort of cross team effort in the measure of fairness and then implement that as well.
So that sounds like it might be something that's quite difficult to communicate to users and make sure that all your customers have sort of trust in what you're doing here.
How do you message this fairness? Do your customers and explain what you're doing.
Alexandra Ebert: That's a great point. So on the more general level, we've seen many organizations coming out with their own ethically I responsibly I principles, however, they're going to call them here. Of course, it's super high level, and I think in nearly everyone you will see. We take fairness and non discrimination as a crucially important point.
Therefore, I think it's also important to add up to this and show how you're actually doing this by giving a sort of explanation also in the context of AI transparency, if whenever you use an AI system that users are exposed to a general. explanation of what the purpose of the system is, which data points will be used to come to a decision, which training data was used, how you counteracted potential biases, how you observe this.
And then of course, how to make this in an easily digestible question or in a. And then, of course, how to make this in an easily digestible way is one of the open research challenges where, unfortunately, again, I don't have the three points answer that everybody is looking for
Richie Cotton: Okay, but it just sounds like just having some sort of system in place to try and measure your level of fairness and being able to at least show the methodology or report on some things to use that's going to go a long way to making them trust that you are at least trying to. Be fair with your AI.
Does that sound right?
Alexandra Ebert: two points here. So I would argue it's not only about measuring because then data scientists oftentimes like to go to the mathematical side of things. things, look for some metrics to use and then make the check mark that the system is fair. But unfortunately it doesn't work like that because if you haven't looked into how certain data was collected, you could get the most perfect score on your fairness test, but it still would be heavily discriminating.
To give you an example, there's this famous ProPublica case where algorithms were used to help judges come to a decision who should get early released from probation versus who has to stay in, and their scientists found that there was discrimination happening against black skinned individuals. And if you looked at the data that was used to train the system, The algorithm was behaving correctly. It satisfied a specific mathematical fairness definition, and it also didn't do anything unfair if you looked at the data source. The problem came before the data was initially collected because it happened to be that police was predominantly present in predominantly black neighborhoods, which of course led to the result reflected in your data, that more black skinned individuals.
commit a crime after first being held in probation. And therefore, it was a correct conclusion that it would make sense to keep them in. Of course, if you consider that if more police would have been present in predominantly white neighborhoods, they might have seen the same rate of crime happening, then this would change the picture.
So it's never, ever enough to just measure fairness and just rely on a mathematical way to do so. But you really have to think hard, how was the data collected? How am I using this AI system? Which parameters am I using in my model? How am I modeling things? Because all of these things can actually affect the outcome.
So as we've discussed, quite a tricky problem.
Richie Cotton: Absolutely. And data collection is one of those things where it's sort of in principle seems easy. It's like, oh, yeah, you just go out and collection data. And then often by the time it reaches a data analyst or a data scientist, they kind of assume, well, okay, the data is probably fine. This is what I've got to work with.
And then the rest of the analysis is fine. But you've actually got a problem right at the start. So I guess related to this, so we talked a bit about how sometimes AI can give you the wrong answer. And are there any ways to sort of, mitigate problems with this, like how do you communicate this to your customers say, okay, well, you know, sometimes you get the wrong answer and how do you deal with it?
Alexandra Ebert: Can you give me a specific example where you're interested in this answer? Because I think if we keep it on this high level, then I again can only give you a high level answer.
Richie Cotton: Okay. Medical diagnostics is a good example. So quite often use AI to assist doctors in coming up with a result. So, given the AI can sometimes give the wrong answer, how might the doctor go about communicating this? What might you do to. Keep trust in the AI system, but still have your patient happy.
Alexandra Ebert: Yeah, makes sense. So I'm neither a medical expert nor a psychologist to know what's the best way to deliver messages, even though I've heard that particularly in a marketing context, people actually appreciate getting bad AI and positive news from the human being. Not sure if this can be translated to a medical context, though.
I would say if it's still okay to use AI in this context, which for example, would be that even though there might be some wrong answers overall, you would get correct answers or you would perform better than if you only had the doctor without AI assistance making decisions about, I don't know whether you have cancer or something like that.
the case. And if overall it makes sense to use AI here because you can more positively impact more patients, then I would still communicate that the AI system not always is 100 percent wrong. And I hope that the specific hospital also doesn't let the AI. on its own, do the doctor's job, but that it's just used as an assistance tool.
And therefore this could go about and explain how AI is one piece of the puzzle that helps the doctor to come to its conclusion. So I could imagine that something like that would be appreciated.
Richie Cotton: Okay, so it is possible to still retrain some trust, even if you are getting wrong answers some of the time, just as long as you're a bit clear on exactly what's going on and explain how AI is not like everything, there is also a human involved.
Alexandra Ebert: Exactly. So I think that's one point. And also we oftentimes have this conversation with self driving cars where this question is, okay, what if the self driving car has to make the decision between running over the granny or the group of school kids, which kind of. a decision to take, that's, of course, a hard problem.
But I think in this context, even more interesting question is, how does it look on a more overall level? So we have, I don't know the exact number, but we have a significant percentage of people dying in traffic each year. If we can reduce this number to, let's say, only 10 percent of this number with switching to self driving cars, is it for us on a societal level okay to use AI and to use self driving cars, or do we still insist that everything has to be human, including the human damages that we see in traffic?
And again, this brings us to this point that AI is touching us in so many different levels of our day to day life, that it can't be data scientists alone making these decisions. It's important that also on the regulatory side, they will come up. with how to navigate this current challenges, because as of now, we still have scenarios where anti discrimination laws and privacy laws sometimes force data scientists to build AI that's, for example, discriminatory, but legally compliant, or to build something that's not legally compliant and in conflict with privacy and anti discrimination laws, but does a better job on the fairness side.
And I think this is something that we also need to clean up on a societal level, on a regulatory level.
Richie Cotton: Okay, yeah, so, Maybe in some sense, it's about benchmarking and saying, well, you're not going to get a perfect AI system, but how well does it do relative to humans? And so certainly with the self driving car example, if if the car can drive better than a human crashes less often, then probably that's good enough compared to it crashing.
Absolutely. Never.
Alexandra Ebert: For some societies, others might have a different opinion. So of course, I'm not the, not the judge on this, but I think that's one way to think about it. Yes,
Richie Cotton: Okay. Alright. I'd like to move on and talk a bit about privacy with ai. can you tell me like what are the most common privacy concerns related to AI
Alexandra Ebert: I'm going to answer this on the business perspective. So for businesses, when they want to use AI, the most common privacy concerns first are not having access to data because it's not possible to access them in compliance with GDPR, CCPA, other privacy laws, or It's the time to data because they have to go through this cumbersome case by case processes of anonymizing data.
And then it just takes what we hear from the organizations we had mostly I work with, if they're lucky a few weeks, but much more often two months, five months, six months, sometimes even eight months if they want to share externally. So these are the two big. privacy problems, and then, of course, in case they use some data unlawfully, if this comes out, then, of course, not only the legal repercussions, but also the reputational damage that comes with that.
Richie Cotton: and with generative AI in particular? I think there are sort of some new worries around the use of data particularly like, people's privacy being violated or in fact, like the sensitive business data being sent to the model and then suddenly it being generated in someone else's conversation.
Can you tell me how real of a concern this is?
Alexandra Ebert: I would say the biggest privacy concern with generative AI and particularly large language models like GPT and ChatGPT, the biggest privacy concern many legal professionals and privacy pros and organizations have right now is their employees actually using ChatGPT and mindlessly typing in some privacy sensitive data of their customers because that's definitely a privacy breach.
On the other side of the spectrum, if I, as an individual, am concerned about generative AI infringing my privacy, I would say it's a problem, but in the current discussion, it's much more heavily debated whether it's okay that LLMs like GPT scrape the entire internet, including all the copyright infringements that come with that.
Of course, if you have your personal data also publicly available. It quite probably was included in some training data for generative AI. How big of a concern that is on a general level, of course, hard to tell, but that definitely has been some scary examples. So for example, a US professor in who was accused by Chattopadhyay to sexually harass people, which wasn't true, but since some information was picked from the left, some information from the right, and also his real name, then this resulted in some accusations that nobody wants to have out there about themselves.
So that's definitely a problem. And then also in the context of hallucinations, if I get a name from ChatGPT that happens to exist in real life. And then something bad that this person did, for example, it's hard for me to tell whether this actually happened, particularly with ChatGPT being so deceptive, giving you I don't know, fake URLs to New York Times articles, even sources to papers and something like that.
And this brings us back to this point, why it's so important to educate every user. That GPT has some of these problems and that generative AI large language models tend to hallucinate some information and that you have to take it with a grain of salt.
Richie Cotton: Absolutely. So, if you're looking for facts then probably the generative AI,
Alexandra Ebert: It's not
Richie Cotton: you've got to be very careful in terms of using it. It's maybe
Alexandra Ebert: at least this type of generative AI, there are others, but with large language models like that, in this context, you shouldn't use them as your crystal ball.
Richie Cotton: Okay. And can you just talk me through, like, what are the most sensitive types of data when it comes to AI? what do you need to be worried about with respect to privacy?
Alexandra Ebert: I think a common misunderstanding when we talk about sensitive personal data is that many people have this old concept of personal data in their mind, which is okay. First name, last name, home address, social security number, something like that. But in today's world, where we have big data, where we have behavioral data assets, scientists actually concluded that there's no, not personal data anymore.
And it's really surprising how easy it is to re identify customers, employees, based on just tiny bits and pieces of data. And therefore, it's important to protect your entire data asset. So to give you a more tangible example, one study, for example, looked into credit card data.
And everybody, I would say, has at least a few dozen, if not a few hundreds of credit card transactions per year. And this study found that only three out of these hundreds of transactions per individual are sufficient to re identify over 80 percent of individuals. But the surprising thing is you don't even need the entire information about this transaction.
You just need the date and the merchant. So something like yesterday, Starbucks, the week before Walmart, and two weeks ago, McDonald's. These tiny bits and pieces are sufficient to re identify over. 80 percent of customers, and I think this is something that many people are not even aware of and why they still rely on legacy anonymization technologies like masking, obfuscation, and the like, which by now are proven to not work for this big behavioral data assets anymore.
Richie Cotton: That is fascinating and also terrifying. I mean, the fact that you said that you can identify people just from like a Walmart or a, McDonald's purchase, it's not even like, Oh, they bought some like, obscure thing. It's
Alexandra Ebert: Whatever, no it's, it's really very basic information and I could give you the same examples for healthcare, for telecommunication, mobility data, even demographic data, because today we have this much more unique digital fingerprints and much more high dimensional data, which is something that legacy anonymization techniques, which always stick to the original data and just try to delete, distort, mask certain elements of this data simply can't protect anymore.
Richie Cotton: so in that case, you're saying like a lot of the standard techniques don't work. What can we do to mitigate these privacy concerns?
Alexandra Ebert: Great question again, and this is why I'm so passionate about synthetic data, which is also something that's was created with generative AI, but in a completely different context than what many people now are aware of with chat GPT and the like. So AI generated synthetic data is basically anonymization technology that was developed to solve particularly this problem.
How to. Protect people's privacy, how to create something that's impossible to reverse engineer, that's impossible to reidentify, while at the same time not destroying the utility of a data set, because you as a data savvy person, but I'm just highlighting it also for everybody else listening today, can already guess that if you have this rich amount financial transactions and everything you as a data scientist get is Walmart, McDonald's, Starbucks, and three dates, of course, the analytical value, the utility of this data set is heavily diminished.
And the interesting thing with synthetic data is I can basically have the cake and eat it too. So what happens here is that. We work a lot with financial services providers, insurance organizations, that they use a software like ours, a synthetic data generator to let generative AI train on their existing customer data sets, the existing financial transaction insurance claims.
And the generative AI can automatically understand the patterns, the correlation, the structure of the data set. So basically how an organization's customers. Act and behave, and then in a completely separate step, once this training, this learning process is completed, you can use the synthetic data generated to create new synthetic customers and their synthetic financial transactions, their synthetic insurance claims, or their health care records.
And from a statistical point of view, the real production like data and the synthetic data will be nearly indistinguishable. Just a ballpark estimate or a very rough number to paint a picture. You can basically retain 99 percent of information. You lose the extreme outliers. So, for example, we in Austria don't have as many billionaires as we have in the United States.
If one of our Austrian customer banks synthesizes their customer base and they only have two billionaires, they might not be included, but they will still get a much more granular picture of fully anonymous synthetic data. And that's so interesting, not only because it finally allows you to Accurate and representative data for machine learning purposes to share with startups to upload to the cloud and use for machine learning projects, but you can also be much more inclusive and much more diverse in what you develop because you don't only get this average.
Chain or John Doe that you get with legacy anonymization, where you have to strip so much away, aggregate a lot and so on. But you really get granular level individuals down to the very edges of your spectrum of customers. And this also allows you to understand how do, does this subpopulation of our customers behave, how do they act and behave and how can I develop products and services that cater much better to their needs and not only to the average customer.
Richie Cotton: Okay, so this sounds really useful in that you've got some, well, I want to pretend data is not the right word, but this, so the synthetic data is giving you some of the same properties of your original data set, but it does have this sort of privacy benefit. I'm worried that if you start making up data, is it gonna cause those sort of accuracy and fairness problems that we discussed before?
Cool.
Alexandra Ebert: No, and partially. So synthetic data or the partially was was related to the fairness. Let's start with the accuracy. I mentioned you can't get 100%. It simply wouldn't be possible from a privacy point of view, but you get super, super close to your production date. And this is also where our customers find when the first test synthetic data.
And they do this mainly by training a machine learning model on the production data and then a machine learning model on the synthetic data that was created from this production data. And they find that the performance is on par or that they have a deviation from area under the curve of, I don't know, 0.
2 or something like that. So it's really a super interesting technology that is as good as their production data. particularly for those organizations who are in a position of saying, okay, either we don't do anything because we can't get access to data or we use synthetic data, which is so close to the real that we can use it as a replacement.
So from an accuracy point of view, there are not really any concerns with synthetic data, because particularly from machine learning and not interested in this one billionaire, but I want to find granular yet. generalizable patterns, and this is what still sticks or still stays in the synthetic data.
Fairness question, however, with the general process of creating synthetic data, you want to have everything from the statistics, everything from the information in there as in the original. So if your data set originally was biased or discriminating or something like that, you will still find this in the synthetic data set.
But that's oftentimes a good thing because in most organizations. really just a tiny group of privileged people can even access the production grade data. So it's really hard for them, particularly if you don't have the knowledge, to spot any potential bias problems. But once the data is synthetic, you can share it with a much broader group of people, which on the one hand brings in diversity, but also allows you to even share it externally, for example, with experts on AI fairness.
Because many organizations don't yet have this experience in house and get their point of view on whether this data is suitable, whether you need to collect additional ones, or how you could mitigate this. So in this sense, it can actually help with fairness, but you will still have the biases in there that you had originally in there.
So you still need to mitigate them once you have detected them. And then there's also this fun thing called fear synthetic data, which helps you to mitigate biases in the data set. Think of a data set where you don't have a gender pay gap anymore, or we have much more ethnic diversity in there. This works to a certain extent and in some cases can help with fairness, but it's definitely not the solution to end all fairness problems that we have with fairness.
Richie Cotton: It does sound like we're going back to this idea that there are lots of different metrics for fairness and you need to do a bit of controlling and thinking about exactly how you want to get to those targets or how you're going to measure what success looks like.
Alexandra Ebert: Exactly, Exactly. So if I want to have a better understanding of how many females I have in different management positions in my company, having a fair synthetic data set where everything is 50 50 or something like that, of course, would give me a wrong picture. So for analytical purposes, it might doesn't make sense to change the real world, but in some areas.
You don't want AI to perpetuate historical biases. And for example, building a model where you want AI to suggest which salary you should offer a new candidate. There, it could make sense to also tweak the data and make it fairer to improve fairness in downstream models, but it's something that's rather new.
And we, for example, have one customer who started experimenting with this, Humana, I also have a data democratization podcast out on this, which we can maybe link in the show notes, where they're looking into fair synthetic data to help them to. be much more inclusive in their quest to be more proactive with health care and have more ethnic diversity in a data set to make sure that algorithms then allocate health resources more fairly and diversity.
Richie Cotton: This is fascinating stuff and it sounds like, because you're using generative AI to create the synthetic data, it's like, it feels like it's a productive use of hallucinating in some sense.
Alexandra Ebert: I wouldn't say that it's hallucinating in the same sense as we see with chat GPT because there we sometimes don't even have a clue where this information is coming from. With synthetic data, you're super close to the original. With fair synthetic data, you're still informed by the patterns in your original data set.
So you couldn't use this process if there wasn't any example to learn from. Let's think of a medical study that was only performed on a male body. There, it wouldn't make sense to let the AI dream up and hallucinate stuff. But if you have just a few high earning females, for example, but the AI could learn on other high earning individuals, then it's much better in giving you realistic examples that could have happened, but just.
didn't happen to be in your data set. So it's much closer to the room of real possibilities and not as all over the place with some other hallucinations that we see.
Richie Cotton: So, Let's nerd out for a moment. So this idea of creating synthetic data does sound very close to the idea of imputation, where you sort of fill in the blanks in missing data. Are these two techniques related at all?
Alexandra Ebert: You can actually use synthetic data to impute data much more effectively than with more common approaches, but no, I wouldn't say that they're similar because I mentioned earlier that legacy anonymization techniques always stick to the original data and just try to strike through. the stored mask, certain elements of the data with synthetic data.
On the other hand, you learn the entire data set. So with legacy anonymization, let's say if I had 200 columns in the beginning, I would only have three columns, five columns in the end because I had to delete so much information. With synthetic data, all the information is there and I learn all the information, its entirety to then present you with another data set where I again have.
All the 200 columns populated. So it's not necessarily filling out missing gaps. You could, of course, use it if there are some gaps, some values that you need to impute to also fill them out with a much better educated guest and more simple imputation approaches. But in general, you need to have quality data to get quality synthetic data.
And it's not so much the purpose of imputing information, but rather protecting privacy while retaining the value.
Richie Cotton: before we move on from synthetic data, one last question on this topic. it seems like the main benefit is around the privacy of data, and you get some sort of benefits around, you can control for different types of fairness. Are there any other benefits of using synthetic data?
Alexandra Ebert: Actually plenty. I mean, you're right. Privacy is oftentimes one of the reasons why synthetic data is discovered, but it's also this time to data element that I mentioned, which is a huge concern for many organizations today because particularly smaller organizations are quite fast in innovating and the larger organizations, particularly in banking, financial services are afraid of lagging behind.
So getting access to data in a matter of one day or one week in contrast So having to wait six months as a data scientist is definitely quite interesting. So it's the time to data. It's the collaboration aspect. Many of our customers use synthetic data to kind of kickstart collaborations with startups or with other partners in the AI ecosystem, because it's so challenging to get data out there.
It's also more innovation because in many organizations, we still see the data is siloed and only a small group of people can access this resource. But also you would. data camp with your mission to democratize data skills, see that if more people are empowered with data, and this could also be synthetic data in an organization, then this can have massive impact on the capacity of an organization to innovate and do something that they haven't yet considered before.
So this is also why more and more organizations use synthetic data, not on a case by case basis to, for example, say we have a credit scoring algorithm that's not performing as good as it could be with synthetic data. We can feed in high quality data and we can improve this, but that really provide internal synthetic data hubs or marketplaces to any employee from the intern to the CEO so that much more people can become data driven and can really understand.
And are the customers of our organizations and how could it translate this to innovation. So these are a few of the points how synthetic data is used today. And then an emerging area is the whole part of synthetic data for augmentation purposes. Rebalancing data, fair synthetic data, using it to impute and in the future also to simulate certain things.
So it's really quite a growing area, but already today quite interesting not only for privacy.
Richie Cotton: That's absolutely fascinating. The idea that by getting rid of some of the privacy concerns, you've actually improved productivity because more people get access to it and you're gonna be able to get results just much faster. So you really are improving performance there. Brilliant. so like to talk a bit about transparency and explainability of AI.
I think sometimes AI is seen as a black box, but it's not sort of clear like how AI made the decision. do you have any ideas on how you can make AI more explainable or more transparent to users?
Alexandra Ebert: I think there are plenty of different tools out there to improve explainability of AI. But what I see very often with data scientists approaching the problem is how can they build in explainability that helps them to debug a model. But I think explainability also in the context of regulators is really seen as something that should not only serve the creators of AI.
But also those affected by it, maybe auditors and so on. So I think one very good point to start is what is actually the information that a user would need to understand to be able to, for example, challenge a decision made by your AI and then work out from that. And as many people find, it doesn't have to be that.
detailed, it doesn't have to be that granular, which is why this excuse of, Hey, this AI is a black box. We don't know how it is actually operating oftentimes can't be holed up anymore because what users actually expect is much more high level, but it's an open area of research. So again, not the three points how to actually achieve explainability.
Richie Cotton: Definitely, it does seem to be an ongoing problem is trying to figure this out. But it does sound like if you can show people, like, what data is going in, that's at least gonna make it a little bit more transparent about what's happening. And perhaps this is where synthetic data is gonna help you out.
Alexandra Ebert: It's on the one hand, what data is going in and for what AI is used in the first place, but also which data points or the feature importance the most influential on a given outcome and so on. I think this also brings us a little bit to distinction between transparency and explainability, which if you ask different experts, you will get plenty of different answers because again, this is something researchers haven't concluded about how to actually define it, but transparency at least the understanding that I like to perpetuate is more on this general level.
How is the system working? For many, initially, it's just being transparent that AI is used, that I'm affected by AI at the moment. But of course, you shouldn't stop there. You should give a general explanation of what's the purpose of the system. which data is used, which data is processed, what's the feature importance how are decisions being made, which training data was used, how did I mitigate biases, so it's also some overlap to fairness versus the explainability being seen more as a reasoning for the individual decision that the individual output that a model created, so that I as an affected person can understand how was this decision about me being denied credit was made versus the overall system explanations, which wouldn't help me to argue and make my case in this context.
Since you mentioned synthetic data, yes, synthetic data can help with explainability, particularly because we as human beings can't reason upon the model just based on the code of it. We need to have specific examples, and particularly if I as an external auditor, for example, or even within an organization as a different unit that that's job it is to make sure and assess that the systems that are put out are trustworthy, explainable, I would need plenty of different granular examples to see how does my model behave in different scenarios, how does it behave with particular sensitive outliers, and here synthetic data is so handy because there's no privacy concern, so there's no issue to share it with another department and not even an issue to share it with an external auditor, and yet it becomes possible to argue on an individual level how specific decisions are being made.
Richie Cotton: In this case you could make up your billionaire and they've got their three purchases of a yacht and a Lamborghini and whatever else. And then that's okay. Cause you've not revealed the privacy of like that of any real individual.
Alexandra Ebert: exactly. So oftentimes this is also one finding when it comes to AI fairness. Microsoft Research conducted a survey, I think back in 2021, with leading AI fairness practitioners. Number one problem they had in counteracting AI bias was actually knowing whether the model was biased or not. Because again, anti discrimination laws, privacy laws, oftentimes prohibit that you use sensitive attributes.
So they have to operate in the blind and don't see if there's some adverse effects happening for specific ethnicity groups or so. With synthetic data, you can not only make these sensitive attributes available from your existing customer base, but you could also use it, and there we come back to this fear synthetic data idea, to create more diverse could have happened in the real world.
And you with your human expertise can assess this, but that happened to not be in your customer base, not in your training data set, and therefore something the algorithm wasn't exposed to. And I think this becomes quite interesting, particularly with this whole AI assurance ecosystems that are currently created, because there's so many organizations that should provide this auditory and certification services, and many of them are currently looking into synthetic data to bridge this privacy gap between.
getting access to a customer's data and making their assessment and their decision.
Richie Cotton: And so once you start getting into this regulatory aspect, it really is quite important that you both keeping data private, but also like showing the regulator some stuff and explaining things to them to see what's going on. Okay. So like to talk a little bit about the processes involved in ensuring trust in AI.
So, Okay. Are there any particular processes that you think are important in making sure that any AI you're building is going to be trustable?
Alexandra Ebert: I think the process aside is tremendously important. Many organizations just think about their AI principles, set out with the list of, okay, we want to have fair AI, explainable AI, transparent, robust, privacy friendly AI, and then forget about it. So to make sure that this is something that's actually translated into practice, you need to incorporate this in your existing business processes.
You need to set up. the governance structures, you need to educate people on how to actually translate these high level principles into the day to day data science and engineering work. It's also something that involves a broad group of people. You will, as we already discussed before, run into problems where not individuals, not data scientists can decide, okay, how to move forward, but where it would be helpful to have a group of AI experts.
responsible AI experts, legal experts, and so on and so forth to come to this decision. And then one other element, which many organizations tend to forget, it's not only about building your own AI, but you definitely also need to have some guidelines and processes for the procurement of AI systems, because this is an area that's oftentimes left out in programs.
But as we all know, there's so many AI systems being developed and many more systems will have AI components in the future. And therefore it's important to also think about procurement and which questions to ask new vendors, et cetera.
Richie Cotton: So it does sound like there's going to be a lot of teams involved in this. Can you maybe sort of, iterate, like, what are the different roles involved in creating AI or making sure that AI is trustable and just how they interact with each other?
Alexandra Ebert: So if you want to set your organization up for success, as with many other initiatives, C level support is something that's tremendously helpful. I also mentioned, of course that you want to have a more holistic view and many different perspectives, not only from different professional departments, but also from a diversity point of view, young, old, different ethnicities, different cultures.
This is definitely something AI centers of excellence, for example, or even some committees that have to decide about more challenging or high. Stakes scenarios. Of course it wouldn't work without the CDO and many technical folks working in this department. It wouldn't work without the legal specialists, and I think it's in general something that should touch every employee in an organization, but just with a different depth of information.
But we all know that so many people will use AI based systems. And even if I'm just using chat GPT and not building GPT like systems, I would benefit from some responsible AI information to know how I can use this in a trustworthy manner that doesn't hurt my organization or my customer base.
Richie Cotton: So since you mentioned that this is going to touch basically everyone in the organization, what sort of skills do you think everyone needs related to trust in AI? Like, what should everyone know?
Alexandra Ebert: So I think AI and data literacy skills at large definitely help, but more specifically diving into all these elements of responsible or ethical AI, so having a general understanding of what the challenges with fairness are, why this is not such a black and white issue, but really tricky, explainability, privacy.
Privacy and so on. And I think most importantly, this general understanding of that many of these aspects can't be tackled at one stage of the AI development or deployment life cycle, but really have to be considered throughout the process. So I think that's the kind of entry level information, but in general, I would wish for people to look more into AI ethics, even though it might, doesn't sound that interesting at the beginning.
Richie Cotton: I feel like AI, I think it's this sort of thing, it's good to have like a late night argument with people about. So it's probably, yeah, it's worth having a bit of a learn about it, if only for that. Okay. and on the more technical level, like once you got past the sort of the basics particularly for people who are in data roles or machine learning roles.
What sort of skills do you need there to make good use of trustable AI?
Alexandra Ebert: I would say more in depth ethics if you really want to build it yourself, but also again, bringing this back on the meta level, I think we need much more responsible AI talent, but with the speed of the development that is ongoing and also with this ambition of many nations, economic unions to have significantly more organizations using AI, we need to have AI governance and responsible AI assurance ecosystems because otherwise this is not going to work out and I think in this puzzle also the general purpose AI systems of the big tech companies will have an important role to play because I would assume that in the coming years.
I don't know, 80, 90 percent of all AI systems will have some of these building blocks from the big tech companies in there. And they are currently some of the best equipped institutions to not only make sure that they're building blocks are adhering to responsible AI principles, but also developing new tools that making assessing and achieving AI fairness, explainability, a breeze for the end users of the systems and or the developers of the systems.
So I think that's one area where I really hope. that we will see much more coming from.
Richie Cotton: Okay, yeah, that would be nice if there were tools to sort of help you out with maybe some of these ethical issues and and fairness issues and things like
Alexandra Ebert: Exactly. And we have many of these tools today, but it's still very early stage and many still think, okay, if I use this specific tool, then I could put the check mark under the fairness questions. It's much more complicated than that. And really having some more comprehensive, holistic tools that help you to develop it in a more, string and manner, I think this is something that's still needed, but we still have a vast open area of research, so we don't yet have all the answers to that, but I hope that more of these will come in the coming years.
Richie Cotton: Okay, yeah, it does sound like maybe the mathematical side of this is the easy part and the tricky bit is really thinking about, like, well, what, how does fairness apply in this particular context?
Alexandra Ebert: Absolutely.
Richie Cotton: Okay. All right. So, before we wrap up is there anything that you're particularly excited about mostly?
Alexandra Ebert: Anything I'm particularly excited about. I mean, in general, I love the world of synthetic data, not only because it helps you to reconcile data utility and using data, democratizing data with the privacy side of things, but also for this it. impact on responsible AI, and what I'm personally excited about in the next few months is definitely the ongoing work with different regulators being allowed to advise in some responsible AI context, and also all the keynote speaking and podcasting that I get to do because it always exposes me so to so many brilliant minds, and this is something that I really appreciate.
Richie Cotton: Fantastic. And do you have any final advice for people who want to improve their skills or learn more about trust in AI?
Alexandra Ebert: Go to Datacamp,
Richie Cotton: That's the best answer. I like that.
Alexandra Ebert: not sponsored. No, definitely great way how you approve, how you approach data literacy. And of course, educate yourself on AI ethics in particular. And hopefully there will be plenty of courses on this topic on Datacamp, including synthetic data in the future.
Richie Cotton: Absolutely. All right. On that note, we'll wrap up. Thank you very much for your time, Alexandra.
Alexandra Ebert: Thank you for having me, Richard. It was a blast.
You’re invited! Join us for Radar: AI Edition
DataCamp Team
2 min
The Art of Prompt Engineering with Alex Banks, Founder and Educator, Sunday Signal
The Future of Programming with Kyle Daigle, COO at GitHub
A Comprehensive Guide to Working with the Mistral Large Model
Josep Ferrer
12 min
Serving an LLM Application as an API Endpoint using FastAPI in Python
How to Improve RAG Performance: 5 Key Techniques with Examples
Eugenia Anello