How Data Science is Transforming the NBA
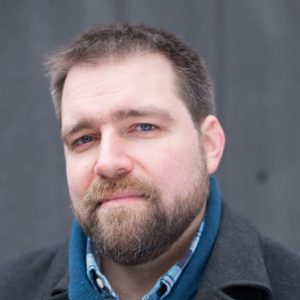
Seth Partnow is the Director of North American Sports at StatsBomb, where he previously served as their Director of Basketball Analytics. Prior to joining StatsBomb in 2021, Seth was the Director of Basketball Research for the Milwaukee Bucks basketball team. Seth is also an accomplished Analyst and Author, having worked as an NBA Analyst for The Athletic since 2019 and having published his own book on basketball analytics, The Midrange Theory.
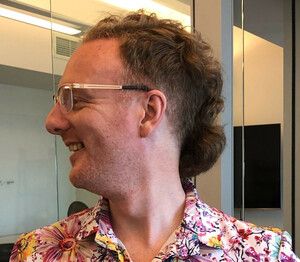
Richie helps individuals and organizations get better at using data and AI. He's been a data scientist since before it was called data science, and has written two books and created many DataCamp courses on the subject. He is a host of the DataFramed podcast, and runs DataCamp's webinar program.
Key Quotes
A key inflection point in the rise of the 3-pointer was the implementation of the play-by-play data. There's a bit of conventional wisdom that's like, all right, you're not a great three-point shooter. Take a dribble and get an easier shot. Now it stands to reason that taking a shot 19 feet away from the basket is going to go in more than taking a shot from 24 feet away from the basket. Two point shots have always been made at a much higher percentage than three point shots, even more often and at a higher rate more than the 24 footer that even the extra point does not overcome that increase in efficiency, that increase in accuracy. Empirically, what we found is that's not true. Once we had detailed location on where shots were taken from, you kind of see that shots near the basket go in a whole lot, it declines very quickly until you get out to about five feet. And then once you get out to about nine feet from the basket, it basically stays more like pretty level declining slowly until you get out about 27 feet away from the basket. So, you might make the 19 footer two percentage point higher rate than the 24 footer, but the extra point swamps that advantage. With the data, it's demonstrable that there are very few players for whom the shot from that one step further in is actually worth doing than the shot from further out. But until we had that data. It was an argument that was plausible either way.
I think there is an important broadly applicable lesson about the importance of having knowledge of your subject matter, so you're aware of what the data you're working with actually says, actually represents, it's an abstraction of the reality. What's what is it abstracting away? What is it capturing? What isn't it capturing? I think that and having a decent level of understanding of that really gives you the first level of sniff test of almost QA-ing your own work. You get a result, ‘that doesn't make sense.’ ‘I did something wrong.’ ‘My data is bad.’ ‘Let me go examine that again before I, look what I found.’ And then it was you reversed a sign somewhere in your code or the data was faulty in some ways. You're in a garbage-in garbage-out scenario, then, being able to catch that yourself can save you a lot of pain. I guess if I was going to reduce what I just said to two, two maximums, it would be like, pick your lane and learn about the thing you're doing data science work on, learn about the thing you want to talk about. So we can talk about it intelligently.
Key Takeaways
Know how your data is generated. Regardless of whether it’s sports or a product, understand the processes that lead to the generation of the data you’ll work with. Understanding the subject you're analyzing is crucial as it allows you to interpret the data accurately, use appropriate terminology, and avoid misinterpretations that could lead to faulty conclusions.
When building models, prioritizing interpretability can be more beneficial than seeking the highest predictive power. An explainable model is easier to sell to decision-makers and can have a bigger impact.
There is power in saying “I don’t know”—In the face of unpredictable outcomes, learning to say "I don't know" can be powerful. This acceptance of uncertainty can lead to more careful data analysis and prevent hasty, potentially incorrect conclusions.
Transcript
Richie Cotton:
Hi Seth, great to have you on the show.
Seth Partnow:
Thanks for having me.
Richie Cotton:
Brilliant. Yeah, I'd love to just kick off with what basketball teams care about. So can you stop by telling me what sort of problems the team's tried to solve with data?
Seth Partnow:
So there are on the first of all, the when we're talking about sports organizations, the first sort of split is between the sports side and the business side. And for most top, like, certainly at the top level of sports, not just in the US, but across the world, like the people who are doing the analysis, the recruitment, those things. are very separate from the people who are worrying about, you know, ticket prices and, and, and, you know, projecting concessions and, and, um, and all those, those, those fun data challenges that, that sort of revolve around revenue generation and drawing eyeballs and stuff like that. So that's, that's the first one. And I am a wholly on the side of the, of the kind of the sport side. I, uh, I, you know, it was with the bucks. I, there were people who did similar jobs in the business side, but kind of never the twain shall meet. I mean, they were friends of mine, but we didn't work together other than, you know, some pooled technical resources. But broadly speaking, it's a very different jobs. Now, within the sport itself, there's kind of three areas really where data can have an impact. The first one is sort of sports science... See more
Richie Cotton:
All right, so it's kind of amazing if you think, okay, well, it's just about winning games, but actually there's all sorts of data involved in these kind of supporting functions of like, well, how do you even optimize, how many tickets you sell, or how many cans of beer you sell, ringside or whatever. So, all right, today we'll focus on the sports side of things. So can you tell me, are there any teams that you think make particularly good use of data?
Seth Partnow:
That's always a little tough to say from the outside. It's sort of more anecdotal from talking to people inside organizations. It's always hard to say, well, that was a data decision they made. It's much more about how integrated in the process is the use of data. There's sort of two poles, and most teams fall somewhere in between. Uh, but they tend to one side or another on one hand, it's sort of using data as like a, almost a, a encyclopedia resource where, all right, we've done all the, we've done all the real work. Let's consult the data to see what they have to say about it. And on the other hand, it's, it's something that is sort of integrated at every stage of the information gathering, dissemination analysis, decision process. Um, and I think. early in the days of sports analytics, many more teams were, were sort of on the, uh, well, let's go ask the nerd, uh, after we've largely made the decision side. And it's sort of over time is pushing towards the other way, but there are still definitely teams that are, um, you know, this isn't just basketball. This is every sport. Um, now that data analytics has become a thing that gets noted. Um, and you know, there are, uh, ESPN does rankings every year across sports of which teams are, are kind of the most data forward and it's not great PR to be last in those. So there are teams that have those groups to just to say they have them, not necessarily making great use of them or really getting anything out of it because it's this siloed off thing. And, and there are other teams again, that, that have it fully kind of, you know, internalized in. the process of everything they do. So it's almost hard to separate it out from kind of the broader decision-making process.
Richie Cotton:
I think maybe a lot of our listeners will experience the idea of being a data person who's just sort of token, let's throw in some data to support the decision we've made already. But it does sound like some progress has been made in terms of integrating data and analytics within to the game. And I would add one thing that sort of, these are almost two axes in the level of investment from a headcount financial standpoint and the level of integration. There's some correlation because the teams that tend, there's some tendency for the teams that are well invested to want to integrate that. But it's not at all a linear correlation. There are some teams that have. You know, very small levels of investment and, and, you know, only, uh, only one or two people, but they involve them intimately and there are teams that are spending a whole bunch of money and not getting much out of it because it's, it's sort of, well, we've got this fancy group and we asked them questions and then we go do what we're going to do anyway.
Richie Cotton:
Okay, do you have a sense of whether the teams mostly do their analytics in-house or are they using consultancies or data platforms, things like that?
Seth Partnow:
For the most part at the NBA level, it's mostly in house. Now there are some use of some use of consultants for certain problems of certain degree of complexity. I think a lot of a fair amount of kind of systems development work is maybe outsourced. This is a difference between baseball and I think other American sports is baseball is so integrated with use of data that there's almost two tech two data groups within a baseball organization. There's one that's the analysis and the other one that's sort of systems and platform and stuff like that. But that's that's, you know, having a 15 year head start and certainly with respect to basketball, a much larger player pool to deal with. You know, a baseball team will have the major league team and then their minor league subsidiaries and looking at, you know, drafting players at volume from all over the world. Whereas the NBA team has 15 guys and maybe a G league team, which has maybe four or five guys they care about. And the universe of, of players who conceivably matter is considerably smaller. So the, the, just the, the, the head count is, is just naturally smaller to deal with that, you know, more contained. bits of analysis you're doing.
Richie Cotton:
That's really interesting that it seems like recruiting players then is a bit more of a... I don't know whether... is it an easier problem or is it just like you've got a smaller pool? I'm not sure whether it makes it easier or harder.
Seth Partnow:
I don't know if it's easier or harder, but it is certainly an easier way for it. It's the acceptance of using data in that effort is an easier road because, you know, the evaluation of players has always includes some level of statistical, you know, now, well, we have better stats now, so let's use the better stats and more advanced methods to get those better stats. All right, we're still doing basically the same thing we were doing before. We're just using a little bit of technology, a little bit of statistical technique to be a little sharper at it. That's a difference in degree, but not in kind. Whereas certainly with the advent of player tracking data, the application of that to kind of on floor in game strategy, that's a little bit newer, a little bit different. probably a little bit more directly threatening to sort of the existing kind of coaching structure. So while that's certainly has gained inroads there, that was kind of a tougher nut to crack initially than the personnel side.
Richie Cotton:
Okay, interesting. All right, I'd like to talk a little bit about your book. So do you wanna tell us about what the midrange theory is about?
Seth Partnow:
Sure. So the mid-range theory is in some ways, uh, uh, I I'll use the, the analogy of a band in that the first studio album of band records, they have, they have the entirety of the material they've ever developed over the course of their lives and they can pick the best stuff and throw it in there. Um, and on some level that's, that's a little bit what the mid-range theory, uh, isn't and was, is a lot of topics that I have, I've written about research thought about, talked about, pretty extensively over the time that I've been, you know, doing basketball analysis and kind of sharpened it up and put it, you know, kind of in sort of anthological form, put it together and put it out in the world.
Richie Cotton:
Fantastic. I have to say, it is a great read, like really, really amazing sort of details and folklore about basketball. But one of the things that was a recurring theme was that it's really hard to figure out the attribution of like how, which player contributed what to a game. Can you tell me why there's a problem in basketball?
Seth Partnow:
Um, I think it is, it is, uh, of this team sports where credit attribution is fairly straightforward. They're kind of one-on-one confrontation games. So baseball, cricket, sports like that. Uh, a bowl or a pitcher throws the ball of a batter or a batsman hits ball. Things happen. It's not too terribly difficult. Obviously how the fielders interact and stuff like that is a little more complicated, but largely speaking. Either the pitcher is able to, the pitcher or the bowler is able to fool the batter or not. And either the batter is able to execute, you know, hitting the ball well or not. That's pretty straightforward in terms of the one person's success is to some degree, is the other person's failure. In a sport like basketball or soccer or American football or hockey. or any other rugby or Australian rules football or any of these other more dynamic sports, um, the interaction between players, uh, often has a, a great deal of impact on what the outcome is. I can certainly look and see, okay. I know what percentage of shots a player made. We certainly have the data now to, to know that the quality, the expectation of those shots going into difficulty of those shots can vary widely from player to player and figuring out why a player is getting easier or harder shots is quite difficult. Maybe a player is very good at freeing themselves. Maybe they have teammates who draw a bunch of attention or very good passers or any number of other things. So how do you divide the credit for, okay, this player made, made a lot of shots. They, they got very open shots. Is that them doing something? Well, is that teammate doing something? Well, how would that change in another context where they were being asked to do more or less, uh, it becomes very complex on the offensive side. Very quickly. And then on the defensive side, you're sort of trying to measure what didn't happen and who gets credit for what didn't happen on the defensive team. Or if there's no credit at all to be awarded to the defensive team, the offensive team, just, you know, failed at executing what is a difficult challenge. The uncontested three-pointer in the NBA is made across the league about 38 and 1.5% of the time. Player misses an open jumper. Did the defense do anything, or is that just one of the just under 2 thirds of shots that guys just miss? And do you get any credit for standing near the guy when he does that? Probably not. So. Those problems, just that sort of problem is really at root of trying to figure out who's good and who's not.
Richie Cotton:
Okay, it does seem like there's maybe a bit of a subjectivity in terms of like how you decide like who gets what sort of credit but it seems like because basketball analytics is getting fairly mature there are a lot of sort of standard statistics that people track to try and measure these things. So can you just give us an overview of like one of those things like you mentioned like the number of shots someone takes but I guess there are a lot more of these things.
Seth Partnow:
So I think that when we're talking about individual players, a place to start is the intersection of kind of scoring volume and efficiency. You know, I said earlier that, you know, you can't always tell how difficult the shots a player is getting based on just the accuracy of those shots. There is a pretty strong correlation in like, the higher the frequency of shots you take, there's a strong tendency for the average difficulty. And certainly the marginal difficulty of the additional shot to be more difficult. As you know, if you think about it, if you only shot wide open layer, put some into the basket, you could probably, you might get to take two or three of those over the course of a 48 minute games. Now say you have to take 25 shots and progressively they get more and more. If you know, you have to fill that quota, they get more and more difficult because the defense is, is doing more and more to try to stop you. So that's sort of on one axis is its usage is the stat. And it's just basically the play ending efforts, whether it's taking a shot, getting fouled, attempting a shot or turning the ball over that a player takes per a given number of scoring chances for their team while they're on the court. And the other level is efficiency, which is how many points are you getting off of those attempts? And... The intersection between those two stats tells you a decent amount about a player. Now, having said all that, it, of course, is highly contextual because the player who might be the best player on one team at a certain level might be the third best player on a better team. And now the mix of shots they're getting for the volume, what will tend to happen there is their volume will decrease and their efficiency will increase because to get back to our, the first thing we talked about, like They're getting easier shots because they're, they're reliant. They're being relied upon less, but still that first, that, that, that the first pass is, you know, this player is, you know, is, is taking, you know, 25% of the team's attempts and doing so at. And a rate of efficiency that that's league average above, below what have you. That, that, that's a good first pass at kind of what a player is doing strictly from a scoring standpoint. And. That's not the entirety of a player's contribution, but that's the biggest chunk of it.
Richie Cotton:
Okay, so do you have examples of like players who were like maybe good on volume but not good on efficiency or like either way around?
Seth Partnow:
Oh, sure. So I mean, one of the one of the the current big examples of someone who is in the technical term as a chucker, someone who takes a lot of shots and sort of over the latter half of his career, Russell Westbrook has been a player who has had very high usage and very middling efficiency and some of that high usage has been the result of of you know, the talent around him and some of it has just been that's kind of the player he is. On the other hand, there's any number of players who have more specialists, not to get too deep in the basketball weeds, but you may have heard the expression of a 3D player. That's sort of a mid-sized player who's a good defender, but they don't necessarily have a lot of skill relative to the rest of the NBA with the ball in their hands. So their offensive role is mainly to stand on the perimeter and wait for the more talented players. to create opportunities, pass them the ball, and then they shoot open three-pointers. Those players, the better of those players, will tend to be low-volume, high-efficiency players, because mainly the shots they're taking are either layups or kind of open, team-created three-pointers, which are, those are two of the higher expectancy kind of types of shots in the game. Um, so that, and then there's, there's a, a, a sort of related player type, which is a certain kind of big man who is basically like a garbage man around the basket who only shoots if he's right at the rim and he can dunk the ball. Again, you have a pretty low usage. You probably, you're maybe using 15% of a team's chances, but you're doing it. So at a very high rate of efficiency, because, uh, you know, dunking the ball is, is, uh, those tend to go in quite a lot.
Richie Cotton:
Yeah, so there's this big trade-off between just like do you take tons and tons of shots even though they're not great chances Or do you just wait for the actual chance? So tactics they’re alright.
Seth Partnow:
No, and it's just this interesting conundrum. And maybe this is getting too in the weeds. But at a certain point, like you're actually, even if your own individual efficiency might drop, you might be hurting the team because maybe you're better at those difficult attempts than the next guy. So you almost become a sin eater almost. Well, someone's gotta do it, might as well be me, even if it hurts my individual stats. It... maybe maximizes the team's overall efficiency.
Richie Cotton:
Okay, yeah, like sometimes you just gotta be the person who takes the shot, right? Okay, so just in terms of like how you go about tracking plays, the new metrics being developed and the different things that are being tracked in terms of player performance compared to historically.
Seth Partnow:
Yeah, so there's there's been sort of three levels of data. Prior to about and this I'm speaking specifically the NBA level, I think that that other other levels of play kind of we are there is the data that's available is is definitely sparser, less reliable. So I'm going to speaking specifically the NBA level of play. Up until around 1996. There was box score data, which is, you know, you, you would know, uh, there's been iterations of it, but the, the, the, the, the version that, that was around for the last couple of years of that era was, you know, how much a guy played, how many shots a guy took and made, how many free throws he took and made some, uh, rebounds assists, steals blocks, turnovers, fouls, you'd know all those things and that's, you can tell some things about that, that gives a reasonable, you know, that, that, that does it. reasonable job of saying who has contributed what on the floor. Then starting in 96 and then probably a couple of years later, the data quality became sufficient to really rely on the NBA started doing detailed play by play. Um, and that meant not only, you know, a little bit more information about not just how many shots did the guy take, but where he took them on the floor. If the shot was assisted, who assisted it? Um, if the shot was blocked, who blocked it? if he was fouled, who fouled him, some of those things. But additionally, since we since it's also tracking substitutions, the key change there was you could know who which 10 players were on the floor for any one game events. And then that allowed you to start doing some correlation doing some some analysis. Okay, well, when this player is on the floor, what happens when this player is off the floor? What happens when these combinations of player and And so that unlocked another level of analysis. And then starting 10 years ago or so, starting for the 2013-14 season, across the league was implemented. It's, it's known as player tracking data. It's essentially a number of, of sophisticated cameras placed in the, uh, the rafters of every arena that allow for players to be identified. Um, uh, 25 times per second in XY and XY location and the ball. And this was another kind of exponential growth in the kind of things that you could know because when we got to play by play, you knew kind of some events that were happening. Now we know a little bit more about why. You can start to see the, okay, you know the dish that's produced when a player, okay, player X made 17 foot jump shot. from this location on the floor assisted by player Y. Okay, we know that. Now with the player tracking data, you're allowed to say, all right, the player who shot the ball, he cut from this spot on the floor and got a received a pick from that this player and the pass was delivered at this time and he caught it. Didn't take a dribble. Defender was however far apart he was closing at whatever rate of speed he was or wasn't closing and then took the shot. And that's, so that's an exponential increase in the amount of data that, that you have and it allows stuff like measuring, uh, we, we, we had a colloquial understanding of, of, you know, uh, maximum basketball is get good shots. Have a pretty good idea of what those are. Uh, but now with this tracking data, you can actually much more directly measure kind of the quality of shot. I mean, if, if, uh, I know you're, you're, you're from the UK. So You're probably more familiar with soccer. It's, it's a similar concept to XG. Like, okay, from this spot in these circumstances, how often is this, is this attempt successful? And, and so for the last 10 years, we've had that, uh, and not just that, that end of the, of the chain, but also all the events that happened beforehand, whether it's a, whether it's a pick, whether it's a drive, how many dribbles, how many passes, uh, how long a player has possessed the ball before all these things happen. All of that is a, is a, just a vast increase in understanding the context of, of how the, the, you know, kind of the possession ending thing happened.
Richie Cotton:
So that's a spam and grubble growth in the use of analytics and just from sort of simple, a few simple metrics up to like really sophisticated, well, yeah, like camera data.
Seth Partnow:
Yeah, I'll add one more by the way. Starting next year, the tracking data, the tracking data currently is just like a dot at center mass of a player starting next year, it's, it's going to be the data at the same frame rate, which is going to be pose data, which I, I forget off the top of my head to how many data points on each player it is, but it's, it's a, some number in the, in the, in the low double digits. I don't remember if it's in the, it's in the. If it's a dozen or two dozen somewhere in that area, but, but so you can track arms, hands, feet, things like that. Um, well now what's going to come out of that data kind of remains to be seen, but it's another exponential increase in the amount of raw information that's out there.
Richie Cotton:
Okay, so I guess that leads to a natural question around like, how do you go about harnessing that? Because once you have these sort of dizzying amounts of data, it's very easy to get lost. I was wondering if you have any thoughts on how this is going to be used or how it's going to become useful.
Seth Partnow:
I mean, so it's, it's, I mean, you can just think about it in the, in the tools that, that you, you had, um, the number of data points when it was the box score, you know, there might be 80, a hundred data points for a game. When you had, when you had play by play data, we're talking a couple hundred data points a game, maybe depending on how you want to, you know, depending on how you want to count them, maybe as many as like 1500 data points a game. With the tracking data, when it's just center mass, now we're talking about 900,000 data points for a game. And with the pose data that's coming in next year, now all of a sudden we're talking, probably getting close to eight figure, up into the tens of millions of data points for games. So just the technology that you would use to be able to address that. that amount, that size of data has obviously changed. You know, back when it was, you know, you could still do all your analysis in Excel, uh, when you were working, even at the play by play level. Um, once you get to the tracking data level, that's just not possible. Now we're talking about database solutions now just to pull the events. Like the, the, the basketball events, the, uh, the CEO of, of second spectrum, which is the company that's, uh, had the rights to the provider of the NBA's tracking data for a number of seasons, describes it as pulling basketball words from the data. To do that, now we're talking about applying some pretty involved algorithmic and machine learning techniques to identify, okay, this pattern of moving dots is a pick and roll. This pattern of moving dots has these features, these characteristics. of a pick and roll, whether it's the players involved or some categorization of, you know, the style of what they're doing and the outcomes. And then I, the, the, the, you get well beyond my expertise to, to, if to start opining about what like, you know, how you, how you actually even start to really glean additional insights when you start to move to the pose data that they're going to, that that's going to be available from next year.
Richie Cotton:
Would that sort of file under fancy deep learning and AI and it's probably going to turn crazy big data into something meaningful on a sort of basketball level? Okay, I actually have a nerdy question about one of the metrics you were talking about in your book. So there's this recurring thing about play impact plus minus, which it seems like... This means a player is better than expected once you sort of fit a model to it. And usually when you're fitting a model, some sort of statistical model, machine learning model, then you look at the predictions. But actually it seems like you fit this model, you discard the model itself and you're just looking at the residuals and that's the player in fact plus minus. So it seems like a sort of backwards way of doing things from a modeling point of view. Could you just tell me a bit more about how this metric works?
Seth Partnow:
Sure, I think you're slightly misunderstanding that particular metric, but there are metrics that apply that way. So let me back up. So I think the basis of this kind of analysis comes from when I talked about the difference between we went from box score data to play by play data. And there's a whole family of kind of metrics called adjusted plus minus. which are, okay, we know the 10 players on the floor, the five on offense and five on defense, and we know the outcome of every possession. That seems like we have enough information to fit a model to, you know, across the however many possessions there are in the NBA season, give or take 250,000, if I'm doing the math in my head right. With the 10 players on the floor, we can fit a model knowing the outcome of those possessions. And what are the exponents of basically each player in that, excuse me, the coefficients on each player, in that model, and that gives us an idea of on a per possession level, this player is worth X points per, or. this fraction of a points per possession. And you know, the better players will be more and less. Now that worked okay, but it turned out that across the 450 players or so in the NBA season at the time, across that number of games, it actually was, the data was too sparse. And what was happening is players who didn't play that much were getting these like huge values one way or another. So then regularization was brought in to sort of pull everyone back to an origin point. Um, and then the next level of that was not just everyone being pulled to the same origin point, but using a statistical prior based on some other information, box score information. Okay. We can go, we have this relatively stable measure of what we think players impact are for, what we think players impacts are now let's use some of this other data, the box score data largely to say, okay, we don't know exactly who to credit this plus or minus two. But using this other data, we can make some inferences about the players who do better on these kind of box score stats tend to also do better on these impact stats. So we can use that to create a statistical prior. So each player is being pulled against. And that's a little bit what you're talking about, is we start with that. The model is almost creating that prior. And then the actual observed, you know, variation from those priors is sort of further assigning the credit or blame from the scoreboard either moving in your team's favor or the other direction. Now, those are great, except those tend to be kind of black boxy and huge sample sizes, and you can't really say in this one game, this player had an impact X, Y, or Z. So what an impact like PIPM does is it then... uses some other external data. Some of it is play by play based, some of it is box score based, some of it is tracking based to kind of create a statistical, kind of a whether it's linear weights or interaction effects, all kinds of those things to then get a sort of an analog, an estimate of a player with these inputs will tend to have you know, this level of impact in that model. So then that allows you to look at a single game or a small subset of games, or value certain actions on the floor. And it's not perfect, but it's actually a little bit more useful because it allows you to kind of make inferences about the mechanism, what this player is doing, that makes them look like a great player or a poor player, which is... to bring it full circle to what analysts within teams are doing. Being able to say, well, he does this, and that is this good. Therefore, we should sign him. We should trade for him. We should draft him. Is far more useful than saying, well, this guy's worth 2 and 1 half points per 100 possessions, so we should get him, because that's a pretty good player. We should get him. It's like, well, what does he do? I don't know. He just did the thing. Good things happen when he's on the floor. That's. not the easiest cell to subject matter experts. So having all that information that we can tie back in to that larger model and kind of on a small scale estimate or make some inferences about the mechanism then allows you to a much more explainable model, even if it might lose a little bit of kind of predictive accuracy.
Richie Cotton:
So really the interpretability and the explainability of these models is very important.
Seth Partnow:
I think it's I very much lean towards interpretability. I quite frequently prefer simpler models that are more explainable. I will gladly trade some degree of accuracy and predictive power. Because I don't think it's hard for them to be actionable. If you don't have that now for other use cases. you know, for maybe for a gambling use case, you might not care. You might, the why might not matter as much. But from the standpoint of giving actionable advice in the team building concept, you know, both from a standpoint of. getting people to listen to what you're saying and also being able to kind of, whether formally or formally adjust for how a player's context might change from the one in which they kind of produced this level of performance. I think that explainability and that decomposability is pretty vital. Have I lost you completely?
Richie Cotton:
Okay. No, no, no. I think. I think. We ended it so it makes sense. It's pretty flat. All right.
Seth Partnow:
Hopefully, hopefully I'm making sense though.
Richie Cotton:
Yeah, so the idea is that if you have a model that's more explainable, then it's gonna be more useful for the people who are making decisions and therefore it's gonna have a bigger impact.
Seth Partnow:
It's certainly going to be an easier sell to the people that end up making the decisions and it's, it's in some cases it's changing, but the people who know how to build and really interpret the models and the people who are making decisions are separate, are not the same people. And so, um, the, the, uh, the convincing part of it is pretty important and explainability goes a long way towards helping with that, with that task of, of saying, no, he is good and here's why you should believe me.
Richie Cotton:
All right, just on related note, I'd like to talk a little bit about incentives related to statistics. So I believe some of the players will get pay bonuses depending on whether they hit some certain metrics on target. And I think like anyone who's worked with a sales team knows that that sort of biases like the behavior to like, oh, I'm just gonna try and hit my target this quarter. And then, you know, who knows what happens next time. And it doesn't necessarily help the whole team. So can you just talk a bit about how this works in basketball?
Seth Partnow:
Um, I mean, I think that if you incentivize hitting certain statistical targets, um, though the, the generation of those statistics is sort of assumed to be on the outgrowth of playing basketball, trying to win. And now in, in, in many cases, those things will be perfectly aligned, but in some cases they won't be, and you can see all kinds of behavior where, well, I'm not really worrying about us winning the game. I'm worrying about me getting this next rebound because that gives me 10 rebounds for a game. And then I have a triple double, which is, which is, you know, a laudable thing in itself itself. And people say, wow, he had a good game when really that extra rebound I got was, was, um, somewhere between inconsequential and perhaps harmful to, to chasing that rebound, maybe not defending my, my own play, my own defensive assignment as closely as possible so I can be near the basket. and be more likely to get this rebound. Maybe that actually hurt my team. So being very careful about the incentives you set up so that as much as possible, chasing the incentive will be indistinguishable from playing a winning, it's a bit amorphous, but playing winning basketball. There'll be, like those incentives will be, occur as a natural outgrowth of playing winning basketball, as opposed to being sort of independent targets that have a tendency to be at odds with that. That sort of overall team goal.
Richie Cotton:
So it seems like this is a sort of standard management thing. It's like, how do you align your, the incentives of your individual players in order to align with the team goals? All right. Nice.
Seth Partnow:
And there's always some tension insofar as it's not an explicit thing, but it's demonstrable that players who score points get paid more. Now,
Richie Cotton:
Okay.
Seth Partnow:
only one player can shoot the ball each time down the floor, but there's a lot of different things that we talked earlier about the way that the various contributions of all players on the floor might affect the ability to score. of the, for the team to score efficiently. Um, but if the individual incentive is, well, if I get buckets, I get paid. There's the tension there is already, it's, it's, it's obvious. Like there's, there's five guys who each know that, that, that if they do these things, that they might get paid more and trying to channel that so that they, um, that goal exists alongside of kind of the broader team goals. Um, and is secondary to. the broader team goals is sort of that's the it's not a statistical thing, but that's certainly like, that's why coaching is hard. You're basically you know okay this thing might not be the best thing for your for your very narrow self interest, but it's better for all of us. And if I can convince you that being better for all of us is then okay you may get a smaller slice of the pie, but the pie itself is bigger so it's actually good that you do this. That's not really a statistical argument. That's a sort of a psychology. But that's where the psychology and the incentives sort of overlap and create kind of what could be these misaligned incentives, which lead to counterproductive from the standpoint of the team behavior.
Richie Cotton:
Yeah, so it's like rather than just trying to look cool on TV on the game or whatever It's like you actually want the whole team to look cool.
Seth Partnow:
There's a specific example I use in the book. And it's kind of it's like these funny edge cases always come up like you like. What is generally like a just a straight performance incentive? Like the player, the example I used in the book is a is a player who was not a great shooter, had incentives in his contract to make a certain percentage of his three pointers. Well, he's not gonna try to miss three pointers. So it's just like you play well, you get paid more. It's fairly straight like that, except it kind of came down to the end of the season and he was just above that threshold. And so the best way to make sure he ended the season above that threshold was just to not shoot anymore, give himself no chances to like miss enough shots to fall below that threshold. And so then, okay, if his job on offense is to be one of those, we talked, you know, again, earlier about the 3D player. Well, he's not going to do that anymore, because he didn't want to catch the ball there, because he just want to take that shot. Because if he misses that shot, he might cost himself several hundred thousand dollars. Okay. Like that was that that's a situation where kind of everyone on the team is like, you get your money and we're fine with it. But it's also, it's also understood that that's not. If you were playing strictly to maximize the team efficiency. Well, I don't care if you missed the shot and you go in, you go, you need to stand there and space the floor. So, so that the whole offense works, works properly. But that's it. Like that's a very, that's an odd edge case to what is broadly speaking, a properly incentivized. you know, play better, get paid more kind of, kind of incentive.
Richie Cotton:
Okay, that's a cool story and I hope he did take some shots in the end and get paid.
Seth Partnow:
No, he he he though the intro. I mean, again, the interesting thing is is he didn't even he he didn't even stand in the spot on the floor where you would be asked to take those shots. He's like, no, I'm not. I'm not going to I'm not catching the ball in the corner because I might have to shoot then. So I'm going to stand out everywhere else but there.
Richie Cotton:
Wow, okay, some bad behaviour there. All right, so I'd like to talk a little bit about how basketball is changing. And what I think of what makes a good basketball player, like the obvious thing is they have to be really tall, but you make a case in your book that actually height's been becoming less important at basketball since about 1980. So the era of the big man that sort of ended at that point. I'm just wondering why you think that's been the case?
Seth Partnow:
I'm so height is becoming less important only in relative terms. It's sort of, if you want to, you could be reductive and say, like the two traits in basketball players are skill and size. Obviously there's a lot more than that. Each of those things has more thing more, but because of rule changes, because of style of play, because of realization. Um, of the value of certain areas on the floor relative to others, the perceived and probably actual returns to skill have increased relative to size.
Richie Cotton:
Okay, so maybe height is as important as it was, skills become relatively more important to compensate. So, go on.
Seth Partnow:
No, and it's there and there's some some demographic aspect of it too, in that like, if you need a certain level of skill, the certainly once you get to NBA size, every kind of additional inch in height is a drastic reduction in the in the number, the sheer number of people of people, not just players who kind of fit that bill. So naturally, like there are far more six footers in the world than seven footers. So if the key thing that you're indexing on is a certain skill-based ability, you're much more likely to find a six footer that can do it than a seven footer. Now, height is still important enough in basketball that you actually don't. In most cases, you don't actually want the six footer, no matter how good they are at whatever the skill is. So you do stand to move up higher, but the, again, the relative impact of a guy who is 6'6", with certain skills and a guy who's 7' without those skills, has moved more towards the smaller player with the skill. Again, based on rule changes and the competitive environment and the dominant strategies and all those things.
Richie Cotton:
Okay, so there's some sort of optimization process and it's shifted slightly from height to skill. The other notable change seems to be that it's been like the rise of the three-pointer shot. That's a lot more three-pointer shots being taken than two-pointers. And beyond the obvious three-pointers more than two. Why do you think this has come about?
Seth Partnow:
So I think that a key point, a key inflection point from this was the development of the, was the implementation of the play-by-play data. Um, there's, there's a sort of a, a bit of conventional wisdom. That's like, all right, you're not a great three-point shooter. Take a, take, take one dribble and get an easier shot. Now it stands to reason. And it's true that taking a shot 19 feet away from the basket is going to go in more than taking a shot from 24 feet away from the basket. Um, and you know, for the entirety of the time that, that we've had, uh, the three point shot in the NBA two point shots have been made at a much higher percentage than three point shots. Um, maybe not at the, at, you know, when you factor in the extra point, maybe not at the level of the higher level of efficiency. Um, but that's, that is a plausible story to tell that even accounting for the extra point, you will make the 19 foot or more. More more often. at a higher rate more often than the 24 footer that even the extra point does not overcome that increase in efficiency, that increase in accuracy. Now empirically what we found is that's not true. Once we had detailed location on where shots were taken from, you kind of see that shots near the basket go in a whole lot, it declines very quickly until you get out to about five feet. And then once you get out to about nine feet from the basket, it basically stays more like pretty level declining slowly until you get out about 27 feet away from the basket. So yeah, you might make the 19 footer two percentage point higher rate than the 24 footer. the extra point swamps that, that of the three pointer, swamps that advantage. Um, so it's, it's a, really the empirical recognition that relative to shots, just a little bit closer, the three-pointer is better. That's one thing. And then you realize also, Oh, so if I'm not doing anything, the better place for me to stand isn't 19 feet away from the basket, it's 24 feet away from the basket. And then that has some knock on effects in terms of, well, my defender has to stand further away from the basket too. So the person with the ball who's, who's more skilled and is trying to get to the basket because shots right at the rim are the most efficient. I've basically created more space on the court for them also. Um, so it's, it's, it's that kind of changing of the, the geometry, the, uh, the, the topography, if you will, of, of the court that was, uh, unleashed by the kind of the empirical understanding. And there were people who even in the absence of data sort of intuited this by observation. But with the data, it's demonstrable that, well, that's it's no, there are very few players for whom the shot from that one step further in is actually worth doing than the shot from further out. But until we had that data. It was an argument that was plausible either way.
Richie Cotton:
That's cool that you actually need the data in order to make the decision about that. Like a pretty major strategic component of what you're doing there. And I do find it amazing that there is such a drop-off in terms of the shot success by distance. You'd imagine it'd be fairly linear, but you're saying it actually just drops off very quickly once you get a few feet away from the basket.
Seth Partnow:
I mean, essentially, once you're shooting jump shots under NBA conditions, the accuracy tends to be in the low 40s to mid 30s. When you're closer to the basket and you have more of a variety of ways you can shoot the ball, whether it's use either hand or full flip shots, use the, what have you, to avoid the defender, that's where, or just take the ball and dunk it in the basket, which is the easiest shot. Again, height is good because it's easier to dunk if you're tall. Again, and being slightly reductive, but I think that's sort of the mechanism we're talking about is when you're close enough that you have that variety at your disposal, it's easier than if you, well, I'm gonna shoot a jump shot now because I'm far enough away from the basket, that's the only way to really do it.
Richie Cotton:
Okay, just related to this, with the sort of, the increase in, or this new strategy where you're going for more three-pointers, has that decreased the amount of like, the fancy photogenic dunks that you get in the game?
Seth Partnow:
It has not. That's an interesting sort of. Um, the aspect of it is the, a misnomer, kind of a misnomer of the rise in the three pointer is like teams are shooting a lot more jump shots than they used to be. Really, if you look at it, teams are shooting about the same number of jump shots as they always did. Same number, same proportion of shots near the rim. It's just the character of the, the, the jump shots has changed. And so you're getting about the same number of shots at the basket about, uh, I don't know, we don't have, we don't have detailed enough data to know how frequent dunks were. in, uh, you know, in, in 1984 or whatever. But I think that the, the rate of dunks has stayed relatively stable. Certainly the rate of, of, of, you know, layup attempts shot, like shots at the basket has stayed relatively stable over the last 25 years, even as the proportion of the rest of the shots that being three pointers has skyrocketed.
Richie Cotton:
Okay, that's good to know. And are there any other ways you think that analytics has changed the game of basketball?
Seth Partnow:
Oh gosh. Um, I mean, I think in many ways it has, um, just, I think related to that, there are certain types of ways to attack a defense that have been empirically shown to be more or less efficient than, than others. Um, now whether or not those that that's strictly an analytic, like this is where it gets tricky because is that an analytical thing, is it a rules based thing? Um, There are certain ways of defending because the way the rules change, you used to be able to defend certain ways that you can't do anymore. And that makes certain, I think fairly obviously will make certain strategies more or less viable than they used to be. And maybe determining the, you can probably more swiftly determine the efficacy of various, of new strategies using statistical analysis than it would, than you would maybe by long-term trial and error. So I think that's certainly one way where sort of the speed of discovery has probably increased because of being able to, you know, the, the, if I'm watching film to try to figure, if I'm watching a game tape to try to figure this out, I can watch about maybe a game an hour, probably a little less if I'm giving it real attention. If I'm using statistical analysis, I can watch every game from last night in five minutes. maybe at a lower level of detail, but to find out, like, if I've defined these events, these strategies, correctly in the data, it's a press of a button, and I can know a lot very quickly. I can look at several seasons of data, of several thousand games worth of trials in a few minutes versus something that would be essentially impossible. for a human to do in a more analog fashion. So I think that's, that has certainly, the speed of kind of exploration, I think, has definitely increased because of this.
Richie Cotton:
I'm sure like if you haven't watched videos of games then yeah there's a limit to the number of videos you can watch in any given time so I've done automatically seems like a huge improvement. I'm wondering are there any big challenges left in baseball? Sorry, baseball, basketball analytics? What's still to be worked on?
Seth Partnow:
I mean, the whole analysis of defense is still very difficult. Again, you're sort of, you're almost inherently looking at counterfactuals. Um, defenses is as much about changing the decisions an offense makes. As it is about the outcome of what they, of, because as, as, you know, we, as I said earlier, like sometimes guys just miss shots and even over the course of a season, the sort of the, the natural variance is going to in many cases overwhelm kind of whatever defensive skill might have been involved there. Um, but if we are, if we can look at the choices that, that an offense makes, the offensive, the process by which they follow and see those things change, then we can maybe start to really directly, um, measure what a defense is doing instead of just doing so kind of by, by imputation of kind of what happens to the offense when they're there. But that's really difficult. Any sort of analysis of counterfactual analysis of what would have happened had the player with the ball done x instead of y. The level of complexity there becomes very quick, very fast. And so all of which is a very long-winded way of saying, defense is hard, especially crediting individuals for the defensive outcome. that their team the defensive levels of achievement their team is seeing
Richie Cotton:
Okay, we've come back to that attribution problem again, is trying to work out who did what and why. Okay.
Seth Partnow:
Well, I mean, that's, I mean, that's on some level, that's the whole game. Um, because, you know, okay, we had success. Why if we want to have more success, what, what should we change? If we change that one thing, what are we losing in other areas? Like these are, you know, these, this is the, the difficulty of it because it's not just, you're not just like adding a widget and, and it's purely additive. Like you're removing a piece. You're, you're doing a different strategy. You're playing different players. So. You're not just the benefits of the new piece, but the, what you're losing from the old piece and it's, you know, it's multifactorial. It's the, it's, it's in all these different areas. This player does interesting things and we're not, we're nowhere close to really understanding what all those are in some areas, we have a close enough understanding that we can make some reasonable predictions about it, but I don't think that's anywhere close to saying the whole thing is solved.
Richie Cotton:
I suppose if it was solved then every team would do the same thing and then it becomes on 12 again so maybe it's an impossible problem.
Seth Partnow:
It’d be pretty boring. Yeah.
Richie Cotton:
Okay.
Seth Partnow:
I hope so. Because it's more interesting as long as there's always more to learn.
Richie Cotton:
So I'd like to talk a little bit about careers in sports analytics. So maybe you can tell us how you got into basketball analytics.
Seth Partnow:
Uh, by blind chance and luck. Um, I, I think, um, certainly I'm of the generation of kind of the early enough generation of people who work in the field that I kind of stumbled into it from doing other things and there are much more defined pathways to getting in now, uh, for better and worse, I think largely for worse, if I'm honest. Um, But I did a number of different things. I graduated from undergrad right as the first dot com boom was kind of crashing. The first dot com bubble was bursting. So I worked for an e-commerce startup, which failed after I had been there for a little bit. And then my first job ended with a layoff. And I went back to school because I didn't know what else to do. So I became a lawyer. because I had did not have the inventiveness to think of another anything else to go back to school for, to get my JD and then I practiced law for a little bit and hated it. And while I was in law school, I had learned that I had a I like, so my relative to people who do these kind of jobs, my my level of formal statistical training is pretty low. But I played poker professionally for I had a talent for for estimating distributions essentially, which I discovered. And gambling for a living is a graduate level, perhaps even PhD level crash course in applied statistics, if not formal statistics. If you're going to be successful, you have to have to develop that statistical intuition. So I did that for several years. And then I moved into sort of educational consulting. And while doing that, which was doing project evaluation. And interestingly, a lot of the stuff we talked about earlier in terms of incentives, like figuring out the best way to set up an educational program and then to set the goals in such a way that you're trying to incentivize the right behaviors rather than just sort of ticking boxes and teaching to the test. That was. I, that was certainly a concern there for a long time. Um, while I was doing that, I started like writing about basketball on the side. I, I played small college basketball. Um, and so I've always been involved in the game. I played a lot growing up. I've always been interested in the statistical side of sports. Um, and so I just, you know, started my own website and started writing. And, uh, this was around the time when the NBA was releasing the first, uh, sort of And because I think the combination of my statistical intuition and my kind of basketball as basketball experience and knowledge, I think, I don't, I don't think I'm being arrogant by saying I was doing more with that new data than, than most people in the public sphere were. And sort of from that, I got, you know, both better opportunities to write and do analysis for, for larger outlets, but also. started to get contacted by teams. And, and, you know, I was old enough at the time that I was not going to take an entry level job. Um, and I, so, but the, the, the, the team that eventually hired me, the Milwaukee bucks like created a role for me that was sort of commensurate with someone, you know, middle of the middle of their career rather than at the start of their career. Um, and so that's, that's sort of how that all came to be was, um, a very windy, non replicable. certainly nonreplicable in today's environment now that not just sports analytics, but data science and analytics in general is a much more formalized field of study. So that's how I got there. I don't know how useful that is necessarily to other people, other than saying that the parts that have served me best in that career are the practical. subject matter training that I've kind of gotten from whether it's basketball, whether it's, you know, learning kind of hard lessons about incentives in other fields have served me well in ways that that pure training in whether it's you know, statistical programming or, you know, formal statistics and machine learning and stuff might would not have.
Richie Cotton:
So I do actually love that you've got this winding path to getting into data. And it seems like it's very common that people discover midlife that, oh, actually, I really do enjoy working with data and end up in the field. I'm mildly worried it's gonna corrupt our listenership just with deciding to go, oh, I want to work in data, I'll go and be a professional gambler. So for any of our listeners who want to jump in basketball and athletics, do you have any advice on what skills they need?
Seth Partnow:
So there's several different answers to that question. The first one is a little bit of introspection about what they mean when they say they want to work in basketball or sports analytics. There's sort of two different questions there. Do you want to work in sports and sports analytics, or do you want to work for a team on the sports side? One of those is a much larger target to aim at than the other. And also is sort of the costs of doing so and of succeeding is lower. Because working in, whether it's the business side of a sports team or as a data, working for a data provider as I do now, is much closer to kind of a. stable, you know, stable workday work life balance, plan your vacations, do all these, do all the normal career stuff. Whereas it's almost like working for a team is, you're almost working chef hours. You know, you're, when I worked for the Bucks, I went to I went to 41 home games a year. That's 41 nights that I, that basically like there, like my work day did not end at like, not that my work day ever ended at five anyway, but like my work day ended at 11. Uh, and maybe later if something happened in the game that needed like an immediate response. Um, and really, and, and considering there was 41 home games and it might even happen on a road game, like even if I was at home watching on TV, I'm still kind of at work. And really, when you're working for a sports team, you're kind of always kind of at work, even when you're not at work, because things come up at any given time. Which isn't in any way trying to dissuade people from chasing that if that's what they wanna do. It's just sort of understanding that's the choice that you're making, and being kind of upfront about, are there rewards to that in terms of being in a competitive, high performance environment? Is that your thing enough? for it to be worth it. So that's the first thing I said, that has nothing to do with technical skills or anything like that. That's just, you know, setting appropriate career goals aside from that, I think is very important. Cause a lot of what is very common for people to land one of these jobs and then start to do the job. And it's like, this kind of actually sucks from a life perspective. And that's no good for anybody. It's no good for the team that hired you. It's no good for you. So trying to... If that's not going to be your thing, don't do it. Now. And that's probably good career advice regardless of anything. But I think in this field, it's worth pointing out. Now, from a more job skill standpoint, sports analytics are becoming mature enough that a lot of the roles that one would see in a technologically-based role are starting to see. I mean, it's. teams are now hiring for data engineers and they're hiring for analysts. They're hiring for front end and back end developers. They're hiring for all of these different roles that touch data. And so finding your lane in that is from a standpoint of identifying what job you're going to chase is the next most important thing to do. What is your competitive advantage on the hundreds of people that apply for any of these jobs with the team? And if you wanna be a data engineer, like if that's your skill set, then like focus on that. If it's more on the analysis side, then honing not just your statistical techniques, but your communication ability, whether it's written, whether it's verbal, whether it's. you know, doing it, writing a bulleted deck, whether it's a data visualization, stuff like that, that's as much, that's a communication job as much as it is a technical job. Um, and you know, the closer you are to that, the more the subject matter knowledge of the sport becomes important. On a certain level, you're setting up a data pipeline data as data is data. Um, it certainly will help to, to, to know kind of the, the, what to expect from the structure of data you're, you're getting. from a given sport, but it's not as necessary. If you're in a standpoint where you're going to be, you know, presenting to coaches and front office executives your findings, you better have the terminology, right? Otherwise, you know, there's no quicker way to kind of lose buy-in than, you know, we, it's funny, we talked beforehand, you were asking me, like, is it basketball teams or basketball clubs? You start talking about something as simple as like, well, We're trying to catch this club. It's like, that's not the right word. Like it's silly, but like that's a pretty quick way to lose buy-in. So that in parallel with kind of the technical skills is important. And I think there's, even though it's probably more, so in sports and other industries, I think there is an important more broadly applicable. lesson about the importance of having that knowledge of the subject matter, just so you you're aware of what the data you're working with actually says actually represents it's an abstraction of the of the of reality. What's what is it abstracting away? What is it capturing? What isn't it capturing? I think that on having a decent level of understanding of that. really gives you the first level of sniff test of almost QA-ing your own work, you get a result. That doesn't make sense. I did something wrong. My data is bad. Let me go examine that again before I, look what I found. And then it was you reversed a sign somewhere in your code or the data was faulty in some ways. That is... I mean, you're in a garbage in garbage out scenario then and being able to catch that yourself can save you a lot of pain. Um, so, you know, I guess if I was going to reduce what I just said to two, two maximums, it would be like, pick your lane and learn what, learn about the thing you're talking about, learn about the thing you want to talk about. So we can talk about intelligently.
Richie Cotton:
I mean, it does seem like there's a pretty sort of broad set of skills you need. You need the technical skills, communication skills, you need all the sports knowledge. And it sounds like particularly if you want to work for a team, you've got to really, really love the game. And it's more of a lifestyle than a job.
Seth Partnow:
I think that's a great way of putting it. And I will say teams, like for a long time, teams were not very good at when they put, you know, job descriptions out. They weren't very good at describing what they were actually looking for. Cause it was like, you know, a full stack developer with, you know, eight languages and this and that, and this and that we're going to pay you $40,000. That's not really the case anymore. They, they, I think the teams have become mature enough that they can start to. No, what we actually need is an analyst. What we actually need is an engineer. What we actually need is a backend developer and, you know, putting job requirements that increasingly though not perfectly reflect the tech stack they're actually working with. So finding a job description that actually matches skills you have is or can work towards is a little bit more of a guide than it might have been even five years ago.
Richie Cotton:
Well, that's very promising. Alright, so we should actually talk a little bit about what's going on. right now means middle of May. So have you been involved in any analysis for the playoffs this year?
Seth Partnow:
Uh, it's, this is the, this is the best worst time of year because it's, it's, there's a ton of games happening and they're all interesting and meaningful. So yes, I've been, uh, we're at the point where we're recording on, uh, the, the, the day after the first game of the conference finals. So we're finally at the point where we're down to no more than one game a day, uh, which is sort of, uh, when, when, when work-life balance can, can return or, or when, when some balance can return to, to, to the force a little bit. Um, but yeah, no, I mean, it's, it's doing, uh, watching in detail and analyzing kind of across the, the first two rounds of the playoffs. Um, it's, it's, uh, now that I no longer work for a team and, and don't, and I'm not responsible for kind of day to day content, uh, produced off of basketball. It's, it's kind of, kind of come full circle into like almost a, uh, a semi-professional hobby to, uh, to, to analyze what's going on now.
Richie Cotton:
Okay, have there been any kind of interesting data moments, any upsets or things like that, or remarkable stats coming out for the games?
Seth Partnow:
Um, so sort of an it's a, um, An interesting kind of under tone, under background bit of NBA analysis is. Um, the, the, the, the style of play, the strategy of play, um, the value of certain players has always diverged between regular season and playoffs. To some degree. Um, you know, the, the, it's very different, you know, preparing to play one team seven times across a couple of weeks as it is preparing to play against, you know, a different opponent every other night, and sometimes even consecutive nights. And the level of detail you can get into about what a team, a player is or is not good at, will or won't do, just allows you to do different things. And second of all, just like, you know, when you cut off, you know, to go from regular season to playoff, you're basically eliminating half the teams. So, you know, it's maybe it's not one to one. It's not just the top half of players. But your average opponent is much better in the playoffs than they are in the regular season. So, you know, whether your skill set translates as well against better opposition as it does against worse. So there's always been this divergence between regular season and playoff play. I think over the last several years, and why that could be is a whole other several hour podcast, but that divergence has only increased. And this year, it almost feels like we're at the, at a peak of, of regular season and playoff divergence. Don't though, maybe not because this was a year where we're sort of in a transition period in the NBA where. Kind of the, the previous dynasties have largely run their course and the, the newer ones haven't quite risen up. So this was the most compressed. that the league was kind of top to bottom of relevant teams in terms of how good they were, which is in many ways, I think fits well with this, this, these playoffs being kind of less predictable. Maybe they were predictable in the fact that like teams were closer. So things were much closer to coin flip than they normally are. And when things are closer to coin flip, weird stuff happens and you shouldn't be surprised by it. So either the regular season was not very predictive of what would happen in the playoffs if you look at sort of the rank ordering of teams, or it was very predictable that weird things would happen if you looked at like the narrowness of the distribution between teams.
Richie Cotton:
OK, and I guess that's the most exciting time when all the teams are pretty close and it does become more unexpected. So it sounds like some great games are coming up. What's the data showing? Which teams are looking- I was thinking, what's the data showing?
Seth Partnow:
Yeah it's unexpected. I was gonna say it's exciting, but it also has a tendency to make you feel stupid. Because it's like I haven't I, you know, I've made all these predictions, and they're all wrong, except for the ones that are right. But there's still a lot of them are wrong. So do I know anything? Or is it just weird? Um, in terms of what is the day? I mean, I don't know. That's a like Billy Bean, it was quoted in Moneyball, which is obviously a fairly seminal text in the development of sports analytics. He's quoted as saying my shit doesn't work in the playoffs. And it's and there's something to that because again, it's a inherently the sample size are smaller, be their bias sample sizes, because you're only playing against one opponent instead of the league as a whole. And see, it's just that the style of play is kind of weird and more intense and, and, and, and kind of things, things that sort of can just happen. And you kind of brush off over the course of the season, all that'll even itself out, um, losing an extra game here or there means is the difference between winning and losing a series advancing or not winning a championship and not. So, um, what do we make of those statistically? That's always tough. Like you can maybe. We'll say this, well, this team made a historically high rate of shots in this game, and that's why they won. That's neat, that's interesting. What does that say about next game? You made shots tonight, you might not make them tomorrow night and we have no idea why. I just kind of have to live with that and kind of hang on for the ride. That's not a very satisfying data answer, but learning to say I don't know is pretty powerful. And when we're like, you know, you have game one of the Eastern Conference finals tonight. Uh, on paper, the Boston Celtics are much superior to the Miami heat. Miami heat have been a better team over the playoffs than the Celtics have. Uh, which one of those is going to hold sway? I don't know. And even if, and even like, and even figuring out once we actually see what happened, trying to unpack why it happened will be, will be at its own challenge.
Richie Cotton:
Sounds like it could be anyone's final lent. So really exciting stuff. Okay. All right. So with that, thank you very much, Seth. It's been great having you on the show.
Seth Partnow:
Oh, thank you for having me. Hopefully I didn't become too much of a windbag for your listeners.
Richie Cotton:
That was absolutely fine. Perfect.
blog
How Data Science is Changing Soccer
blog
Sports Analytics: How Different Sports Use Data Analytics
podcast
Data Science & AI in the Gaming Industry
podcast
Data Science at McKinsey
podcast
Data Science, Gambling and Bookmaking
podcast