[AI and the Modern Data Stack] How Databricks is Transforming Data Warehousing and AI with Ari Kaplan, Head Evangelist & Robin Sutara, Field CTO at Databricks
Ari is "The Real Moneyball guy" - the popular movie was partly based on his analytical innovations in Major League Baseball. He is a leading influencer in analytics, artificial intelligence, data science, and high-growth business innovation.
Ari was previously the Global AI Evangelist at DataRobot, Nielsen’s regional VP of Analytics, Caltech Alumni of the Decade, President Emeritus of the worldwide Independent Oracle Users Group, on Intel’s AI Board of Advisors, Sports Illustrated Top Ten GM Candidate, an IBM Watson Celebrity Data Scientist, and on the Crain’s Chicago 40 Under 40. He's also written 5 books on analytics, databases, and baseball.
Robin is the Field CTO at Databricks. She has consulted with hundreds of organizations on data strategy, data culture, and building diverse data teams. Robin has had an eclectic career path in technical and business functions with more than two decades in tech companies, including Microsoft and Databricks. She also has achieved multiple academic accomplishments from her juris doctorate to a masters in law to engineering leadership. From her first technical role as an entry-level consumer support engineer to her current role in the C-Suite, Robin supports creating an inclusive workplace and is the current co-chair of Women in Data Safety Committee. She was also recognized in 2023 as a Top 20 Women in Data and Tech, as well as DataIQ 100 Most Influential People in Data.
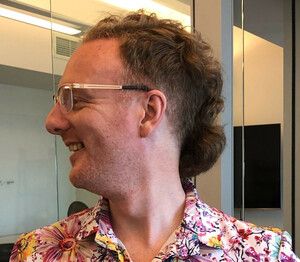
Richie helps individuals and organizations get better at using data and AI. He's been a data scientist since before it was called data science, and has written two books and created many DataCamp courses on the subject. He is a host of the DataFramed podcast, and runs DataCamp's webinar program.
Key Quotes
People are not going to be displaced by AI. AI is just going to augment and make you more efficient and capable to deliver faster. And the other thing is, I think we need more people with creativity, which is a great way for us to introduce diversity into these roles, right? People with different backgrounds, with business backgrounds, with finance backgrounds are now going to become data people because AI now exposes the technology to people who in the past would have never viewed themselves as technologists, but your programming language now becomes natural language. So everybody is going to become a data person.
My own three children, they're like, hey, if I want to go into programming, should I learn Python? Should I learn R? Maybe a year or two from now might be predictable, but four years, it's going to be hard. So, you know, the value will be people who understand the concepts like what is data? How does data relate to the real world? You know, business logic, but then foundations of math and data science, probability and statistics. You're going to need to understand the context. So if you have a choice of 10 different models, you're going to want to understand what's going on in the creation of the model, but from a math or probability standpoint, how does that relate? Also understanding the business is really important.
Key Takeaways
Great data and AI require you to consider people, processes, and platforms - technology is just an enabler.
Incorporating both structured and unstructured data into your analyses can provide a more comprehensive understanding of complex real-world phenomena, enhancing the quality of insights derived.
Data Intelligence platforms are the next paradigm in getting insights intelligently from your data, code assistance, and workflow automation.
Links From The Show
Transcript
Richie Cotton: Hi there, Ari, and hi there, Robin. Great to have you both on the show.
Ari Kaplan: Hey, Richie. Hey, Robin.
Robin Sutara: Hi, lovely to see you both.
Richie Cotton: So, I'd love to start with a little bit of context on Databricks and I remember back in the old days, you started out as this platform for using the Spark big data tool. And that's a long time ago. So Databricks has grown a lot since then. Can you just tell me what's the state of Databricks now?
Ari Kaplan: Yeah, Databricks. Super exciting time for us to be here. We are known as the creator of data intelligence platform, and we'll get a little bit into there, but it's helping companies get actionable insights. Gen AI data analytics from there were known as the data and AI company and very well known for also having created the lake house technology, which is the merging of traditional data warehouses and machine learning unstructured data in data lakes.
And you had mentioned Spark. So one other great thing with Databricks is our founders. Super cool folks. They started in the technology realm and very well known in the open source community, creating Apache Spark, Delta Lake, ML Flow, which remarkably is getting hundreds of millions of downloads every single year.
So that's really like the underlying foundation. Love to get more into that. But, since we've been growing extremely rapidly, we're now something like the third largest. Our fourth largest private tech company in the world.... See more
Richie Cotton: lots of exciting technology stuff to cover there. So data intelligence and Lakehouse and things like that. And even Spark before we get into that can you tell me about like, who your customers are, like who needs this sort of solution?
Robin Sutara: Maybe let me take a stab at that one and then Ari would love to get your perspective as well. so I'm one of the field CTOs for Databricks, and so I have the distinct pleasure of getting to work with hundreds of customers every year as they think about how are they going to use Databricks technologies and Databricks platforms?
super interesting to me and that I would say that there's no single vertical that we work in. We actually work across every sort of industry and vertical globally. So everything from financial services to retail, our customers are the retailers, financial services and public sector. Healthcare communications, media.
can't think of a single industry across the world where they don't care about their data, right? And how are they trying to drive insights out of their data? How are they using their data to provide better services for their customers, for their citizens? How do they actually optimize their operations?
How do they drive efficiencies, particularly in today's financial climate? How do you react? respond to things like global unrest or civil unrest across the world. How do you respond to the, the right, the disruption for your supply chain? So every organization across the world is trying to figure out how do we get more effective.
And how do we now leverage generative AI and, and sort of this hyper awareness that, our consumers and our customers, as well as our employees are seeing with the rise of chat GPT and sort of LLMs now becoming very, or sorry, large language models, becoming very accessible to everybody.
I can't think of a single organization in the world that isn't thinking today about. You know, We have such a plethora of data. That data is very valuable for us. It's what gives us our competitive advantage. It's our intellectual property. And now, how do we take that to the next level? And so when you say, who are our customers?
Everybody's our customer, right? We want to make sure that everybody is being successful using their data to deliver those that they're looking to drive and achieve. Ari, anything you would want to add to that?
Ari Kaplan: Yeah, I'd add. So Robin and I have some fun jobs. Rob, we both travel the world. We're out there talking to customers and partners, getting feedback of what are the actual use cases? And what are the art of the possible and bringing back to product everything? So on one hand, Databricks is like horizontal, kind of like the plumbing to enable workflows, machine learning, and Gen AI.
We also do have solution, like industry vertical focuses and, what are called solution accelerators. So if you're looking to do like a marketing mix or sales forecasting, there are solution accelerators that get you a large amount of the way there. and then also on the size of the company you can be a small company and do what's called like pay as you go.
So just to get started, just to dip your toe in, get out. Or you can be a major company with, thousands of data scientists or data engineers. And, have a larger commit. So It could be horizontal, it could be small to large, or it could be a industry. But yeah, as Robin said, every single company that has data and who doesn't these days can will benefit.
Richie Cotton: I love that the answer is really like. Every single company needs to care about getting insights from their data and they need to care about AI as well. So, this is just broadly applicable to everyone. So actually on that subject it seems that one of the big sort of pitches for Databricks is that you have data and AI in the same place.
Why is that a good thing?
Ari Kaplan: Sure. So one thing that comes to mind is the term multimodal, which is a term that means you can have structured data like numbers and date fields, historical information, and you can also have unstructured data, things like videos, audio, PDF files, social media, real time streaming. And the reason that's important.
That you want to have in many cases, a variety of data is that helps you the insights. The real world is complex. There's human behavior. There's ways things interact, and the more variety of types of data you have, generally speaking, that will give you more realistic answers, more realistic strategy.
and so having structured and unstructured, like I came from the sports industry, if you can have written reports by scouts that are human observing, and then you can have biomechanical metrics you can have end results. If you put that all together, then these insights are more practical. So that, that's why you want that whole variety of types of data in there.
Yeah,
Richie Cotton: there is a definite conception that data science is all about working with numbers, but that's really no longer true. It's like you do have this text data, you've got image data, you've got all sorts of other things as well in there. And I think we've been remiss not to talk about generative AI.
It's the hottest topic of the last year or so. And so, What sort of generative AI applications are you seeing your customers build? Like, who is building this?
Robin Sutara: Yeah, I think it's super interesting. don't think there is a shortage of use cases for organizations, right? As Aria mentioned, we get to speak to lots of customers and fly all over the world. Every time I talk to the board or to the CEO, they give me this huge list. of use cases that they have come up with on how they want to leverage generative AI.
I think there's a couple of things, though, that organizations tend to forget about. One is you need the foundations in place You need to have a good handle on your data. Your AI is only going to be as good as your data is. So if you haven't thought about things like Data governance, data quality as Ari was mentioning, right?
We're built on the lake house paradigm because we believe that this structured and unstructured data needs to have simplicity because you have costs and efficiency issues et cetera, when you start to have complex ecosystems. And so how do you really think about having those foundations in place to be able to leverage against those generative AI?
Once an organization has those foundations in place, it's amazing what they're being able to unlock and uncover, leveraging generative AI. It's everything from how they're delivering health services to their citizens and being able to drive that more efficiently. It's also commercial benefits, right?
Where organizations are saying I'm an airline and I can now have my, Customers interact directly with our back ecosystems when things like aircraft maintenance or weather, et cetera, is going to delay a flight. I no longer have to have 25 representative answering phones to be able to re pivot or reassign, reallocate these customers to different flights.
Customers can now self serve because they have access to these generative AI systems on the back end to be able to deliver against that. And so I think there's, commercial benefits, their societal benefits. We're definitely seeing, I think, use cases continue to expand, but it really becomes an issue of can you prioritize the list of use cases that your organization has come up with?
And and do you have the right foundations in place to actually be able to deliver against that value that you're looking to drive for the organization?
Richie Cotton: I really like that idea that these applications that are going to improve the customer experience or improve society in general. So I think that tricky part you mentioned there was like, how do you it? What's the most important thing to build? Ari, do you have any opinions on this?
Ari Kaplan: this question holds true no matter what it is, whether it's been traditional projects and data, whether it's machine learning, artificial intelligence and data science, which are typically how can you predict something into the future based on past evidence, or whether it's the newish, like about a year now on gen AI and typically the types of Priorities they have are, the common one is like chat GPT like things, which is like a chat bot or text, but other really fascinating use cases are coming out like internally at Databricks.
We have this, the whole data intelligence platform, but Databricks assistant, for example, you're writing Python code. People may not realize that this is like a whole thing. It's going to change the way software developments made, but you can Yeah. Automatically document your code, put in comments, you can troubleshoot.
My own daughter does this in college, like there's a bug. You just hit the AI button and it'll like give you advice and feedback on what's wrong with the syntax. If you want to use AI to search for your assets and not the traditional keyword search. But in addition to that, like context, like what is churn?
Churn may not have the exact letters C H U R N, but it a different context. So those are like ways that AI is being embedded in applications, the way it's being embedded in code. But then the chatbots, but then another big one is just like summarization, like you have a bunch of documentation, just summarize what, what's the main points and, five bullet points.
So that's another like great suite of use cases and every single company that we're talking to is at least like. Curious, they want to know what's possible. Most of them are actively working towards it. We're still at the phase where it's not like not every company has a whole buzzing full of LLMs out there working.
But this is the year, I think it's going to take a huge step many, many companies getting to that maturity stage.
Robin Sutara: I think the only thing I would add, maybe, is that this transformation isn't unique, right? The AI has been around there for a long time. I think it is the accessibility that everybody sort of saw the power of ChatGPT when it came out, and now organizations are really starting to think, what can that uncover?
You know, We talked a lot about platform and technology, but there's also the people process and platform, right? So what I tell organizations when I'm talking to them is what are those use cases that you're going to actually be able to manage the people and process change management to execute? Because we have great technology with Databricks that's going to unlock a lot of capabilities and capacity across your organization.
But how, how can we bring people along on that journey? How do we think about the change management, the behaviors, the culture, all of those other aspects that you're going to need to be able to leverage AI to its full capability within the organization? And oftentimes, those early use cases aren't the big ones that are going to make the New York Times headlines, right?
They're going to be the ones where you're solving some of your internal process, things where people look at it and say, what the heck? Like, why am I investing so much time into rationalizing these spreadsheets or synthesizing this, knowledge base, et cetera. Those are the great use cases to start with because you have more control over the data.
You can really leverage open models, which Databricks has foundationally sort of built the precipice of what we we want to make sure that you're, we're not locking your data in into a proprietary ecosystem. So what are the things that you can actually control the data, control the IP? You're not exposing yourself to the risk while we wait for legislation to continue to be decided.
So there's lots of opportunities as you look at all those use cases. It doesn't have to be the big one. That's going to suddenly make headlines across newspapers, but it could be the ones where you're really going to save your employees a lot of time. You're going to be able to deliver your services faster for your customers.
There's lots of back office internal process that you really should target. I think, and as your first early use cases because so many others have already created that you can just leverage, like Ari was talking about, these solution accelerator type of things. Where you can just implement relatively quickly, you minimize your risk and you protect your intellectual property, your IP.
Richie Cotton: I really like that idea that you make some internal tools first before you do the external tools and that way if it goes horribly wrong, no one's going to know about it. You're not going to annoy your customers until you've got the hang of things. Excellent. So you also mentioned that about people and processes need to be put in place.
In order to actually do something with this. Do you want to expand on that a bit? Like what sort of processes do you need to make use of these, this new technology?
Robin Sutara: from my perspective, I think the big thing that we tend to overlook is enablement, When you think about Executing a change around your people around creating net new processes around optimizing existing processes, people tend to overlook the change management. They sort of feel like, oh, well, communicated from the top and it'll just go So before joining Databricks, I actually spent 23 years with Microsoft where I was helping Microsoft with their digital and data transformation, and it was great that Satya Microsoft. Right, came in as the CEO and said, we will transform having your CEO say that and actually executing against that transformation to very, very different worlds.
And so you really have to be mindful and plan full around creating actual structural frameworks around the change to be able to do that. And so I find that It starts with, communication. It starts with people enablement, not just of your data teams, but also your business users.
How do you actually think about how you're going to execute against that? How do you build awareness and a desire and an understanding of why you're trying to go through that, process change or why you're trying to optimize that process? And that's why having good, solid foundations around the technology and the platform so it works when people go to execute and implement.
For example, you don't want to try to roll something out to your customers. finance team, and then they go to use the tool and it doesn't work. Right? So having those good technical platforms in place, but don't just build the platform thinking that everybody will come use it because they're all like your data team and they see what a great value it is.
You have to really think about how you're going to bring them along on that journey with you and really help them understand you're going to gain 10 more hours a week where you're not rationalizing 700 spreadsheets to come up with a single number for your business group. I'm going to save you all of this time.
And now you can deliver against all these other creative capabilities that you just haven't had time to do in the past. And so I think people have to remember that is a change and that is a change management that you have to drive across the organization and being mindful about that as an organization from the top down and the bottom up to make, that successful.
Ari, what about from your perspective? I think you've also had some of these conversations as well.
Ari Kaplan: yeah. 100%. Yeah. So change, we have like the people, the process, the platform. So the people like it all depends. A lot of what you're going to be doing is automate, but going to be automating the repetitive, time consuming boring, so to speak, parts of the job. So from the people perspective, some people like that, some people don't.
From the organization perspective, makes a ton of sense and is what Robin was alluding to. If you communicate it appropriately, people are being elevated from just being like routine type of work to more creative. Maybe they're automating the like the more simpler use cases, and then everyone gets elevated to work on the more complex or more meaningful or collaborative.
And speaking of collaborative, that's another aspect of the. After you get past the initial use cases, like what platform will help you scale, scale in terms of size, like some of these, just the LLMs, you're talking hundreds of billions, if not trillions of records. So how can you get a response, sub second, as opposed to someone asks a question and eight hours later, the response comes back.
what. Platform do you want, where it can scale, performance, have the whole Explanation transparency. So you understand and trust the data. And then, like, the other part of the people is on the positive side. How can you help enable this change throughout the organization throughout your partners?
How can this collaborative platform? Let people like Richie, you create something. Then Robin, you make your version. And then I jump in the next day and I make something new and we all just collaborate upwards together. And yeah, I have seen so many cases where, they're off the ground, they're running, they're humming, they have thousands of use cases in production and all building upon each other.
And it's incredible the innovation that we're seeing across every industry out there.
Richie Cotton: This idea of change management does seem incredibly important. I think like working in tech, you sometimes think, Oh yeah, people love tools and most people actually, they get a new tool, it's like, Oh man, I've got something new to learn. And so you do need to provide that level of support and like, Persuade them that, okay, this is going to be a good thing to use.
Okay. So on the subject of tooling, for companies who are wanting to get started, like creating new AI applications or generative AI applications. What does the tech stack look like? What are the different components of this?
Robin Sutara: Yeah, so Ari's referred to it a couple of times around the data intelligence platform at Databricks. Maybe let me give a little bit of context of what that really is for us. So foundationally built on the lake house, what we have done internal at Databricks has built an intelligence engine on top of that lake house to be able to give you that semantics that Ari was talking about earlier.
So, to understand because we talk about enablement and enabling the. Business to be able to leverage this the power of your data is only when you actually, I know people hate this phrase, but democratize it right to make it accessible to everybody across the business, your people in the business don't necessarily know SQL and Python.
And so you have to figure out how are you going to actually have a platform that allows your business users to be able to get accessibility to the data in the terminology that they want to. So what we have done with the data intelligence platform is we've actually built. An intelligence engine on top of that to help you figure out within your organization.
What are those semantics and syntax that makes sense for your business? So, for example, a Databricks, our fiscal years are February 1st through January 31st. And so if I ask a question as a business user inside of Databricks about the fiscal year, I wanted to understand that it's not. It's not a calendar year.
It's, you know, a very distinct sort of time frame by asking a question about the Europe region. I wanted to understand what countries are included in that. And so what we're super excited about is now built on the capabilities and the power of the lake house. We now have this intelligence that we have using AI built on top of our platform to be able to help it.
Business users get democratized access to the data that exists in your lake house and across the data bricks ecosystem. The other great part is that we have also integrated the capabilities for you to leverage your data bricks platform to try your own generative AI solutions and creativity. So things like giving you Databricks assistant that can help you create or identify errors in your SQL or Python code based on what you've created in a notebook.
Optimizing your workflows so that you're minimizing the amount of cost of moving data or having to read or write data out. Like there's lots and lots of things that we have actually now built into the platform, leveraging AI to help you create your generative AI. So I think when we think about tooling, we're really thinking about simplification How do we make it easy for business users to be able to get to get access around that democratization of the data?
And then how do we democratize AI by making sure that the platform can help you create your own AI solutions and products within the platform as optimized as possible to minimize costs? So we're super excited. I think what the data intelligence platform will create. Databricks did create the Lakehouse paradigm 10 years ago and sort of that foundation.
And we really think this is the next generation. This is the next sort of frontier that organizations and companies and developers are going to be thinking about.
Ari Kaplan: And to add on that, since I just was a co author and just produced a blog on how do you get started? What are the different steps of going, from that foundation that you have the data and it's all harmonized to doing your. Just do you use chat GPT, which is some open solution that may not understand the context of your business.
Like that fiscal year or what does the word churn mean? What does the word broken mean? Then you could do what's called rag, which is a term most people are getting familiar with, which is to like augment an existing. created LLM to, fine tuning your own LLM to creating your own LLM from scratch.
So, one big leap was this company Mosaic and Databricks acquired that over 1. 3 billion. But what they did is make it super easy. So you don't need to have hundreds of data scientists to duplicate. Open A. I. You can leverage a tool like mosaic M. L. Then data bricks where once you have the data to make an L.
L. M. Not just is super easy. There's a few commands you could learn, but I think importantly is it's trained on your own data. So that keeps privacy issues like you hear of companies not wanting to use it since if it's medical information or private information, if you want to control it, it won't get out into the public.
If you want to limit when people are asking questions with public solutions, like the whole world knows what's questions are being asked or what, like, documents are being fed in to, to write better. If you create your own LLM or your own Gen AI more generally you can control all of that. And that was one that has been one challenge of companies implementing their own gen A.
I. Is there just worried that the whole data privacy will get leaked to the world. So if you build it yourself and it's easy and it's much less expensive instead of millions of dollars to build, you're talking maybe thousands or tens of thousands of dollars to build. And that's just within the last year.
It's getting much easier, less expensive, more scalable. It's, it's incredible.
Richie Cotton: Excellent. So lot to unpack there. Perhaps to go back to Robin, your point about data intelligence. So, can you explain a bit how this is different to traditional business intelligence? Is it just the natural language interface on top or what do people need to know about the difference between data intelligence and business intelligence?
Robin Sutara: Yeah, I think. The business intelligence from my perspective is just reports, right? I can't tell you how many organizations I've walked into where you say, are you data driven? And they're like, of course, let me show you this beautiful dashboard. Right. And because they sort of think if they have that business intelligent and some level of reporting based on their data, Data intelligence is taking it to a whole nother level.
It's how do you actually make your data intelligent, right? How do you become more intelligent about your data? And it's not just a backward looking report. It's how do you now become predictive? How do you become preventative? How do you become more forward looking and, and Create environment in a platform that allows you to not just be reactive, but to actually be proactive based on the data and the insights that you're creating in real time and based on historical data assets.
So I think there's lots and lots of when you think about, yes, it's an integration of AI inside the platform, but it's the thing that's going to help organizations that have been stuck in. This sort of stagnant middle. I think I even saw a slide once from Databricks. It's in purgatory. You are like a data purgatory between backward looking and forward looking data.
Intelligence platform is the thing that is going to help organizations get away from just being backward looking DSO. I think that's where we're super excited is what? What is that actually now? uncover when your data is helping you be intelligent as opposed to you just leveraging humans to look at past past behaviors.
Richie Cotton: Excellent. And do you have any, like, concrete examples of how this might play out in practice? Like, when you can use this data intelligence to make some prediction or make some decision?
Robin Sutara: Yeah, I think organizations have done this manually for a long time. If I think about things like predictive maintenance of aircraft engines by Rolls Royce, I think is a great example, right? They were using huge volumes of data sets primarily trying to be backward looking and then have the human technicians trying to pull all this data together to decide when should they Preventively go change a part on an engine to expand the life cycle or save some funding now by leveraging the data intelligence platform.
They're actually able able to run AI leveraging the lake house and data breaks on top of that to be preventative to go and replace that part on the engine before. It fails and thus they're able to reduce costs by ensuring that they have the right parts at the right place at the right time and that they're minimizing the downtime.
Not to mention things like the societal impact that we talked about, the carbon footprinting, all of the different aspects that go into something of that size and scope and scale. I think organizations absolutely are going to start finding more and more. use cases that help them be able to leverage their data intelligently using a data intelligence platform to drive those types of activities forward, which ultimately, I think, will be beneficial.
Richie Cotton: It sounds like there's a few components to this then. So some of it's about automating things like having data stuck in different places and bringing it all together. And some of it's about adding semantic information about like, how is this data related to the business? And some of it's about helping you make decisions.
Is that an accurate summary of? bringing data intelligence as a whole.
Ari Kaplan: Yeah, it's like the whole end to end one end is like getting the raw data, and there's the traditional way of, what you call ETL, extract, transform, load, or ELT, depending on the case. And so there's intelligence there. How can you automatically, like, correlate and join columns on someone's name if it's Mike versus Michael, fuzzy logic.
There's intelligence to automatically document and understand your data model. So you might be a company with thousands of tables or tens of thousands or hundreds of thousands of columns. And one, one cool thing that we like to showcase is automatically document what is contained Each of the columns, what type of data, how does that interrelate?
And then, like, the workload is how do you look at data at rest versus and in addition to data you're ingesting into your cloud? How does it all work together? And then the actual models itself when I say model like a predictive model to hear terms like linear regression or gradient boosted trees or random forest like that.
That's all part of the intelligence. But even one level above that. what different types of models is called AutoML using MLflow? Which models are more accurate for like the given data and the given question you have? And then, like on the tail end, how do you have intelligent interfaces?
So semantically, you can ask, who's churning in this fiscal year? And the fiscal year could start in January, it could start in February. The word churn could have different meaning. The word sale could have one meaning if it's at the point of purchase or if it's once it reaches the 30 day return limit.
So all of that are like ways that you have the data intelligence. It's the challenge companies have is they have so much vast amount of data. And the, the phrase data is the, the oil of the company. Certainly it's major asset of most companies. And if there's data out there being untapped and general rule of thumb, 90 percent of your unstructured data is untapped, unused, you collect it.
It's sitting out there, all this effort. But it's being unused, so that's where data intelligence adds value. It taps into, like, really all of your assets so much easier than it ever was possible before. Since you don't have to manually write code for everything, the intelligence finds your assets and knows what to do with it.
Yeah.
Richie Cotton: That sounds incredibly useful. So one thing you mentioned there was like that churn might not mean the same thing everywhere. And I think this is a very common business problem is like every team within a company define things slightly differently and suddenly you've got seven different definitions of churn or marketing attribution or customer lifetime value or something like that.
So how does this data intelligence help you deal with this sort of thing?
Ari Kaplan: there's, stuff, data, intelligence, specifically, could either intelligently try to find it out for you or like any gen AI, you can have humans guide it along the process. So you can have like humans rate the result, positive, negative. And over time, has like And what you might call reinforcement learning.
So it gets smarter and smarter the more people use it. and the more value you get, it falls short. So it learns along the way. Then above and beyond, we do have, plenty of partners out there that also focus on the semantic layer in pretty powerful and unique ways. And, through partners that integrate with the platform and what we do internally in the platform.
Are, great harmonizing benefits.
Richie Cotton: Fantastic. I'd like to go back to something you were talking about earlier, which was the data and security issues. So, I think this is a very old fear, is that a lot of companies are like, well, if I send my data to another company, particularly like customer data or financial data, then something terrible is going to happen.
Can you tell me, like, how real are these fears?
Robin Sutara: It depends on, how have you thought about the risk and compliance around your data? I think we are seeing examples, for example, the recent New York Times case against open AI, right, for sort of taking their information sort of not giving attribution. I think that's a very real risk that organizations have to think about when you put your information into a ecosystem that you don't control.
I think this is why foundationally Databricks is such a great platform in that we do know that some proprietary models are going to be the best ones for your use case. But what is that data that you're putting out there? It can't be your trade secrets, like what Samsung put into ChatGPT when it first came out, right?
It can't be your customer data where you're going to run the risk of GDPR and being unable to comply because you put that information into a system that you don't control. That's why you need a platform that can support those proprietary ecosystems on the use case where that is the right model, where it's most cost effective and efficient to use those.
But you also need a platform that can support creating sort of your own models, your own based on open source technologies, et cetera, within your own environment and ecosystem where you could create sort of that Chinese wall that this is the data, our trade secrets, our customer data, our financial data, where it would be super detrimental to the company if that information were to get out.
Those are the things that we want to make sure that we're doing within our own platform where we have full control from left to right of those data assets. And so making sure that you're looking at the use cases, looking, this is why you have to have those foundations in place, right? Looking at the use cases that you want to deliver, understand what's the data necessary to be able to deliver against that generative AI solution.
And then, making sure that you're using the right tool within the platform. for him to be able to deliver against that. And that's why we're super excited. I think about data breaks and that it is that it gives you the capability to do both of those things, We're not telling you that you have to lock all of your data into a single proprietary ecosystem that one model is going to control everything. We do think that as fast as models are being created, as Ari mentioned earlier, right? The last year we've seen a phenomenal pace of innovation. I think we'll continue to see that going forward.
And so there will be new models and new capabilities that continue to come out that will be more efficient or effective for your organization to use that in specific use cases. And so making sure the platform is open as possible to be able to support all of those because you have to think about.
The risk and the right and not, restrict your pace of innovation for fear of releasing anything, but making sure that you're being very cognizant of releasing the right data into the right system and models to be able to deliver that business value. So, sorry, that's a, that's a dependent answer, but hopefully it gives a framework of how you should think about it.
Richie Cotton: Yeah, so, I certainly hear your point about you need to have control over which bits of data are being put where and what you're doing with them. And that's, that seems like a pretty essential thing when you get to high value data, like customer data, financial data, things like that. related to that do you have any advice on like, what companies need to do to improve this sort of data management then in order to It seems like this is important to get started, like before you even start thinking about building the AI, you need to get your data management right.
So what advice do you have for improving data governance in general?
Ari Kaplan: Yeah, so one challenge with data governance is if you have an environment where you have one tool for your data warehouse, another tool for your data store, another tool for your, data lake another tool for visualization before long, you have the sprawl of all different tools. And especially around governance, you'll have, let's just say you have five vendors, you're going to have five audit logs, you're going to have five different like username passwords.
Most of the time you're going to have like what we call lineage, like where the data Comes from how it's joined, how it's processed, what the models are based on, and that's going to be a problem for not just governance in that case, but for, like, even understanding when data is drifting, the world changes when do you need to redo your models?
that's just operationalizing, but from governance, that's why companies Want to standardize on a few platforms as possible. Hopefully, just one. Of course, we recommend Databricks. We have this pretty sensational part of that called Unity Catalog, where, you could, monitor everything.
You can, automate, scheduling. But one of the things I love is like that whole lineage. You get A viewpoint over who what when is accessing and also you get a viewpoint into have all these data assets who's not accessing not just the data But what models are people running when and by whom?
If it's internal people, but also now as we were talking about there's like this whole marketplace where You can create a model and then your customer could be running it. You could have access by a website like who are running new predictions on existing models who are creating new models. When was the data made?
Who has access to it? And that's why you really need that unified Governance solution out there and Robin, anything you'd want to add there?
Robin Sutara: Yeah. No, I think we referred to it earlier, right? when we think about how do you create the single view of your governance across your organization? I think Unity Catalog is definitely where we're making all of our investments to become foundational for organizations to be able to help that even things like Federation so that you could access data assets that are outside of the lake house because we know not every organization is going to be homogeneous.
Yes. on a single platform. And so I think it's definitely something that's been on our radar for quite a while and thinking about that. And when I think about things like intellectual property protection, that organizations are thinking about really understanding where those data assets exist, what data is going into what system, what model has leveraged ethics and transparency.
I think with the EU AI Act, the President Biden's executive order. We're just seeing more and more of this moving very, very quickly. I think there was even a ninth circuit decision a few weeks ago around, marketing content that was created via generative AI was not subject to copyright protection.
So I think there's lots and lots of things where organizations are really going to care about what is the data that's gone into the model? What's it created? Where is it being fed into? could we say exactly the algorithms that we use to be able to get from X to Y?
And I think organizations are going to have to really think about that. Going into the future. And so making sure that they have something that's a single governance solution that they could easily get access to that insight in that information to be able to provide it, I think is going to become critical.
Richie Cotton: seem like there's a lot of legal action coming very soon, but it's hard to predict what you need to do until those laws have come out. But I take your point that some of these sort of copyright issues and IP issues are going to be very important. So for the more conservative organizations who are risk adverse, what do you need to do that to make sure that you're protected against potential problems in the future?
Robin Sutara: Well, if I knew that I would be a billionaire, I think. Right. But I I think I think what we talked about earlier, right? Really thinking about for these early use cases while you continue to wait and see, make sure that you're balancing that risk that you're willing to take versus the pace of innovation that you want to deliver against.
So the more things that you can think about where you're leveraging, a platform like Databricks, where it's as open as possible that you could leverage proprietary models where it makes sense that you could leverage open models inside your organization on on your data without exposing yourself.
I think that's going to become critical to think about those early use cases where you're thinking about internal sort of even external facing to your customer, but where you're trying to control those data assets and minimizing your footprint of risk by exposing your data assets outside of your organization, I think is going to be critical in the short term while we continue to wait and see what legislation comes up.
Ari Kaplan: Exactly. And this concept of clean rooms is something that's when you start sharing data and I. P. and code externally, you know that that's where like it obfuscates things like social security or HIPAA or internationally regulated data itself. So that's going to be more and more important as time goes on,
Richie Cotton: So can you give you a more controlled way of sharing either customer data or other sensitive data with other organizations then?
Ari Kaplan: exactly without sharing the like specific portions of the data that you want to protect or sharing it if everyone has like an NDA, legal agreement, but you get that fine grained access control at the row level, at the column level Things like that at the table level.
Richie Cotton: Excellent. So I'd like to take a bit of a sidestep here and talk about jobs because it seems like with all these changes in the last year, particularly around AI, that many data roles are changing. So can you talk about like, how you've seen data roles changing recently?
Ari Kaplan: was just moderating this amazing panel on this subject with like the head of AI for Meta and some other cool folks. And this is the big question. My own, three children, they're like, Hey, if I want to go into programming, should I learn Python? Should I learn R? And it's like maybe a year or two from now might be predictable, but four years, it's going to be hard.
So, the value will be people who understand. The concepts like what is data? How does data relate to the real world? Business logic, but then foundations of math and data science. So I had probability and statistics. I think those things are still you're going to need to understand the context.
So if you have a choice of 10 different models, you're going to want to understand what's going on in the creation of the model. But from a math probability standpoint how does that relate? And then understanding the business is really important. Like a couple examples I like to give one is I worked at a retailer at a consumer product who had a consumer product.
Company had a partnership with the retailer and the AI system said, just don't sell in Walmart. And that was the optimal strategy. And then the business said, wait a minute. We have a five year contract. We can't break that contract. So understanding that you should relate and put that into the model.
That's the role and the job of people who know that. And then the other example was giving a general manager and a major league baseball team recommendation to sign a picture, but that picture was injured and wouldn't be, playing the entire next year. And the data scientist said, Oh, I had no idea that, there's a data source of injuries, just, didn't understand. And if you sign that player, it wouldn't have been good. But, it just goes to the point that you want data scientists or math people to understand the business along with that. That being said, a lot of jobs are going to change. A lot of programming is going to be automated already is being automated.
So, yeah, everyone's going to have to level up. It'll be good to understand, you know, the basics of SQL and Python and some other languages. So you'll be able to get a concept, but just doing your basic, select star from a table, that type of stuff will be automated. So you'll need that as a stepping stone on the way to being able to do more and more complex information.
so, yeah, it's going to be fascinating. Three years from now, where, where this all leads, where you're going to have CodeGen built on CodeGen, yeah. Yeah,
Richie Cotton: on top of code gen, like it's just AI all the way down. I like it. And I have to say that example with a data scientist not knowing about the injury table, I suppose that shows that, yeah, you need to have your data in a place where all the, all the data professionals can find the data.
So related to that what sort of jobs what sort of roles are using Databricks? Is it data scientists or are there roles involved as well?
Robin Sutara: part about our platform is we're actually created so that all those personas are using one platform, right? They're not all using separate tools and you're not trying to copy data from one system tool to the other. So everything from your data engineers to your data analysts to your data scientists.
To your business users should all be able to use Databricks platform so that you have the same capabilities that you have your single source of truth. Everybody's using the same data sets, etc. I did want to just add maybe one comment to Ari's like absolutely agree that people need to understand the foundation, particularly early on our A.
I journey, right? I, it's interesting to me that Engineers are leveraging things like assistants and co pilots, etc. to be able to generate code. But there are times where you need a person to look at that code and say, Hey, wait a minute, that's not quite what I thought it was going to do. And so that's why understanding those foundations are going to be critical, think, for this interim period over the next four or five years.
Because you still need human intervention. People are not going to be displaced by AI. They're right. AI is just going to augment and make you more efficient and capable to deliver faster. And the other thing is, I think we need more people with creativity, which is a great way for us to introduce diversity into these roles, People with different backgrounds, with business backgrounds, with finance backgrounds. Are now going to become data people because I now exposes the technology to people who in the past would have never viewed themselves as technologists. But your programming language now becomes natural language.
So everybody is going to become a data person. And so really figuring out, I think, over the next several years on, what is that value benefit that you bring in your design background in your sort of different non traditional data science. And so I'm super excited because I think That traditional approach of just thinking of STEM think is gonna go away.
We're gonna now see people from foreign languages, people from, arts and all sorts of sciences, not just computer science now being able to really unlock the power of data across an organization. And so it's super exciting times. I think for anybody thinking about wanting to get into the data field, it's a great time to start.
Richie Cotton: That's a wonderful vision, the idea that yeah, data really is for everyone and it should be made for everyone. So, just related to that is there one thing that you think everyone needs to know in order to be considered data literate or AI literate? What should everyone know about data and AI?
Ari Kaplan: do love the phrase Robin used, democratization, that on one hand, yeah, having a PhD in computer science is great and gets you a lot of places, but you don't need all of that to get started to get benefits from it. one thing that like excited me is spent many decades just trying to convince people, just take a look at what you could do.
And now it's almost flipped on the head where people are running, saying, what are the things we could do? Or I've used this, how can I use that? So, that's one thing that think it excites me the most.
Richie Cotton: Absolutely. And yeah Robin, what's exciting you the most in the world of data and AI?
Robin Sutara: Yeah, I think I would tell people be inquisitive, right? Like we just don't know what we don't know, and things are moving so fast and translating so fast. So I, think as you think about everybody will ultimately become a data person, ask the questions, be inquisitive, learn the technologies, understand the platforms.
We really want Databricks. to be accessible to everyone. Download it and play with it. I think we'll share some links with you. Like, just get your hands in there and get dirty. Play with things that are gonna sort of help you understand where we're going. Because I think everybody across the ecosystem gets help define that direction.
And the more we get engaged and the more we get involved, and the more we leverage the technology and the platform, I just think there's nothing but greatness for for not just corporations and organizations to monetize and generate a profit, which we all like to see, but I think the societal impact of the things that data can unlock and empower, and things like, creating environmental impact Personal and professional health, et cetera.
I just think the power of data is limitless. And so be inquisitive, play with the technology. Only you have sort of the brainpower and the knowledge about your area of expertise and that data and that space. And it's only by you doing that thing, those things and getting involved that you're going to be able to help us solve the next generation of problems.
Richie Cotton: I love that. Yeah, so you can use data to make some money, but saving the world as well, having some societal impact is also pretty awesome. Excellent. Ari do you have any final advice for organizations wanting to improve their data capabilities?
Ari Kaplan: well, I'm not a salesperson, but yeah, Databricks, having that end to end platform so, so, important and the challenges along the way are, how do you get started when you don't have skills that that's. One way we help, how do you do things cost effectively? You know, Robin had mentioned, you don't have to move data around.
If you have five vendors, you may have to shift data around five times, like for everything there's cost involved in terms of money, in terms of time. So. We see customers like dropping their price. You vary, 10%, 20%, 80%, 90%, you need to scale up. You have a chat bot, for example, and you want the response to be sub-second versus five hours.
You need a performative platform. Everything based on Apache Spark and and that compute platform, you really need that as your data is growing and a lot of companies, their data grows doubles every year, every couple years. And if you don't have that scalable infrastructure, you're going to Hit a wall at some point, a dollar wall, performance wall, and so on.
And then I guess the last thing is like, we just did this world tour of all dozens of cities. And the theme was generation AI. And what's inspirational to me is that everyone listening, we're all defining what the next generation of Gen AI will be. And so we all have a say in we were talking about the themes of, ethics, of governance, of what the potential could be the positives, the negatives.
What do we as a society and as humanity want to take it? So that's inspirational, is that whole generation AI theme.
Richie Cotton: That's fantastic. I like the idea of Gen AI. It's like, I guess, whatever comes after Gen Z.
Ari Kaplan: Yeah.
Richie Cotton: Brilliant. Okay. And Robin, do you have any final advice?
Robin Sutara: No, like I said, I think it is just be inquisitive. Like I already said, it is generation AI and we're defining what that generation looks like. So, get engaged, give your feedback, make a difference, impact and influence. It's such a great, phenomenal time, I think, to be in the data space and super excited to be on that journey with the two of you.
Richie Cotton: Wonderful. Excellent. Thank you so much for your time. It's been really informative show.
Ari Kaplan: Yeah, thanks Richie. Thanks Robin. Thanks for everyone listening. Appreciate it.
podcast
[AI and the Modern Data Stack] Adding AI to the Data Warehouse with Sridhar Ramaswamy, CEO at Snowflake
podcast
[AI and the Modern Data Stack] Accelerating AI Workflows with Nuri Cankaya, VP of AI Marketing & La Tiffaney Santucci, AI Marketing Director at Intel
podcast
Scaling Enterprise Analytics with Libby Duane Adams, Chief Advocacy Officer and Co-Founder of Alteryx
podcast
Data & AI Trends in 2024, with Tom Tunguz, General Partner at Theory Ventures
podcast
Data Trends & Predictions 2024 with DataCamp's CEO & COO, Jo Cornelissen & Martijn Theuwissen
tutorial