How this Accenture CDO is Navigating the AI Revolution
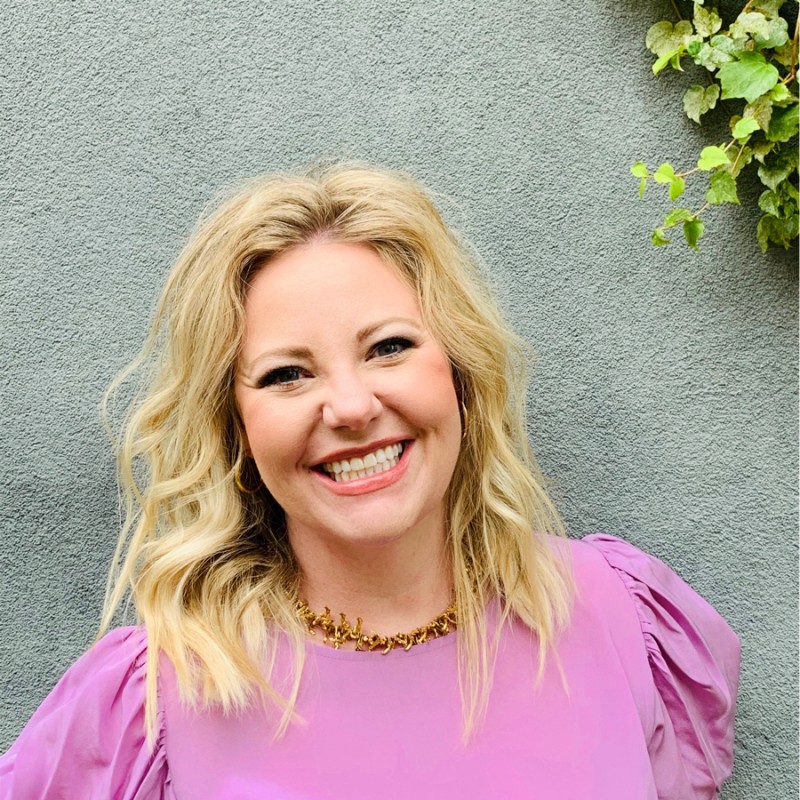
Tracy Ring leads Accenture’s Applied Intelligence Products Category Group, in this role she has leadership across Consumer and Industrial Products, Automotive, Life Sciences, Retail and Aerospace and Defense. As the CDO and Global Generative AI lead for Life Sciences, she personally anchors the NA Applied Intelligence Life Sciences practice of more than 500 practitioners. Tracy has created solutions for Generative AI, Data led transformation, Artificial Intelligence, Data and Cloud Modernization, Analytics, and the organization and operating model strategies for next-generation adoption and AI fluency.
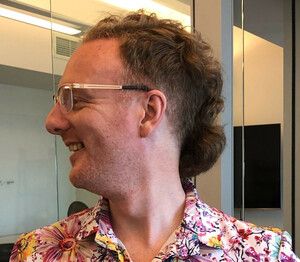
Richie helps individuals and organizations get better at using data and AI. He's been a data scientist since before it was called data science, and has written two books and created many DataCamp courses on the subject. He is a host of the DataFramed podcast, and runs DataCamp's webinar program.
Key Quotes
I have a very basic rubric of when to use generative AI. The first question one must ask themselves is, do you care if the answer is correct? And if the answer is no, then it's yes, go ahead and use generative AI. And then the follow on question, if you care that it's yes, then the question is, are you uniquely qualified to confirm the results and to test them? And if the answer is no there, then you should not. AI makes mistakes, humans make mistakes. This is, I think the perfect balance of getting to the point where humans can focus on the things that are most important. Humans are not correcting the things that are fatiguing or mundane or commoditized. We know that AI is gonna get smarter the more that we use it, the better that we train these models. And that's really core to the genesis of all this.
One of my favorite people to read and listen to is Brené Brown. She talks about how within her team, when there's a big decision to be made, she realized what was happening was that she would answer the question first and then everybody would follow suit. Right now, when there's a big decision to be made, she writes down her answer. She turns it over and everybody else has to go first. And it doesn't matter that, you know, she might have a certain opinion, but she realizes that she was biasing her whole team and they were following the leader, so to speak. We all can be watchful of how we're introducing bias and catching ourselves and really thinking about new ways of working. We've spent the last several years working in new ways. But, for the most part, we're back in front of our clients. We're back inside workplaces. It's an opportunity to think about how we shape and grow and cultivate the conversation. And for me, it's always about, are we having the right conversation or is there the opportunity to talk, to have the conversation or ask a question. Infinite curiosity is something that I always try to instill in all my teams.
Key Takeaways
Embrace your data like a product, with continuous updates and improvements. This mindset helps to avoid the trap of seeking perfection before releasing a solution, which often leads to low user adoption.
Try to foster an environment of infinite curiosity within teams. Cultivate conversations, ask questions, and continually learn. This approach can lead to more innovative solutions and a more engaged team.
Balance your AI and human inputs, try to keep a targeted approach to implementing AI while addressing the technological debt your team may have.
Transcript
Richie Cotton: Welcome to Data Framed. This is Richie. With the current pace of technological change, keeping your organization's data practices up to date is demanding. At the best of times in the life sciences sector, whether you're in pharmaceuticals or biotech or healthcare, there are additional challenges due to regulatory and privacy requirements.
The explosion of AI in the last year has made everything even more complicated. Our guest today is Tracy Ring, the Life Sciences Chief Data and Analytics Officer, and global Generative AI lead at Accenture. In this role, Tracy manages a group of over 500 data and AI practitioners in life sciences. And works harm data modernization and data transformation programs, as well as AI adoption programs.
She's got more than 20 years experience advising clients on how to innovate with technology. In short, she's just the right person to help us thread the path to success here. I'm really looking forward to hearing her advice on the subject.
Tracy Ring: Great to be with you, Richie. Thank you.
Richie Cotton: Brilliant. And let's start talking about data and modernization. So, you've led both data modernization programs and also data transformation programs. can you tell me what the difference is?
Tracy Ring: I think A lot of times they're used interchangeably, and I think even myself, I make the mistake and use them interchangeably. But the truth is that not every modernization is a transformation. Sometimes it's really only about moderni... See more
And so, while a slight nuance I see that they have vastly different results, vastly different trajectories Typically vastly different funding as well. And so, given my druthers, I would always choose to be a part of a data transformation. And encourage anyone that's embarking on a modernization journey to think more holistically.
Richie Cotton: So it seems like modernization is really a sort of simpler, like a subset almost of data transformation.
Tracy Ring: Yeah, absolutely.
Richie Cotton: to make it concrete, can you give me examples of each?
Tracy Ring: Yeah. I'll take it one step further to say that, you can do modernization and not do transformation. I also believe that you could do transformation without modernization, right? And so modernization would be in, in my mind that I have a legacy data store set of data marts or data lakes that I'm either migrating to the cloud or migrating to a modern C d p transformation would be thinking about data in a fundamentally different way, working with business stakeholders to change the way that data's embraced.
And transformation, in my opinion, would always come with some form. Of data literacy or as I like to call data fluency because no one likes to be data illiterate, but you can certainly be data influence. So I like to, really think about the idea of educating everyone. And this is, even frontline individuals that maybe only are doing data entry, right?
Here's the impact of you putting things in a field that is unconstrained. The number of organizations. I do a data management program with that, that we start out by saying, we wanna do some data quality and have. Heated discussions about how great a quality their data is only to have to prove many times that it's not what's so interesting.
And so when I think about that broader picture around transformation, I think it's about setting The picture around here's data as one of your most valuable assets of an organization. Here's how you can use it and here's how it can propel you to the future. Oh, and by the way, there's this technological backbone that is gonna be a key enabler for that.
But you know, the truth is even if your organization was, was run on spreadsheets, you still could have a data transformation as, as much as I, I think I'm probably making some of my. My colleagues cringe, that this is really about the spirit and way that it's embraced, that it's utilized.
The decision making is underpinned by data and analytics and those two things hopefully go on concert. But I think, I'm a big fan of however we can get more people leveraging data for their strategic advantage.
Richie Cotton: And I like that you mentioned data literacy there, so we're big fans of data literacy. See on this show. But it sounds like, for a data transformation program, you can have a lot of different teams involved. Can you just give me an overview of like what the scope is, like which teams, which roles have to get involved in data
transformation?
Tracy Ring: so I think it goes without saying and I always anchor on this, is that who's the ultimate business consumer? so, the business end users that are building the use cases and are ultimately gonna be. Using the insights that are provided by the data are part and parcel to this.
And I think a lot of times, I personally have even made the mistake earlier on in my career of saying, you know, well let us do all this plumbing work and then engage them later. Right? Like, let me clean up all this and I think that's a mistake. So, so I think it's about business engagement from the very beginning, having a that type of a perspective.
it's no surprise, source systems and feeder systems need to be involved in this journey, right? Because to avoid the unending data cleansing crisis that many of us find ourselves grappling with we have to solve the input side of this equation.
And then I think it's about, thinking more holistically about what does data look like? And. And I always talk a lot about data as a product, right? Like how do you think about continuing to build out on data? Just as if you were releasing a product or doing point releases onto a piece of Software or an application.
And the more that you can get into that product mindset, you get out of the mindset that it has to be perfect and, go away in the back room for nine months and then come back out and whizbang there's a solution that, that probably doesn't have great user adoption. And so, the other piece of this is that on the user adoption side, I think that, focus, change management focus learning and education and that, and again, training in education is not a one time effort.
If you're treating your data like a product and there's always something new coming, right? There's always a new release, then there's always something to educate upon. And then I'm not saying that it needs to always be a training class or a certification but I think the spirit of treating data like a product is really around this concept of communicating, sharing what's there.
I was recently with an organization that said, Hey, we have this backlog of data work that needs to be done. And I said, okay, that's interesting. And in, in the backlog was two years old. And I said, well, there can't possibly be a backlog that's two years old. And they said, well, more or less we still we have to work out all this stuff and it's not been done.
And I said how are your business users okay that, in two years how that. That's probably not just in time by any means. and they said, yeah, we just have so much work to do, et cetera. And we looked at it and ran some analytics on it. 30% of their pipeline was duplicative.
10% of the pipeline of backlog was already available, and they didn't know. And then the remainder of it by some amount of massaging it together, most of it, you could be solving, two for one, right? And so we took this two year long backlog and shrunk it down and said, let's catch up in four months and then never create this problem ever again.
And here's how you create the literacy, create the transparency, create the communications and the last pillar of this is around adoption, The number of times I come in and you'll see organizations that have more than one dashboard for every employee worldwide. That's incons consumable, right?
They're not only consuming a ton of technical debt, but most likely are not using all of that. And, And I think that goes into a lot of it you're not using it, then then you need to deprecate that technology and not drag that forward. And I think that's That continuous process, while I talk about it a lot is like the in real time of a program, it's a lot of times how we start and say, okay, you may think that you need to modernize. But actually, enormous portion of this is never used. And that can be something from entire data marts to a field that's, so sparsely attributed that it's not valuable to carry forward.
And so I think that spirit of keeping our technological and data house very tidy is incredibly important.
Richie Cotton: Find a lot of people are starting to talk about realtime analytics and how you get answers in microsecond and things. I'm thinking like two years is like very much on the other end of the
Tracy Ring: Yes, indeed.
Richie Cotton: that does sound like it was a bit of a horrendous situation and I'd love to get into some of the details about like, What the solutions you proposed.
Were in a moment, but before that, just in general, cuz you work a lot with the life sciences industry, can you gimme some examples of how data transformation programs work in life sciences? Like what are the goals?
Tracy Ring: Yeah, I mean, I like to think of the idea of having a North Star around the program and what are we anchoring towards and how do we paint that mission and vision is a way to to really be there to guide the journey, right? even in the most agile or real-time analytics perspectives, these can be a little bit of a marathon.
And so having that North Star to guide and say, is this in? Scope Is this not, is this something that, that really is behind why we're embarking on this transformation? As a key input filter is of material importance and. I actually find that I'm so fortunate to work in an industry that, we're thinking about how to bring drugs to market faster.
We're thinking about personalized medicine to cure cancer in a way that's never been done in the past. Bringing drugs into clinical trials and short-cutting. What we typically see is, a multi-billion dollar pipeline Anchoring on, we're here to bring something that I think is greater for humanity is always an exceptional place to start.
And then breaking that down into sort of what are those key pillars to bring forward and that's where I encourage organizations to really say, what is most important? In some cases, agility is the most important thing. And to your point of real-time analytics, if I get you to, 98% of the data, it might be, significant enough to make a decision on commercial.
If we're talking about clinical trials, then we're gonna move into, a hundred percent right? Like we have to make those types of decisions. And I think the intentionality by which you embrace that into your organization is of critical importance.
Richie Cotton: Absolutely. It does seem like the stakes are quite high in life sciences. Like you don't wanna be saying, oh, sorry, we didn't. Your answer this year because the data pipeline was broken. So, some of the things you talked about were fairly general cross industries, so things like defining a North Star metric and trying to align your goals around that.
Are there any challenges or requirements that are specific to life sciences?
Tracy Ring: I think that they are, but I'll be honest, at the stage that we are at, in the industry and I do wear a broader hat around broader products, so consumer products and retail and I have a leadership role around that. I think we're at a place where responsible AI and data sharing and data privacy and security are at a point that I would say that those North stars are more similar than dissimilar.
Right. The actual business use case is Probably different than if we're thinking about banking and life sciences. But the truth is, I think the the burden and in, in the opportunity that we each have in this space has to be anchored on the same. And so, it's not phenomenally different and I actually see so much.
Particularly in life sciences of recent years that, we're seeing so much around looking at outside the industry to learn new right? It's by example. I don't think life science is historically based on the way that they serve patients, right? It's a three-tier market. You're going to, your doctor, your doctor is, prescribing, right?
But you've seen this pivot, right? We have direct to consumer marketing for pharmaceuticals, right? And so, so this idea around customer experience and taking a page away from. What we would traditionally think of a consumer product or things like that. They're more similar.
I think the more that we get into the digital world patients are smarter. Patients are going into a doctor and specifically asking for this prescription that saw a commercial on the Super Bowl about, right? So, so we're seeing that most certainly I think we have smarter.
A more tuned in customer space and the industry has pivoted to that.
Richie Cotton: That's fascinating. The idea that people who are just wanting medicine, they have more education now. But I wanna pick up on something you said but you were using ai and so this is obviously a huge topic at the moment, just a little bit. And so, are there any particular sort of uses of artificial intelligence that are particular to life science?
Tracy Ring: Yeah. I mean let's be honest, the most exciting time I think we've had in our careers as it relates to data and ai. And I don't decouple them. I think of them as inextricably linked. But, whether you're thinking about using AI to streamline your product pipeline, the way that we create.
Drugs is really, just like anything else, a huge filter of things that could be potential candidates. And you continue to iterate on those compounds in a way that you can bring them to market and bring them into clinical trials, right? So the more that you can shrink that funnel and the more that you can make that funnel go faster.
So model informed drug discovery is at the heart of that. And then again, that, on average a decade in, in multi-billions of dollars to bring a drug to market. Every day and week and month, you can carve out of that. And every time that you don't pursue something that's ultimately gonna drop out and not move into clinical trials is better, even better.
Anything that I drop out early when I'm trying to solve for a certain therapeutic area but I find indications that could be valuable for something else. Right. So it's everything from dropping out of what you're looking for. But also to maintain that data and and be able to use it forward.
That's the early stages. The other piece is thinking about clinical trials. It, it might not be intuitive, but one of the biggest hurdles that we have is that we have a set of individuals that are brought into a clinical trial. And if everybody doesn't finish the trial, then they have to start the trial again.
Or they have to go through special, steps to make sure. And so clinical trial adherence is absolutely imperative. And so when you think about new ways to communicate and new ways to nudge people, right? I think we've also gotten so used to, whether it's haptics on our watch or, or predictions.
I, I just got an email from my bank two days ago and it said, Tracy, Your bank has new hours, click here to go figure out what the hours are. And I had this moment, I thought, well, gosh, they know which bank I always go. They always, they, why don't they just say, Tracy really only goes to this one bank, right.
Let's tell her the hours of the one that she actually cares about. And if she's looking somewhere else, we can send her to go look at it. And I found myself going, wow. Like I am expecting a curated experience for myself. We are. Becoming self-trained to expect everything to get smarter for us, right?
And so, things like how do you use weather patterns to tell someone, Hey, by the way, you're supposed to go in on Friday for your clinical trial check in, it looks like there's gonna be horrible weather. Why don't you reschedule it now so that you're still on the right cadence to be compliant with the trial?
so thinking about how you can constantly get two steps in front of every. Client and, clinical trial patients would be clients. Right. How do you two steps ahead of that? Because we are all ex expecting that because it's threaded into every single thing that we do.
And the other one that I'm quite fascinated about is marketing and materials consistency, compliant with the F D A or whatever regulatory agency you're a part of One of, well, the biggest things that is material, is that, that it has to be absolutely consistent.
You have field sales organizations that need to all have the right messaging, right? But how do you curate the messaging for a certain type of doctor that you're trying to get time with? While still being compliant, right? Because our doctors are asking us to talk to them and sell to them in new ways. They're not used to having to do, traditional, let me hand you a piece of paper about, why this medicine has higher efficacy or higher safety, right?
So, so, I believe, particularly as it relates to generative ai, that we see some content area that we can be more compliant, more curated. And in the last bit about AI that I think is so fascinating is it's no surprise that regardless of where you are, we are still in a talent crunch.
And talent crunch means that people are constantly looking to have the most fulfilling roles they're looking for roles that they find to be interesting and compelling. And yet we still hear that, many individuals are doing what we would consider repetitive, non-interesting. Tasks, it's hard to retain people and it's also difficult to keep them engaged because when you're bored, you make more mistakes.
And so I'm absolutely super obsessed with this concept A around. How do you use things like generative AI to take out, let's call it 80% of the things that are not interesting or don't require human intervention, and then have that, let's call it the 20th percentile, work on the things that you really need, a human involved, that you need a human to, to to validate and so, those are, just a few examples.
I, probably would tell you that I have. Over a hundred use cases that are in my, library of things that we talk about. in every day we're learning more, right? I the use case that I gave you around marketing compliance is something that, that we're applying over to regulatory filings, which is very painful very error prone, et cetera.
And so each and every one of these it's about how do you take what you've learned and continue to apply it to other areas that could be beneficial. we're learning new cases. And, And I also think our regulators are learning new ways that they'll accept AI enabled capabilities, right?
And so that responsible lens really never goes away. But we're learning a and and being judicious as we move forward.
Richie Cotton: So many great examples there. It sounded like a lot of these examples involve many different systems come together. So you gave the example of like, a weather system feeding into an appointment app. So, once you start doing with all these different systems, how do you manage that?
How do you make sure that everything sort of gels together cohesively?
Tracy Ring: and this is the part that I don't think is all that different. This is still about thinking about how do you take. That fine grain data and combine it with data that is, either third party external maybe it's harnessed internal.
but I think it's really about that, sort of instrumentation of how you think about your data journey. What data can be a combined what data cannot, what data should not. Again, questions as it relates to responsible AI are coming up and I think this is where we can really never let our guard down and we are in uncharted territories for so many areas and I think about the journey of sort of humans plus, right?
And how do we, think about incorporating that in a responsible way, which means that there's always a human angle into it. Just because we might have done something away, a few months ago, doesn't mean we don't need to get smarter and smarter. Right. Because particularly as you're thinking about like weather data that's changing, right.
Whether you're pulling it through Google or other sources, right? Those are consistently getting smarter and smarter on us, and so, I think there's all of the focus around data engineering, which I just absolutely love is a terminology right? This idea that we would model data or, do things like that.
I love this concept around data engineering, and I think it plays very nicely into that idea that I talked about of DDA as a product.
Richie Cotton: Absolutely. And do you think that your processes around data need to change if you're using ai?
Tracy Ring: This is a trick question, right, Richie? Yeah I mean, All of our processes need to be revisited. Right. We are in uncharted waters. And again, the rules of six months ago, let alone the years of five years ago, are no longer applicable. Right. we're working in new ways.
Right. Data DevOps is the standard. We're not. Doing traditional release schedules like we used to in the past. And so I think a entire review of that is absolutely material. Now, do most of my clients have the budget or the interest to embark on a process reinvention?
No. And a lot of times I give my comparisons as it relates to remodeling or building a house, right? No, nobody's all that interested. If you only work behind the walls on the electricity or the plumbing, right? So we've gotta give some, some nice new light fixtures and countertops while we're at this.
So, so doing that. And there's no better way to learn other than through a project or a program. Right. And so that idea that we're implementing new processes and procedures while we're bringing new value to the table, for me is a huge best practice. And I just wrapped up a project yesterday and when we finished a post-mortem on it and we all thought of new ways that we wish, we learned a few things, along the way and that.
process in and of itself of just saying, how do we do this better, faster, smarter next time is absolutely material. so a resounding yes.
Richie Cotton: So I feel like every C-suite in every company is going, we need generative AI everywhere now. But the example you gave right at the start about this company where like they have this sort of two year backlog on like. Fixing anything in their data pipeline, everything's a mess.
Should they be prioritizing AI generative AI because it's so hot? Or should they be fixing what they have already? Or is it just like, okay, give up on that, let's build something from scratch,
Tracy Ring: just close the doors. Forget about it. Yeah. It's I would say that, let's say 10 years ago when visualization was, we moved away from these pixel perfect reports, right? And we got into, every I think Tableau used to always say that their number one entree into an organization was via an intern, right?
And so the intern went with this dashboard over things, and then, oh, lo and behold, the data is. misshapen and needs significant remediation. AI is no different. And it will shine a light on your technological debt in a way and at a speed that we've never seen before.
So I think you're asking when and how do I embark, right? And the truth is that I don't know that anyone has the sort of silver bullet on here's how you look at generative AI end-to-end. And I see most of my clients saying, let's find a targeted use case.
Get some traction with us. And so, no sort of magic on this idea of, pilot innovation and then scale. But I think at the same time taking that generative AI journey and as I mentioned, do you need to clean up the house? At the same time, because you can't possibly proceed with everything that is not tidied up.
One of the things I'm really work a lot and in life science as we speak a lot about this idea of a digital core. And I had this debate with one of my colleagues and they said, digital core is such a fallacy. It's not about this core of digital in the center. It's about this.
Digital fabric so that it's everywhere and then it's in and everything we do. and it was interesting debate about semantics, but the truth is that. Life sciences companies in general are thinking about digital core from a way that drives agility, right? We were forced to do some remarkable things during covid particularly in life sciences, right?
And I think about, okay we all have a little bit of a breather. Are we gonna go back and say, okay, we're gonna go back to normal pace now. Well, this is the new normal. I think what we are seeing is this pivot into that digital core, digital fabric or whatever you'd like to call it, in a way that you can be more agile and that you have the backbone in place so that your data backbone, your architectural background your people backbone, all of these aspects so that when the next big thing comes up, you can react and you can react Astra more accurately.
Richie Cotton: Fantastic. And so, talking about the targeted use cases have you got examples of what people are building with AI at the moment then?
Tracy Ring: Yeah. So a big one that we're seeing again we think about and I think about this in some ways as like the next generation of automation, right? so taking through, I talked about content management, so writing, proofing. Duplicating instantiating into multi-language, right.
That's a big area as it relates to content. I think about a lot of the use cases as it relates to regulatory filings, right? A very repetitive task of submitting and creating content. And the other ones that I think are most important are really thinking through ways that you can, sink two steps ahead of your cut.
Just like my banking example, right? Like how do I educate and drive compliance for life sciences and pharma? We think a lot about, one step is to get somebody to proceed on a treatment journey. The other is to keep them on that journey, right? Keeping somebody compliant with their medication is not so different than keeping somebody engaged in clinical trials.
Right? And so, it's an interesting situation to be in where, almost no matter where you are in the journey, almost the same lessons can apply downstream and upstream, right? so those are all extremely important. And I'll say that the last one which I think Covid again put a lot of pressure around is this intelligent supply chain.
Thinking through how do we Optimize the supply chain. And again not that any industry wants to lose a batch or wants to have a quality or a recall but when you're thinking about specialty medication, and if you have an equipment failure in the middle of the batch and you lose the whole batch we're talking about very significant impacts to their financial story for that day.
And so, I have a client where, we had AI that was telling them that we thought that it needed preventative maintenance and working with the manufacturer, and they said, no, everything looks good. And we had built such a deep relationship with this client and said, everything about what we're seeing here, the algorithms say that this is not the right trend and that this is a risk.
And the client. Who had, really gotten to the point where they trusted this and had, of course had some big wins up until that point. Said, we're gonna go forward and do predictive maintenance on this. And pulled the parts out of the system, sent them out to the manufacturer and lo and behold, we get a phone call that says, we don't know how you caught it.
But this would've failed within two batches. And so I can tell you that particular client and that particular use, like they always trust the algorithm now. But, batches that are hundreds of thousands of dollars and to have a miss on that, it's just too expensive.
And so, those are the stories and the examples that I think when they permeate an organization, Then the degree of trust goes into a level that, it's not AI that might be scary or untrusted or, we still have clients I think to this day that, that kind of make decisions based on their gut.
Right. And while I can appreciate that institutional knowledge and, and passion I think we're at a point and a level of expectations from all shareholders that, things need to be. As accurate as possible and I think AI can really propel us there.
Richie Cotton: Fantastic. I'm glad that batch story, the predictive maintenance had a happy end. Was uh, a a bit touchy. Go for a minute there.
Tracy Ring: Getting dark there for a minute. Yeah.
Richie Cotton: so, loads of great examples. Again, one of the things you mentioned was about using AI for regulatory filings. that surprised me actually because I think when you have regulations, you need everything to be absolutely perfect, I guess, and AI is prone to making mistakes that maybe humans. Would've noticed themselves. So can you talk about how that interaction between AI and regulations, like how
Tracy Ring: Yeah. and and I'll share with you my favorite very basic rubric of when to use generative ai. And so, the first question one must ask themself is do you care if the answer is correct? And if the answer is no, then it's yes. Go ahead and use generative ai. And then the follow on question.
If you care that it's yes, then the question is are you uniquely qualified to confirm the results and to test them? and if the answer is no there, then you should not now. I do not believe that in very few exceptions, none of which that, that are in my industry, and certainly not in regulatory filing or drug development or anything along those lines.
There is not a human aspect of this. And so, yes, AI makes, mistakes. Yes, humans make mistakes. This is, I think the perfect balance of getting to the point where, Humans can focus on the things that are most important. Humans are not correcting the things that are fatiguing or mundane or commoditized.
And the other piece is that when you think about training a model in a way that you can take all historical regulatory filings and train the model and say, every time we file, we get this type of feedback. But 10 different people are filing, so they're not all talking amongst themselves and catching it.
They might not, do regulatory filings all the time, right? So you can take that compendium of knowledge, train the model so that the model's getting smarter and saying, oh, well we've made, that same initial mistake. And we can catch it proactively, right. The nudging that I talked about.
And then the other piece of it is that again, ensuring that all of the things that, that make us compliant with this are still in place. And so we're not talking about usurping any governance or going around any process that's required that a human be involved. I think we're talking about.
Creating smarter models. We know that AI is gonna get smarter. The more that we use it, the better that we train these models. And that's really, core to the genesis of all this. So, yeah, hopefully that, that rubric of when to use is helpful. I hear people saying, oh, I, I used it to write song lyrics, or I used it to write my Christmas cards, or that.
Those are all perfectly wonderful because if your Christmas card isn't correct, hopefully you'll catch it or Hopefully everybody will laugh at your ai generated greetings. But we're talking about a different level of importance here. And despite I think chat, G p t being the one that most people are familiar with generative AI is a completely much broader aperture of the value we're seeing across the industry.
Richie Cotton: I do like your heuristic for like whether or not to use ai, but that last point about is not just about chat G p T. Do you have examples of some other AI that are in use?
Tracy Ring: Yeah, I mean, I think we have everything from, computer vision where we're using, super computer enabled computer vision to, check shop floor compliance to check for. All types of ways that we're using. Sensor data coming through and I think that the sort of like I take a step back and say large language models and the root of chat, G P T is now new.
We've been doing this since the fifties, right? The difference is we're able to paralyze it, we're able to check sentiment, we're able to do it at a speed this's meaningful. That's what's changed. And then of course the final tranche of this, which is that it, it is totally democratized and that it's in everyone's hands, right?
So, this is, I would just call it the latest incantation of how we're implementing AI with a lot of focus in and around it. But it's really in and around everything that we are doing already. I.
Richie Cotton: so one of the thing we, we all talk about, like, basically related to this idea of regulations is the idea of data privacy and data security. So are there any concerns you have for life sciences applications? I mean, I know you've got a lot of personal data and health data, things like that.
So how did it affect.
Tracy Ring: I think it goes without saying, and I would actually go in so far as to say that, while we're piloting and ideating around, ways that you can do regulatory filing or do content filing in a way that's compliant. So much of this is, let's call it the last 90 days, right?
And we're piloting and we're testing and I don't have anybody that is, fully adoptive and have a gen ai, regulatory filing process, right? We're at the tip of the spear here. I think understanding and recognizing that, Because of where we are in the process one, data privacy and security is paramount.
we're dealing with, health data here and that is, highly regulated. It's one of the areas where I feel like my friends that are in, in banking and in other areas of or healthcare We have access to data that, that I think needs to be really finally curated.
And as I mentioned before, this concept of when could, should I combine data or should I expose data? I think it's a fantastic scenario that we've democratized so much technology but democratizing all data is not the genesis of this. And we need to be, the guardians of this in a way that is.
I don't even know if I would say the same as that. It always had been before generative ai. I would go into far as saying that we need to be even more cautious. It's always, for me a big divide when I talk to CDOs of are you the guardian of the data and you try to hold it and encapsulate it or do you try to democratize it?
And I think most CDOs grapple with this because so much of this is about owning and protecting the data. But on the con side of that is, is that you do wanna, make sure that it's democratized in the right place. So, that process that we talked about earlier as far as how do you embrace programs and how do you embark on your data journey and how do you think about all of this differently that data privacy and security is material importance.
And I'll offer one last bit, which is, Public boards are, I think within 90 days of needing to put somebody on the board that is a cybersecurity expert. And I think that speaks so much to what we're seeing is that, this idea that protecting data, preventing data leaks and guarding all of this is, is absolutely paramount when we think about, this is a requirement for board level that's embraced and tons of research out there that.
why this has been brought as a board level decision for all public companies. So, something that is a, I would call it a huge bellwether for how we should think about AI going forward.
Richie Cotton: Absolutely. It does seem. Like, there's a lot of potential for doing something really stupid with patient data there. Okay. So, hopefully most companies are gonna be smart enough to avoid that sort of thing. I'd like to go back to what we were talking about at the start, which is data modernization, data transformation.
I was wondering where AI fits into this. So, I guess to begin with, like what sort of AI tools or certain analogies are you gonna be getting started with when you say, okay, we're embarking on our grand AI modernization program.
Tracy Ring: Yeah, it's interesting. I was talking to someone the other day and I said we're in a space where yes. Everyone who is let's call it a data worker, I think is getting an education around ai. I think simultaneously all the technologies are building AI in, right?
most organizations that are having success and, and traction within the broader data ecosystem. Whether you're talking about data integration, data hosting data, Quality, et cetera. It's in that instance you're looking for it to be AI enabled, right? And so it's part of the data backbone.
I'll share that one of those organizations. For a while I was part of their product advisory board and we talked about how they were making a modernization to the cloud. And I said, so are you guys rewriting the whole backbone? And they said, to be candid, we did a ton of tests around is the old, let's call it legacy code base plus ai.
Better than a all AI code base that we've freshly built. And they said time and time again the legacy code base plus AI is beating every time. And so this idea that everything that, that is going to have some AI in it, I think is the chart of the future.
So whether you're talking about your C r M system or your e r P system or your data lineage and data governance they all have a little AI in it, right? And, And so, we're seeing the software technology partners and of course all of the cloud vendors not only have what I would consider sort of their AI solution.
But then they're AI built in. And so it's definitely both a capability as well as a way that they're solving for the foundation.
Richie Cotton: that's really interesting. I'm curious as to how you got trying to measure. Or estimate like what the impact of AI is going to be.
Tracy Ring: This is another trick question, right? In some cases, like if you think about analytics or if you think about, matching engines or, we've always measured these, we've always measured your data quality. We've always measured whether, you have just in like a traditional MDM system, number of, accurate matches, mismatches, right?
That this has always been something that, that we've tracked. I think with without a doubt, I think the way that we'll measure AI will be something that, we're learning as we're going along. And will continue to be something that we anchor on. maybe in a year the answer will be quite different and it won't be such a trick question.
But I think efficacy, safety accuracy, at least for me in life sciences are always at the core of, of what we champion. And the ways to ensure that all of those are more accurate are at the heart of what we do.
Richie Cotton: Yeah. It just seemed like maybe it is too early to get like a very precise answer on like, how these things are gonna play out. We need a bit more data to figure it
Tracy Ring: exactly. Yeah. That we've not accurately trained the model.
Richie Cotton: Alright, so related to this if you tried to build AI capability, you're gonna need staff for that. And so what sort of roles would you be looking to hire?
Tracy Ring: before we talk about how do you hire to that, I would ta I mentioned earlier this idea of AI fluency, and I would be remiss to tell you that the organizations that I believe that are getting it right, Are thinking about AI fluency, not just people that sit in technology, not people that might have let's call it business or product owner roles.
We have a really nice study that, that Accenture did where they talked about an organization that embraced AI fluency. Everyone, the entire organization, whether you sit in front of a computer every day or whether you don't and in a really remarkable story that, that somebody who worked in the oil and gas industry who had done the AI fluency training and afterwards that they went home and wrote an algorithm that saved them a million dollars in predictive maintenance.
And this idea that the people that are closest to the business, the people that are closest to the core, whether that's manufacturing or interfacing with your clients can at times be the best AI workers that we might have. And so, do I think that there's an archetype and a capability that we look for?
Most certainly, right? We're looking for people that have, analytical and AI skills. But at the heart of it I wanna make sure that it's not. Exclusive to that cohort. I think that it's opening careers and opportunities for people that, that might not traditionally have been in the space.
And that to me, as somebody who works in and around diversity and inclusion is an incredible piece. I tell the story quite often in the use cases is extremely old. But someone that an organization created if you remember the first time that we had automatic hand sensors, right.
Well, the individuals that created the entire device all, their skin color was exactly the same. And so the first time they installed these hand soap sensors in an area where everybody did not have Caucasian skin. They didn't work because they hadn't tested it on someone whose skin was not the same as their own.
And so I think for me, one of the biggest things is when you are recruiting, Yes, we're looking for core technical capabilities, but it's also about how are you bringing forth a diverse team and how are you making sure that not only are the teams diverse in backgrounds and gender and in all of those things but how are they thinking about things in challenging status quo?
We are at a place where, as I mentioned, that Being guardians of our data and being judicious about how to build in a responsible way. I truly believe the most important thing is to do so with diverse teams.
Richie Cotton: That's a brilliant important point. And just related to that so you work with the W L D A, so, the women leaders in data and ai. Can you tell me a bit more about what you do with that?
Tracy Ring: I guess I'm in three years now. I, it's all of Covid. I feel like it's a little bit of a time machine, but I was asked to join on TODA as a member. And so the charter being a invitation only group of women leaders and data and ai with the idea that.
There would be a cohort of individuals that were supportive and that doesn't just mean it to women, it's women and allies that are passionate about creating and sustaining careers for women in that field. And so, I've had the opportunity to be a member for a year. I've sat on the board for two years and really anchored on, not only how do I help women and coach women and support them in their careers and their trajectory.
But I always feel like I, I know when I've done a great job when someone comes to me and says, Hey, can you talk to my daughter? Because, yes, I can absolutely talk to your daughter or your niece or whomever, but I know that the fact that you're asking means that you're an ally and that you're going to build diverse teams and whatever whatever that may look like you're a better advocate.
For that. And so, very excited and proud to be a part of that organization. Not only because it's about helping women in their careers, et cetera, but it's really anchored on, creating the type of an environment where not only. Tech fluency is something that I don't think could be more important.
Right. We're talking now that I certainly don't have the answers to everything. And part of that peer-based learning and networking, and twice a year they have a summit as well as a retreat, to really invest in. Taking a pause and saying, I'm gonna make some concerted effort in learning and growing and contributing back so that not only am I a better leader for myself, but also for my teams and for my clients and for my family.
Because we are really holistic in individuals and how we show up and deliver. So, very proud of Willow's mission and the impact that they are having.
Richie Cotton: That is fantastic and it always seems like such a, an easy, weird win, like you're making a better workplace. And then you gave the example of like the product that went wrong, the hand sub dispensers, cuz. They didn't have the diverse team, so you're making more money as well. great. All round. I think sometimes the practical aspect of this can be difficult.
So, from your welder experience, do you have any sort of practical tips for people who want to, or managers who are hiring, they want to go out building more diverse or inclusive teams?
Tracy Ring: Yeah it's interesting. and while I do a lot of work as it relates to gender diversity, I think we all can have unconscious bias that slips in and things like that. And so I think some of it is about taking a bit of a look and we know Harvard did a great study about the age of a team and, how old is your team based on, you know, how effective are they?
And so, Richie, we only met maybe a month ago, so our team, you and I are a month old. Somewhere around two years we would optimize our team in the moment that we had someone else then the age of our team would go down again. And so this idea of if your team has been working together and they all know one another, and I'm sure that feels very comfortable.
You probably should take a look and consider some ways to add some new talent to that. I think also, the great resignation has a lot of very new teams, which is also at times not the most effective. And so, try to think about how do you create within all of your teams a bit of a challenger network?
How does everybody have. The ability to raise their hand and create a safe space to say I'm curious about this. I'm not sure about this. One of my favorite people to read and listen to is Brene Brown. And she talks about how within her team when there's a big decision to be made, she realized what was happening is that she would answer the question first and then everybody would follow suit.
Right now when there's a big decision to be made, she writes down her answer, she turns it over, and everybody else has to go first. And it doesn't matter that she might have a certain opinion, but she realized that she was biasing her whole team and they were, following the leader, so to speak.
And so we all, I think whether it's how we're forming teams, how we're growing teams and even how we're running status calls we all can, be watchful of how we're itself in introducing bias and catching ourselves. And really thinking about new ways of working.
We've spent the last several years working in new ways and I think now we've pattern ally fallen into some new ways. So, I'm eager to say that for the most part we're back in front of our clients. We're back inside workplaces. I know some people are not so excited to be back in the office.
But I think it's a, it's an opportunity to think about how we shape and grow and cultivate the conversation and, and for me it's always about are we having the conversation or. Is there the opportunity to have the conversation or ask the question. So infinite curiosity is something that I always try and instill in, in all my teams.
Richie Cotton: That's brilliant. And I guess we have to keep continuing to work together for the next 23 months until we peak. Excellent. So I do love that tip actually about the making decisions in meetings because it's so easy to have a rubbish meeting where, Nothing gets done or you come to the wrong answer after wasting an hour. So, all these tips for like how to do meetings better are absolutely invaluable.
Tracy Ring: it's funny I'll, I'll share with you every once in a while I can tell my team is like, messaging each other. oh, this was a rubbish idea that Tracy had, or this is, you know what I mean? Like, or there's, you can see it, particularly if people are on video and so I think this is about catching it, teasing it out, and creating a safe space for people to, to just tell me that my idea is bad in real life.
Richie Cotton: Always a great moment when you can tell your boss they've had a terrible idea that's One of the, those work workplace wins. Brilliant. Alright, so I just to wrap up, do you have any final advice for people think about data or AI in life sciences?
Tracy Ring: Yeah I think a couple of things which is Never has there been a time where I think being our own advocates for our healthcare is more important. We've really lived through an incredible experience. And I would share with you, I am every single day impressed with what's happening in the industry from every single aspect.
And so I would say I'm very hopeful about the future. I'm very hopeful about Better care, better experiences, and better outcomes. those are things that, that I am truly passionate that the data and AI that we're all working towards is improving every day.
And I think that, it's been a real delight, I think, even for me personally to see that, the doctors and the caretakers have had to rely on data in a new way. And I think even, we all became experts in watching covid charts over, over the last couple of years.
And so I would say that spirit of I know much of your audience is already so, so passionate about data. But I know that you know when you hear things, my aunt called me the other day and she said I heard about this program that's better for helping me write song lyrics.
She teaches ukulele lessons and I thought she's gonna say it. And she goes, it's chat. And I thought, oh my gosh, this is it. My, my 65 year old Anne is using chat G p t. And so, for those of you that, well, well, it's a small microcosm of the broader world of generative ai. I would say go out, see what it's about and, and continue the experimentation cuz it, it is an exciting, fun time.
Richie Cotton: Absolutely. Uh, alright, brilliant. Uh, thank you very much Tracy. Uh, So much insight there. That was brilliant. Uh, thank you for coming on the show.
Tracy Ring: Absolutely cheers.
blog
Building trust in AI to accelerate its adoption
DataCamp Team
5 min
podcast
Building Diverse Data Teams with Tracy Daniels, Head of Insights and Analytics at Truist
podcast
Creating an AI-First Culture with Sanjay Srivastava, Chief Digital Strategist at Genpact
podcast
Intel CTO Steve Orrin on How Governments Can Navigate the Data & AI Revolution
podcast
Scaling AI Adoption in Financial Services
podcast