Why ML Projects Fail, and How to Ensure Success with Eric Siegel, Founder of Machine Learning Week, Former Columbia Professor, and Bestselling Author
Eric Siegel, Ph.D., is a leading consultant and former Columbia University professor who helps companies deploy machine learning. He is the founder of the long-running Machine Learning Week conference series and its new sister, Generative AI World, the instructor of the acclaimed online course “Machine Learning Leadership and Practice – End-to-End Mastery,” executive editor of The Machine Learning Times, and a frequent keynote speaker. He wrote the bestselling Predictive Analytics: The Power to Predict Who Will Click, Buy, Lie, or Die, as well as The AI Playbook: Mastering the Rare Art of Machine Learning Deployment. Eric’s interdisciplinary work bridges the stubborn technology/business gap. At Columbia, he won the Distinguished Faculty award when teaching graduate computer science courses in ML and AI. Later, he served as a business school professor at UVA Darden. Eric also publishes op-eds on analytics and social justice.
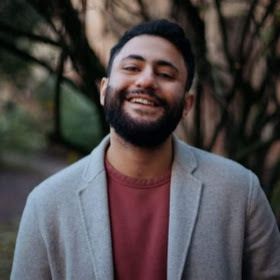
Adel is a Data Science educator, speaker, and Evangelist at DataCamp where he has released various courses and live training on data analysis, machine learning, and data engineering. He is passionate about spreading data skills and data literacy throughout organizations and the intersection of technology and society. He has an MSc in Data Science and Business Analytics. In his free time, you can find him hanging out with his cat Louis.
Key Quotes
AGI is the modern day ghost story, and that as human-like as large language models are, and image generators are in a sense, there's a big difference between what they do and what humans can do, and we're not nearly,it's not just that we're not close. It's that I think it's a mistake to consider what we're seeing now as a concrete step towards AGI
How an ML system is embedded in the business process it’s affecting needs to be conceived of from the inception of the project, because the whole point is to improve operations. The point of the project is not to use cool technology. I might be working as a data scientist because I love cool technology. And indeed I've been in the field 30 years and definitely that's why I got into it in the first place myself. think that's the same for a lot of data scientists, but we're not running this project. The organization is not running the project because it's cool technology. It's running the project to improve operations. So if you're planning a project that improves operations, you also need to be able to plan to measure and track how well those operations have improved in business terms, moving forward. And I think we'd hopefully like a little bit more than that too. Not only the sense of how well the model's performing, both technically and in terms of the business metrics, but also continuing to enable an interactive trial and error of what if scenarios. What if we had over the last few months, what if we had changed the way this model has been deployed, changed the confidence threshold, changed some of the assumptions, the integration, what would that have done? Because we're gonna get ground truth later, right?
Key Takeaways
Success in deploying machine learning projects often hinges more on organizational alignment and collaboration than on technical challenges alone.
Utilize the BizML framework to ensure machine learning projects are aligned with business objectives from inception, involving stakeholders across the organization.
Encourage both data scientists and business stakeholders to develop a semi-technical understanding of machine learning, focusing on what's being predicted, how predictions are made, and the actions taken based on these predictions.
Transcript
Adel Nehme: Hello everyone. Welcome to DataFramed. I'm Adek Data Evangelist and Educator at DataCamp, and if you're new here, DataFramed is a weekly podcast in which we explore how individuals and organizations can succeed with data and ai. I think we can all agree that we are in an AI hype cycle. Every executive looking at the potential of generative AI today is probably thinking how they can allocate their department's budget into building some AI use cases, and probably a lot of these use cases won't make it into production. I say this with relative certainty because we've been in this hype cycle before. Hype around machine learning in the early 2010s led to lots of hype around the technology, but a lot of the value did not pan out.
For example, according to VentureBeat four years ago, 87% of data science projects did not make it into production. In a lot of ways, MLOps was a response to this deployment crisis, but things have not gotten that much better. And if we don't learn why that is the case, I believe generative AI could be destined to a similar fate.
Enter Eric Siegel. Eric Siegel is most known for his consulting work and previous role as a Columbia University professor. He founded the Machine Learning Week conference series and its counterpart generative AI World and teaches an online course on machine learning leadership. Siegel is the executive editor at the Machine Learning Times and a renowned keynote speaker.
He authored the best selling book, Predictive Analytics, the power to predict who will... See more
Machine learning use cases need to solve business problems. And machine learning use cases need to be scoped in collaboration with business stakeholders. This is what Eric calls BizML. I highly recommend you read the book. The link to get it is in the show notes. In our conversation today, we delve into the reasons why machine learning projects don't make it into production.
How to bring business stakeholders into the room when building machine learning use cases. What the previous machine learning hype cycle can teach us about generative AI. And a lot more. If you enjoyed this episode, make sure to let us know in the comments, on social, or more. And now, on today's episode.
Eric Siegel, it's great to have you on the show.
Eric Siegel: Great to be here, Adel. Thanks for having me.
Adel Nehme: Thanks so much for coming on. So it's been four years since VentureBeat released this widely cited yet hard to corroborate article describing how 87 percent of data science projects never make it to production. The big focus of yours the past few years is really deep diving into how organizations can make the most of their machine learning investments.
And your most recent book, which we'll discuss in depth today, the AI Playbook. focuses on this exact problem. So maybe to set the stage, how has the state of machine learning deployment evolved over the past few years since that article was released? And why is it still such a big problem today?
Eric Siegel: Well, I think a few years, it may be a relatively short timeline and how long projects have been out there and also failing to deploy. I don't think much has changed, but what has changed the last few years is we have a lot more concrete stats. I was involved with some research projects in part as a one year analytics professorship at UVA Darden.
And I did that in conjunction with the Rexer Analytics data science survey. And we found in the results that among new capability initiatives, only 22 percent of data scientists say their projects usually succeed deploy. More generally when you go across all projects, including just refreshing a model, it's a bit better, but in that case, 32 percent say their models usually deploy.
So, we don't have a direct match there, but we're definitely seeing that I think you're potentially gonna link to that particular research result and we're linking to other ones. So there's a bunch of stats. IBM recently came out with industry research saying that there's no returns.
That is to say, the average return of an AI project is lower than the cost of capitals, on average. Now, of course, there's many glowing successes, but there's a dismal track record that stands much to improve. And me thinks it's an organizational issue more than a technical one.
Adel Nehme: So you mentioned here the technical issue, you know, I think a lot of the conventional wisdom when it comes to why these machine learning projects never make it to deployment or drive ROI um, the conventional wisdom says that, it's the lack of MLOps or capabilities within many data teams that has been the main culprit of why this is the case.
In many ways, the birth of MLOps is a subfield of machine learning, is a response to this dismal track record that you described. But in your book, you go beyond that, and you discuss how, you know, the current paradigm by which we approach machine learning projects, and you hint at that as an organizational issue, is in dire need of change.
So maybe walk us through that current at the moment, and why is it lacking?
Eric Siegel: I think that another technical approach MLOps is necessary but not sufficient. hinging on that as the solution to this deployment problem is just a continuation of our over fixation of the core technology and the technology in general. This is an organizational issue, and what it needs is a standardized practice, which I offer in my new book, Everyone needs to get on the same page, both in the data science technical, and on the business side, the stakeholders across the organization, and follow a paradigm, a procedure, a discipline, a playbook, right, that everybody understands, so that they can participate and collaborate in detail from end to end, from the inception of the project to its deployment.
That's where we're going to make a difference. And along the way, Most certainly, there'll be ML Ops techniques and tools that are adopted to that end. But the the dog that wags the tail has got to be an organization that's a business project meant to change business operations by way of using machine learning rather than being a machine learning project that, as a side effect, helps the business.
Adel Nehme: And maybe why do you think that we, organizations, you know, despite this current track record, that is, pretty poor from what we've seen from the stats, still operate in this failure mode where they approach machine learning projects as, you know, a purely technical project that has a side effect of helping the business rather than the opposite.
Eric Siegel: Because we haven't had enough conversations like this, maybe this'll be the one that brings us over the edge. It's this fetishization of the core technology. Machine learning is awesome. That's why I got into it originally. The ability to learn from data, to find generalizations that hold over new situations, to actually learn from data in that respect.
It's really awesome. It's very exciting. It's quote, unquote, the most advanced cutting edge so decision makers rest assured they're using the best technology and with all the hype now, especially because it's multiplied so much by Gen AI hype, these failures, when a project actually doesn't get to deployment and therefore, of course, offers no returns are swept under the rug and they're swept under the rug Adeptly, people protect themselves by doing that.
Organizations protect their reputation. And it's only natural. So there's definitely Zeitgeist building more and more of these reports about the track record and concern about that. Executive awareness. It's definitely changing. Running on the sort of fumes of hype and excitement about the technology rather than its actual deployment isn't sustainable.
And it's going to come to a point of crisis, unless people sort of get out ahead of it. And that's what I'm doing. And what you're helping me do, talk about this and try to bring this idea of a standard business paradigm. So it's sort of like right now, the world's more excited about the rocket science than the launch of the rocket.
It's kind of like, hey, this rocket science is so cool. we could launch the rocket maybe next week, maybe next year, but it doesn't really matter. The science is so cool, right? And some rocket scientists may actually feel that way, but you know what I mean.
Adel Nehme: Yeah, I completely agree. And there's someone that I worked with in the past that called this a resume driven development, Where it's not actually focused on driving value. It's actually focused on, you know, being able to showcase the shiny toy that you're working with and you're working on as an organization.
And you mentioned this business paradigm and leading with the business, as an organization. You mentioned this in your book, you call this, you know, aptly called BizML, what this paradigm tries to do is that it attempts to bridge the gap between the business and the data team, So before we go into BizML, maybe walk us through what this gap looks like. Why does it exist and how it manifests within organizations?
Eric Siegel: So the gap is that, both sides are pointing to the other towards taking ownership of running an organizational practice that will successfully lead to actual deployment, capture value. You know, machine learning is a technology that's great at generating value. What we want to do is actually capture it by way of deployment successfully and carefully in a way that meets the business strategy and needs.
My message is that to bridge this gap, The business stakeholders, the business professionals, your client as a data scientist, needs to ramp up on at least some semi technical knowledge, and that is what's predicted, what's done about it, and how well it's predicted. So, this isn't about the core rocket science, it's not about the machine learning algorithm or even what's under the hood inside a model itself.
Although there's certainly no reason to get assigned. When I drive a car, you know, I have a general sense of how internal combustion works. But I don't really need to get into the nitty gritty and I've never changed a spark plug. To be honest, I've actually never done that.
But to drive a car, I need a lot of expertise and understanding momentum and friction and how to steer and the rules of the road and expectations of other drivers and my expectation of their behavior. And theirs of mine. So the same applies to running a machine learning project. Operations improvement.
project really is what we should reframe it as that uses machine learning. And by getting the business stakeholders ramped up on that kind of semi technical knowledge, it's definitely less difficult than high school algebra, a heck of a lot more interesting and pertinent. It's about running your large scale operations more effectively.
What's predicted, how well, and what's done about it. If we get them up to speed on those basics, then they can speak the same language and participate and deeply collaborate. From end to end, backward planning for deployment from the inception of the project. And by having them involved, you're not going to get to, so the syndrome of what happens now towards the end of the project, Hey, we're ready for deployment or we're almost ready, or this model might be ready.
And then the stakeholder often gets cold feet. They don't understand. They don't understand the metrics. They understand, Hey, a model predicts better than guessing. And that's probably good enough. Doesn't have to be a magic crystal ball. Better than guessing. is generally sufficient to drive tremendous impact on the bottom line of improving the numbers games that we play with all these large scale operations and marketing and fraud detection and credit scoring and online ads and all this, but they don't have a concrete sense of the math.
And that's the second of those three. What, what's predicted, how well, what's done about the metric. So, by ramping them up to that point of that certain kind of data literacy, then they can participate and they're not going to get cold feet at the end. They're going to have a concrete sense from the beginning of the project and from its green lighting of what exactly deployment will entail?
Adel Nehme: So we talk about data literacy quite a lot on Dataframe, so I'm excited to unpack, you know, your views on that. But maybe first let's take a step back and look at the BizML framework in a bit more depth. you outlined the BizML framework in quite a few steps throughout the book. So maybe walk us through that framework in a bit more detail and what those different steps look like.
Eric Siegel: Yeah, sure. So, BizML, we break it into six steps, and the first three correspond to those three fundamentals. What's predicted, how well, what's done about it, but not in those order. So they're pre production, you're establishing those, and the other three are the same thing you do with any ML project.
Everybody who's involved with ML knows this. Prep the data, train the model, and deploy. Obviously, you need to monitor moving forward, but we're framing it just trying to get to deployment. We talk about that later in the book. BizML, as I'm formalizing is those six steps. You could formalize the project as five steps or as seven steps.
People, at least senior data scientists, are familiar with the idea that you need to conceive of it into that kind of organizational process, but there's no standard that's generally known, especially the business side practitioners. In fact, your stakeholders, your client, the business side, generally don't even know that machine learning projects really require a specialized kind of business paradigm.
So what I'm trying to do here is send two messages. Ramp them up on those three semi technical concepts. What's predicted, how well, and what's done about it. And the need for a paradigm. Let's give it a nice buzzword. As you said, BizML, So that's actually the domain for my book.
BizML. com. So. I put in several hours, people, picking out just the right five letters for what I think could be an awesome buzzword just to help evangelize this whole point. But let's all get on the same page for the need for a standard business side paradigm or playbook. Let's agree to how to break it down to six steps or something like that, and the need for that collaboration and the ramp up on the business side.
So that's my message, and I think that's the antidote. I think that's where we're going to get a lot more traction, not just in how excited and hyped up everyone is about machine learning, but in actually getting value driven deployment oriented projects, and greatly improve that track record.
Adel Nehme: And, you know, you talk about this collaboration element here. I want to zero in on that. You know, when I look at data teams in general, when I speak to data leaders, I'm much more involved with the data leadership aspect. And what I get a sense from when they speak about data science and machine learning projects is that there seems to be an expectation that both parties have between business stakeholders and data scientists and data teams.
They expect the other teams to, own the main drivers of the ROI of a machine learning project, right? Data scientists see themselves as the architects of a technical solution to a business problem that the business stakeholders should own. And business owners see themselves as handing off to a certain extent the problem to the data scientists and the machine learning engineer.
So maybe how does ownership and collaboration evolve under the business ML paradigm? How should data teams and business stakeholders evolve their collaboration and their approach accordingly?
Eric Siegel: That's a great question, and it's the pertinent question. I'm agnostic about it, and I've framed BizML in a way that it can go either way, or some combination, or a new role, or a new responsibility of the Chief Data Officer, whatever it is. The point is that somebody's got to take it on. And if the organization agrees that, hey, we need to follow a specialized business paradigm so that we actually plan and collaborate accordingly so we can get this thing deployed, not just the number crunching not just a model that looks pretty hanging on the wall then in order to execute on.
this business paradigm, obviously somebody's going to need to lead it, take responsibility. You're very much right the way you put it, that both sides tend to point to the other as a responsibility. And in that way, the hose and the faucet are failing to connect, right? Data scientists think, well, that's all kind of managerial stuff and my job is just to make a model.
It's value self evident. It's not my responsibility to get it deployed. Of course, the organization will deploy it unless they're nuts. Whereas at the same time business professionals say, I don't need to get into those details. I delegate all that to data scientists. So you know, I don't need to look under the hood of the car in order to learn to drive it.
But as I mentioned, no, they do. The organization, business leaders need to learn semi technical, not the core rocket science, but how well the rocket science works, how it's predictive outputs, the probabilities output by model will specifically integrate, will actually drive individual organizational decisions, that level of detail.
And once you start talking to a business stakeholder, well, this is what I mean by the, by semi technical, you don't need to be changing spark plugs, but you do need to be getting a sense concretely of how this is going to deliver value and how much value it's going to deliver. It's not that crazy. And they're like, Oh, Okay, I don't have to be a rocket scientist, I don't have to actually crunch the data myself, but there is something kind of semi technical, and from the data scientist's perspective, what I'm referring to as semi technical, again, what's predicted how well and what's done about it isn't technical at all, and from the business person's perspective, it's often extremely technical.
So it's, there's this long continuum between the two sides that we need to get to connect. So let's agree to sort of midpoint that everyone speaks that same language. It's a sort of a stretch for both sides, but otherwise we're just going to continue the same track record.
Adel Nehme: Yeah, agree there. And then you know, let's maybe take an example. In a lot of ways you know, we talk about here creating that collaboration and someone owning the agenda on both sides, right? Let's say we're starting to scope out a machine learning project. Who should be in the room?
How do you get started? How do you make sure that, you know, you start on the right foot when it comes to, you know, enabling that BizML paradigm to succeed in your next machine learning project?
Eric Siegel: Yeah, it's a great question. Like who's in the room when you're make, when you're conceiving of the project, when you're preliminarily authorizing it, eventually really greenlighting it, investing more and more resources incrementally, all those early meetings and who's the driving force? So the answer to those two questions are very much overlapped as far as who's in the room, Often it's going to be a data scientist, one that's senior enough or for thoughtful enough to realize They're not just there to crunch numbers, but to provide value to the organization. They need to take the bull by the horns in this respect. They're the ones already familiar with what it means for a model to predict, and then they're at least conceptually familiar with what it means to.
take predictions and use them to drive operational decisions, who to contact and which transaction to audit or whatever it is. so the same question corresponds to, does this happen from the top down or bottom up, right? Is it somebody who's, on the staff who's really doing the number crunching or is it literally the CEO, right?
It could come from either side, it could come from both, but it's got to come in a way that's very specific and concrete in terms of the value proposition, the use case, which is two of those three things, what's predicted and what's done about it. Instead of this, hey, what's our AI strategy or let's use machine learning somehow.
it's got to start with that level of detail, but then get a lot more detailed not only from the data scientist side, but from the business side. So again I'm, agnostic. It really depends on the organization. And it varies greatly from project to project and organization to organization.
But however, it's sort of starts to evolve and emerge, It's got to become deeply collaborative. One side's got to pull the other side in.
Adel Nehme: Maybe when you talk about collaborative here, I think you know, a big obstacle data teams and business teams have when it comes to collaboration together is defining successful metrics for a machine learning project. And what I mean by successful metric is not accuracy or precision or recall of the algorithms and their performance, but, you know, the business impact, quantifying the impact of a incremental improvement in the performance of an algorithm from the business's perspective, So maybe what is your advice for whoever is in the room here quantifying the business impact of a machine learning project?
Eric Siegel: Yeah, quantification is absolutely key. It's the second of that list of three that I keep repeating. What's predicted, how well, and what's done about it. What are the metrics? And yeah, you're totally right. The main metrics that we focus on as data scientists are not the most pertinent metrics, and they're sometimes necessary, but never complete.
You need to go to business metrics, profit, number of customers saved, number of lives saved number of dollars saved things that any stakeholder can understand, things that are pertinent to the business, and it turns out. If we haven't noticed, that there's a really big disconnect between the technical metrics like precision recall and even accuracy is just a technical metric that doesn't differentiate between false positive and false negative cost.
It's usually impertinent and often very misleading. But anyway, all those tech, maybe the most egregious is area under the curve, area under the receiver operating characteristic curve which has the same problem, for example, as accuracy of not differentiating between those the costs of those two different, different kinds of errors.
But in any case, let's move to the business metric. And the relationship between the two is elusive as, at best. You don't translate directly from one to the other. You have to measure and forecast how well will this model potentially serve those business metrics like profit and ROI or what have you. or how well has it already when you're monitoring after deployment.
Either way we need to track organizational success. And this is a really key point in bridging that gap. In fact, I've co founded, this isn't even public yet, but I've co founded a startup called Goodr AI, to make your AI more gooder, that focuses on measuring the performance models in business terms.
And the trick is, so my experience is that there are many, tends to be more senior data scientists who have the wherewithal to say, Oh, you know what? We do need to calculate profit. We need to make a profit curve or what have you. And when they decide to do that, if and when a data scientist wants to, existing model training tools don't do that for the most part, right?
You have to hack it from scratch in a bespoke manner, often in Excel or in a scripting language or What have you, then you get a static report. But it turns out when you go to those kind of business metrics, there's a little bit more that you need to do, which is that you need to parameterize in terms of false positive and false negative costs, in terms of the confidence threshold, all the assumptions, the business context, you need to parameterize it in terms of the exact deployment scenario.
A model itself isn't worth a million dollars. It's only worth a million dollars if that turned out to be the, the metric. depending on how you actually use it. So you need to parameterize that deployment, those deployment particulars, and have a nice interactive GUI, an interface where you can set it up and evaluate.
So that's what we're building. If you go to gooder. ai, you're just going to get to my book right now. But, anyone out there, please reach out to me if you're interested in being a beta customer, if you want to have a tool that readily does all the above and lets you do those business metrics.
It's absolutely, I'd say that's the main missing technical component. technical solution in the ecosystem for machine learning that's outstanding when we're talking about getting to business value and ensuring a successful deployment.
Adel Nehme: Let's switch gears slightly you know, When we're talking about the evaluation of the business metric, right? let's say we defined a business metric and we have our forecast, And then we're switching to deployment. I think outside of just operationalization, being able to, you know, embed the machine learning system into, a broader technical system within the organization is how do you also embedded within a business process, think that's a big challenge as well. Organization has have. So, how early in the conversation should we be thinking about? the way by which a machine learning system will be embedded in a business process and how it will be leveraged in daily operations. Maybe walk me through that particular
Eric Siegel: Well, yeah, I mean, I think you've almost, you almost answered your own, like the question itself is a great, you're already making a great point. Yes, this needs to be conceived of from the inception of the project because the whole point is to improve operations. The point of the project is not to use cool technology, And I might be working as a data scientist because I love cool technology.
And indeed, I've been in the field 30 years and definitely that's why I got into it in the first place myself. And I think that's the same for a lot of data scientists. But we're not running this project, the organization's not running the project because it's cool technology. It's running the project to improve operations.
So if you're Planning a project that improves operations, you also need to be able to plan to measure and track how well those operations have improved in business terms, moving forward. And I think we'd hopefully like a little bit more than that. Not only the sense of how well the model's performing, both technically and in terms of the business metrics, but also continuing to enable an interactive trial and error of what if scenarios.
What if we had Over the last few months, what if we had changed the way this model has been deployed, changed the confidence threshold, changed some of the assumptions, the integration? What would that have done? Because we're going to get ground truth later, right? So before deployment, you've got the test set.
That's already the, that's not just the best practice, it's the only practice. You've got the held aside test set. That's what you're evaluating on. You already have the labels or time is told, who clicked, bought. Lied or died, who canceled, right? So we have those dependent variable values. And then you're going to get them eventually after deployment, depending on the use case, you have to wait a while, which of these transactions turned out to be fraud.
But then you have them just the same as pre deployment, and you're once again, retrospectively evaluating the performance of a model. And once again, Do the same thing. Try some what ifs. What if we had done this differently? Well, then maybe we should change that the next quarter. So yes, I think that's a great point that you sort of served up for me.
Right? If this was volleyball, don't you love it when you're playing volleyball and somebody in your Right, so that was great.
Adel Nehme: yeah, I appreciate that. I'm here to provide you layups, Eric. Uh, Yeah, so we talked about quite a bit on the theoretical aspect of BizML. I want to anchor our discussion in a bit of a real world example. And you provide this a great example from UPS and the book.
Uh, how they've leveraged machine learning to on a route optimization use case. Walk us through that case study in detail and maybe walk us through the pitfalls they encounter at the beginning, how they switch around and what you hope other data teams learn from this particular use case.
Uh,
Eric Siegel: That I'm talking about at UPS, they internally call it package flow technology. So it's predicting tomorrow's deliveries and next week's deliveries, et cetera. But it's most active when you're really just talking about tomorrow's deliveries in order to optimize the planning of delivery trucks.
So it's literally the last mile or the last several miles from the shipping center onto the trucks. How do you delegate all those packages to trucks and plan accordingly? And they improve that greatly by predicting because there's a lot of uncertainty. They have a bunch of packages that have already been come to the shipping center, but a bunch more that are still coming.
And there's a lot of reasons that there's uncertainty of about when they'll arrive and whether they're for delivery tomorrow or whether they even exist at all. So it turns out that, that had a great impact. In fact, in combination with not only prescribing which packages go together in which truck, but then also prescribing the driving routes, which some number of years ago was also pretty innovative rather than the expertise of the driver.
Together, that provides UPS an ongoing savings of 185 million miles of driving a year, 350 million, eight millions gallons. 8 million gallons of fuel and 185, 000 metric tons of emissions, saved every year in the U. S. I bookended my book, the AI playbook about BizML with that UPS case study, because I talk about two places where there were organizational challenges.
The early one was getting the green light on the project in the first place, and there you're trying to convince. An executive and then later we're trying to convince the people, staff members working on the loading dock to pay heed to these prescribed behaviors of which package goes into which truck and change their behavior.
And so in a sense, you need full stack organizational buy in. You need to get people at all. I mean, we're changing operations. So this is just change management 101, right? Change management's hard. It's a discipline, there's a lot of ways to do it, and I go through some of that in the book of the cajoling and the aligning incentives and the right sort of short term metrics and scorecards and all that kind of stuff, but the bigger point is that, look, you need to do change management.
And that fundamental is actually overlooked so often because people aren't conceiving this as an operations change project. They're conceiving it as a machine learning project. No, we need to reframe it as an organizational operations improvement project that uses machine learning. But the reason we don't call it that, the reason we call it a machine learning project is because it sounds cooler.
Adel Nehme: I agree. And you mentioned here change management and, big aspect of change management is skills transformation. And this touches upon your early points on, you know, the importance for business stakeholders to develop semi technical understanding of what are we predicting, what's being predicted and how do we measure.
So, I think it's important this business framework for, data teams and business teams to have a common data language So we talked about, the ability of data teams to translate machine learning projects to business impact, but business teams should be able also to understand, to a suitable technical degree, as you mentioned, what goes into a machine learning project and how do we expect that machine learning project to, impact my area of the business?
So maybe walk us through in your view, the importance of upskilling and reskilling here, and what do you see as the basis of this common data language?
Eric Siegel: Yeah, so it's that same three. You don't mind if I say the list of three again, do you? What's predicted and how well and what's done about it. What's predicted and how well, so what's predicted and what's done about it, that's the use case, right? And that's why machine learning is so widely applicable.
Any new potential use case is simply you come up with a viable pair. What's the dependent variable, what's predicted by the model, and then how are you using, integrating in actual deployment those probabilities output by the model what process are you improving, what operation, what large scale number of decisions, and exactly in what way so getting into those details.
And then the second of the three is how well, and that's the metrics part. so there's no standardized curriculum, that list of three and a course on it or a book on it should be standard for all MBA students, for example. it doesn't require more than high school math, the metrics part of it is only arithmetic, but it's very particular arithmetic, and it's not the kind that people are generally aware of, at least outside of data science.
Even data scientists haven't been trained to spend much time on going to those business metrics. And I should mention that the difference between technical and business metrics is technical metrics only tell you the relative performance. So how much better than a baseline, like random guessing.
important, interesting, it's all we're trained to focus on as data scientists. So then we feel satisfied based on the area under the curve or what have you but we shouldn't be satisfied. So that move to, to accuracy and such and understanding the difference in the relationship between technical and business metrics.
but it turns out that this sort of semi technical that I'm not just espousing, I'm strongly espousing that really business stakeholders need to ramp up on universally. Some of that is also not common knowledge, even among senior data scientists. So, For example, everything I'm saying about metrics, like let's break through this, let's look at really what the problem is with AUC.
Let's look at the limitations, precision recall, and what it would take and what it means to transition to business metrics. Also, part of the semi technical, the first of those three, what's predicted, I don't just mean let's predict customer churn, who's going to cancel. It's got to be much more specific, which customers who've been around for at least a year are going to decrease their spend by 80 percent and not increase it accordingly in another channel within the next time window of three and a half months.
All those details, and it might be three times as long a run on sentence, it's a yes no question for, you know, most of these binary prediction goals. That's the definition of the dependent variable. Don't call it a dependent variable when you're talking to a business stakeholder, call it the model output.
In my book, I call it model output because this is relevant to the business. It's not an arcane technical thing. Exactly, precisely what's predicted. And getting into all the gory details, which all those details are relevant from the business perspective. When you think about how exactly those predictions, they're defining what are you predicting, and then you also have to define how those predictions are going to be used.
Those two go together, and in all that gory detail, you need to get the business stakeholders. But data scientists also haven't been ramped up on that exercise, that business exercise of flecting out all the details of defining the dependent variable. Oops, let's call it the output variable. Dataprep, that's another one, right?
If the last three main production steps and the last of six that I call BizML are prep the data, train the model, and deploy it, well, the Dataprep is generally skipped over. And data scientists all their excitement about the core technology, and I'm not immune to this, if you go back in time to earlier in my career, it's like, jump right to the modeling.
Who cares about the Dataprep? But you're skipping over all these pre productions. phases that decide what's going to be done, what's going to actually, how deployment is going to be entailed, which in turn informs the detailed specific definition of the dependent variable, which then is manifested by the data prep, not by the modeling part, you don't.
adjust the modeling according to the dependent variable's definition, no. That is manifested by way of how you prep the data. And getting into all the ins and out of data prep, it's not the fun rocket science, but it's an absolute technical necessity, and it's generally not covered in a very forceful way in data science.
curriculum. So again, my point here is that a lot of this stuff that's semi technical, and that it's important to get the concepts across to business stakeholders and get them upskilled, is also new to data scientists because it's not part of the standard curriculum.
Adel Nehme: Yeah, that's great. And, when, you know, you're walking through these different skill sets and these different kind of concepts and semi technical concepts, you know, that even data scientists need to know about. It seems that there are, you know, generally applicable concepts that, you know, anyone can get into.
But there's also organizational specific concepts. You mentioned like that customer churn metric, right? Customer churn and telecom is very different from customer churn and Netflix is very different from different organizations, right? Maybe walk us through examples of, organizations who've been able to nail that, you know, internal education.
Internally on like really specific data use cases within the organization and how you've seen that play out,
Eric Siegel: Yeah. Well, that's a great question. And there are, as I sort of briefly, maybe mentioned earlier, maybe not emphatically enough, machine learning is not a failed discipline overall it, there's lots of successes, even if they're in the minority a small, whether it's 15 or 25%, depending on how you count and measure the number of projects that actually succeed in land deployments, that percentage of a lot of projects, is a lot of success.
And you know, I think as we all know, there's lots of organizations that are really at the forefront of this not the least of which is big tech. So I spoke to a manager at Amazon and, you know, unfortunately, there's not general solutions to this. that's available.
off the shelf yet, and you know, as I mentioned we're working on this, but Amazon has its own particular solution of mapping performance of models to exact business metrics that they have in mind. And so you're really directly measuring it. And, it's all, it's also makes sense that big tech Would tend to have a more technical mindset across the organization, including the business leaders and managers and executives.
That would tend to be the case there more than a than like UPS, you know, which is more than a hundred years old. And so the change there was a big one. They had to very forcefully push it through and in the end successfully. Another case that I cover in the book is FICO, that is very well known for credit scoring.
Perhaps a bigger part of their business is fraud detection and their fraud detection model scores in real time each card transaction for two thirds of the world and 90 percent of the U. S. and the U. K. of all credit cards in real time because all the banks or most of the banks are customers in use.
So the exact model for card payment fraud detection, it's called Falcon. It's delivered by FICO, and then it's the same best model used across all these banks. So even small banks can use the best fraud detection model. That model and the process has been honed so well over so many banks.
So they know exactly how they want to predict you know, define that dependent variable for fraud detection and churn it out. But then it's up to these individual banks to figure out exactly how do I deploy it, where do I threshold, what's my tolerance for risk of fraud, depending on the size of the transaction, for example.
but in, you know, there's a lots of large financial services organizations that are really also at the forefront and have a well honed process. So, What I'm describing here is, might be rare, but certainly not unheard of. It's a matter of making it pervasive and getting the rest of the world in a position where they can catch up with those at the forefront share in the upside, right?
Because right now what everyone's sharing in it is the hype and the excitement, and it's not yet being matched, But there's no reason it shouldn't be. The core technology is solid. It's a matter of coming down to earth, getting concrete and working end to end in a unified manner that's collaborative with the business side.
somebody who's in charge of the operations that are going to be improved by a model that in a sense is the stakeholder. They're the ones who own the large scale operation needs to be improved. They need to get involved in the nitty gritty and they need to think quantitatively. If they're not willing to think quantitatively, then they shouldn't be in charge of a large scale operation, right?
But again, it's not the rocket science part. It's. just very particular arithmetic to understand those metrics.
Adel Nehme: you know, one of the last things you mentioned here is the not coming to the hype, right? And coming back to earth you know, when we're talking about hype, I think I'd be remiss not to talk about the generative AI elephant in the room as we talk about BizML you know, if we take a step back and take a bird's eye view, I think generative AI is going through the early machine learning 2010s moment where, self driving cars are starting to becoming a bit more of a reality, we find like more awe inspiring results, and I think that led to this gold rush approach of, fetishizing the science rather than the solutions.
Are you worried generative AI is going through the same motion as machine learning? And what do you think of are the dangers here of overhyping generative AI?
Eric Siegel: I think that's a great point to make that parallel between the sort of cycle that we're still suffering from with, you know, you might call it predictive AI rather than generative AI. What, same as what was how, has always been called predictive analytics. Either way it's machine learning, enterprise use cases that improve large scale operations.
So, yes, we're still suffering and that's what we've been talking about today. And the suffering around that generative AI hype is coming and I think very much there is a parallel and I would say that the generative AI hype is worse. So we're setting us, up for a more difficult shift and downfall.
I don't know when the next AI winner is coming. It could easily be five or eight years from now. It's definitely coming, but maybe more of just sort of a reckoning. Or certain reckonings are coming. The thing is that generative AI is amazing. And I was in a natural language processing research group for six years during my PhD before I was a professor at Columbia.
Both, I did them both at Columbia University. And I never thought I'd see what generative large language models can do in my lifetime. But! as excited and amazed as I am by it I'd say the world is about 10 times more amazed. And then of course, in my opinion, 10 times too much amazed. and maybe overvalued this by the same proportion, maybe more.
And what it comes down to is, The AGI hype, and I think that AGI is the modern day ghost story and that as human like as large language models are, and image generators are in a sense There's a big difference between what they do and what humans can do and we're not nearly it's not just that we're not close, it's that I think it's a mistake to consider what we're seeing now as a concrete step towards AGI.
And I think AGI is sort of the often unspoken, but the undercurrent of the hype. It's the sense of, hey, anything's possible, and we're definitely headed in that direction. And that's what's being promulgated a lot by these large language model companies to their own financial benefit. But I don't think that's good for anybody, including them.
It's gonna hurt.
Adel Nehme: It's interesting what you mention here. I agree with you. And you mentioned this concept of AGI in a lot of ways. The foundation model companies don't do themselves favors when they call, when they say that they're developing AGI. And you know, when you take, you know, we mentioned this parallel and we take the BizML paradigm as potentially a remedy to the the underlying problems with machine learning hype or traditional machine learning hype.
Do you imagine a similar playbook will, be needed to succeed with generative AI or do you think we'll be able to to leverage existing playbooks like the Bazelon playbooks to to speed up time to value with generative AI?
Eric Siegel: Now, that's a great question. And indeed, BizML, as formulated, is largely specific to predictive use cases. You know, established use cases are the ones that improve large scale operations. Although, generative, broadly speaking, it's very much the same kind of thing. You need to from the inception, exactly what operations or individual tasks by human are going to be changed in what way.
But I think the answer to your question is that it won't just be a one for generative. It can't be the same kind of one size fits all framework at least fully spanning because there's so many different ways we could be using a large language model or image generator. But for the most part let's be clear, models help people, they don't automate.
In fact, even though they're more human like. They, ironically, actually lend less potential autonomy because you need to supervise every output. You need to proofread everything it writes. You can't trust it, whereas the kinds of things you're doing with predictive AI, all the large scale operations we do as a business, they're wrong.
all, I'm sorry, they're wrong often or even most of the time for mass marketing or whatever the operation is. Now we're improving it, so it's less it's wrong less often. It's a significant improvement, it can be multiplier on the return on investment or the bottom line of the project. But there's that kind of leniency, there's that kind of forgiveness to those, the nature of those projects.
Therefore, you can, it can be autonomous. It automatically decides on the fly, instantly, whether to authorize your credit card charge based on a fraud detection model. That's autonomy, for example. You've got a million prospects, and you're deciding exactly which ones to include in your next direct mail.
Same thing, you've autonomously made those decisions. Even if you're physically licking each stamp manually, there's still autonomy in there. The same thing doesn't tend to apply for generative AI applications.
Adel Nehme: That's really great insight, Eric. So we are recording this on December 8th. And I think this episode is going to release early February, first week of February. what are trends that you see for AI in 2024?
Eric Siegel: Well, I think that there's going to be this strange rise of both disillusionment and hype. I mean, the AGI hype is not going to go away very soon, although by the time you're hearing this, I've hopefully published a new article on that so the reason I think that the hype's going to fade more slowly than might be ideal.
It's because generative AI is capable. First of all, it's the best damn demo ever of anything ever, period. I mean, I'm astounded by it. If I, didn't have so many job responsibilities and family responsibilities, all I would do is sit around playing with a language model. I think it's incredible. Um, and now that, those, that demo effect, and that isn't to say that the only value is the demo.
That's not what I mean. Because certainly, you know, helping a customer service agent on a chat, giving them a candidate paragraph of what they can then. manually review and potentially paste into the chat window with the customer. Things like that can have a real material impact on productivity. And people are starting to measure that now.
We're seeing that. That's great. So I'm not here to slam Gen AI. I'm simply here to say, hey, we need to temper the over, the expectation management, which is very poor at this point. And But the expectations are actually going to continue to be disproportionate because there's going to be new demos and there's going to be the, there's going to be generated video, you know, there's going to be all combinations.
There's so much data that we've generated as humans in our behavior by writing and filming videos and all this kind of stuff. so you can't reverse engineer the human mind just based on what we've written. As much as you can create astonishing results, but you can do some really amazing results, and the same will apply with video and the combination between the two.
So we're going to continue to see things that blow our socks off and are then leveraged to sort of tell the AGI narrative and continue the hype. So it's going to take a while But then at the same time, in parallel, people within the clear machine learning universe where they're actually deploying it at the large or medium sized organization to improve operations in a concrete way, that's where we have this issue of, hey it's not deploying as much as it could and should, and let's, Look at this very clearly.
I think that's where there's going to be a reckoning. So even if the broader world's continuing to ride the hype wave, the sort of inner world's going to go through some disillusionment and a correction. And I'm hoping that BizML will be there to help with that.
Adel Nehme: That is awesome, Eric. Eric, as we wrap up today's episode, do you have any final call to action or note to share with the audience?
Eric Siegel: go to bizml. com. If you're interested in the book, I hope you find it valuable. And I hope that like my first book, it gets concluded with a bunch of university. If you're a professor, I'm sure that we can get you an evaluation copy. Cause I'd love to see university courses that are covering this.
This is the thing that's missing. This is what we need to get machine learning successfully deployed.
Adel Nehme: Eric, it was great having you on DataFramed.
Eric Siegel: Great to be here, Adele. Thank you so much.
You’re invited! Join us for Radar: AI Edition
DataCamp Team
2 min
The Art of Prompt Engineering with Alex Banks, Founder and Educator, Sunday Signal
The Future of Programming with Kyle Daigle, COO at GitHub
ML Workflow Orchestration With Prefect
Serving an LLM Application as an API Endpoint using FastAPI in Python
How to Improve RAG Performance: 5 Key Techniques with Examples
Eugenia Anello