From BI to AI with Nick Magnuson, Head of AI at Qlik
Nick Magnuson is the Head of AI at Qlik, executing the organization’s AI strategy, solution development, and innovation. Prior to Qlik, Nick was the CEO of Big Squid, which was acquired by Qlik in 2021. Nick has previously held executive roles in customer success, product, and engineering in the field of machine learning and predictive analytics. As a practitioner in this field for over 20 years, Nick has published original research in these areas, as well as cognitive bias and other quantitative topics. He has also served as an advisor to other analytics platforms and start-ups. A long-time investment professional, Nick continues to hold his Chartered Financial Analyst designation and is a past member of the Chicago Quantitative Alliance and Society of Quantitative Analysts.
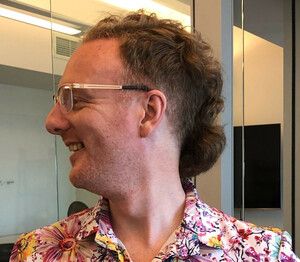
Richie helps individuals and organizations get better at using data and AI. He's been a data scientist since before it was called data science, and has written two books and created many DataCamp courses on the subject. He is a host of the DataFramed podcast, and runs DataCamp's webinar program.
Key Quotes
The intersection of structured and unstructured data, to me, is really interesting. We're doing some very, I think, innovative and interesting things in there to help try and exploit the opportunities that those two seemingly isolated sets of data can provide. Foundational models are at the heart of that. Generative AI is at the heart of that.
For me, AI is a portfolio play, 100%. And when I say portfolio play, I mean, it's you're not doing one or the other, you're doing both. Like the way to get scale out of AI is to have a portfolio solutions that you can leverage across different personas within your organization, across different contexts. And so it's a portfolio. So in-house use cases depending on the organization, depending on how sparse those resources are. Some organizations don't even have data science teams, and that's fine. That's just a recognition that you're going to be looking at other solutions. So in-house use cases, again, depending on the resource availability, my recommendation would be to focus on things that require a level of customization, a level of specificity in the inputs that only those very talented individuals would be able to affect. And then for use cases that are the very core to the business, so to speak, that require a level of precision in their implementation and where the risks are potentially quite large if you don't get it just right. That to me is like, you wanna be hands-on, you wanna be very, very specific in the delivery of that.
Key Takeaways
Prioritize the collection, standardization, and cleansing of a wide variety of data to fuel AI models, as data quality directly impacts the efficacy and reliability of AI outcomes.
Avoid reliance on a single AI technology or application; instead, deploy a diverse set of AI tools and strategies across different business functions to maximize benefits and mitigate risks.
Establish clear governance frameworks to ensure ethical use and security of AI technologies while empowering teams across the organization to innovate and apply AI to their specific areas of expertise.
Transcript
Richie Cotton: Welcome to DataFramed. This is Richie. Generative AI is invading everything, and I'm sure it's no surprise that that includes business intelligence platforms. Today we're going to discuss this marriage of AI and BI, and how you might make use of it at your organization. There are lots of subtleties in this, from how you develop an AI strategy, To how you deal with data quality and which use cases you ought to prioritize.
Today, we're going to figure out the answers to these problems. And our guest sits conveniently right at the intersection of AI and BI. Nick Magnusson is the head of AI at the BI company, Qlik. He was previously CEO at machine learning platform, Big Squid, and the founder of a quantitative investment firm.
Since we're combining both AI and BI in a two for one deal, I'm keen to get started and hear Nick's ideas.
Hi, Nick. Welcome to the show.
Nick Magnuson: Thanks, Richie.
Richie Cotton: So I'd love to dive straight in and talk about what's going on. Qlik. So I think QlikSense is perhaps your most famous product. This is your business intelligence platform. So, since there are a lot of BI platforms around at the moment. So can you tell me what's special about Sense?
Nick Magnuson: Yeah, Sense is sort of our core product. actually, Richie, this is the 30 year mark on Qlik's anniversary. So we're celebrating the long history of in the analytics and data space. Sense is you know, a product that enables people to get insight out of th... See more
So, that could be looking at very complex data across a variety of different sources that you need to bring together, need to combine it, need to be able to make sense of it, and then explore it through a visual means. And so Sense is sort of the core product that makes that process a lot easier enables a lot of other people that aren't really the sequel ninjas of the world to be able to do that.
And yeah, it's been a core product, for us since its uh, inception.
Richie Cotton: So it's really about the, the data analytics side of things, but Sense is part of the larger suite thing. So can you talk me through like what the whole suite involves?
Nick Magnuson: Yeah, certainly so, yeah, like Sensus, the, you know, the core analytics product today, building on QlikView, which many viewers and listeners may be more accustomed, that's the, the longer history product on the analytics side. But over the last decade or so, Qlik has made a, I think a, pretty distinct effort to try and expand that core offering into particularly into the data integration side of the data analytics workflow so that you can bring in sources from a variety of different both on prem and cloud and hybrid sources.
and do that at scale, So doing it with an enterprise grade data pipeline and capability to, to manage those workloads including things like ch change data capture so that you're incrementally updating that, that information and then building pipelines that can trans that can transform it into something that's usable.
Obviously that plays very well with the analytics side, where you're then taking that data and then making robust analysis and, and hopefully making decisions off of it. And then even more recently, we've added capabilities that are near and dear to my heart around machine learning and A.
I. As well as automations, the ability to take data as opposed to like read and infer it and then take an action and do that programmatically through through an automation. then, of course just this year we announced the acquisition of Talon. So Talon brings a very large portfolio of different products.
All the way from data integration into data, quality, stewardship, inventory and some robust capabilities around data prep. So at the end of the day, like the portfolio has expanded quite substantially and to an end to end platform that allows. Our customers to be empowered to make use of that data at any stage of that whole workflow.
So it's a pretty exciting product portfolio that we've now assembled to put together.
Richie Cotton: So this for every sort of stage of the data workflow, but that's kind of nice. We'll leave all this stuff on data preparation, the data engineering bits maybe for later. Let's, let's get into the juicy stuff I think with machine learning and AI. So I know you've just launched Stage. So that's your new AI product.
Come talk me through what the point of this is and how it fits into the rest of your AI tools.
Nick Magnuson: Yeah, stage is a, is an interesting announcement, right? I don't actually think it's a much of a product as it is a strategy. It's a strategy that helps our customers. Be successful in the implementation of their own AI. And for me, that comes down to three different pillars. The first of which is establishing a data foundation that you can leverage to build out AI models.
And that data foundation is, is fairly pivotal because the whole garbaging, garbage out is, no less true than it is with AI where You've got to have the highest level of data integrity so that you can trust the outputs that come from AI because the outputs from AI are dependent completely on the inputs.
So that data foundation is that first pillar to enable that success. The second piece is ensuring that we as an organization use AI to help our customers by infusing it into those capabilities. So as you're engaging in any part of that data analytics workflow, there's AI there to help make that process faster, easier, and more secure.
More efficient, et cetera. And then the last piece is more about recognizing that customers want to get hands on. They want to build their own AI solutions. They want to bring their data to that, and we need self service AI to help them support that. So we're building new products and we do have products today that help customers realize that objective.
So that stage in a nutshell, again, I think the idea is to help our customers get to successful implementation of AI. And that is a a multifaceted effort. Yeah,
Richie Cotton: I do think that's fascinating that you said that like getting success from AI requires you to have a strong data foundation to begin with. I'd love to get into that more in depth throughout this episode. Before we get to that I think, Certainly Datacamp has a lot of customers where they say, okay, we know we need to do something involving AI, but we're new to this.
We're not quite sure what to do. So can you perhaps talk about what some of the most common AI use cases are from Qlik customers?
Nick Magnuson: I can speak specifically on the, newer forms of AI with generative because I think that's where a lot of the focus is today. And, you know, I think one thing to recognize here is that everyone's exploring, like I talked to our global system integrators, our biggest partners across the globe, and they're doing tons of POCs, tons of POCs.
Very little is in production. It just gives you a sense of where people are at. They're trying to understand this technology. how do we use it? What kind of things can it solve for? And so there's a number of ways to look at that. I look at it from the perspective of, What can generative AI do? And I think there's three things that At least at this stage of the technology's evolution, it's very clear. They can do it can summarize very, very efficiently. It can create content, and some might argue not good content, but it can create content a very efficient manner.
And then the third way that I've seen it implemented that I think is worth noting is just around code generation or code interpretation. Now, those three things can be manifested in a lot of different ways. People look at chatbots. To me, chatbots are an implementation, not necessarily what the Gen AI is doing.
Gen AI is doing the summarization or content creation through that mechanism. But you know, that's sort of the primary viewpoint I take is what is it actually doing? And those three things are sort of the primary use cases that I've seen so far.
Richie Cotton: Are there any simple sort of high impact use cases you think are good as a first project for enterprises to start off with?
Nick Magnuson: Yeah, I love the what I would call internal use cases. So using generative AI within the organization, not as a customer facing or some sort of product offering. And the reason for that is, you know, if you think of the various functions you have within an organization, big or small, you've got sales professionals that are in the market trying to position service or your product, and they're doing that based on a knowledge of what your product does, how it operates.
You think of the people that are supporting customers again, they're, they're interacting with unstructured data to help them understand and how to communicate, troubleshoot with customers. so you basically go across the organization and almost every role is interacting with a ton of unstructured data.
Knowledge bases, et cetera, to help them do their job better to become experts in the, you know, the given role. And the process of like interacting with that unstructured data can be very tedious because you've got to go and find a document or you've got to go. Like the most common thing is someone pulls up MS Teams or Slack and says to their best friend, Hey, where can I find this information?
And that's not very efficient. So the use cases I like are, Hey, take all that documentation and put it into a, A reg architecture that an LLM can actually use so that when you have that question you can ask, you can ask a bot and it can go and reference that material and give you something of use.
Both for the summarization and the content generation. And I like that because, one, the value is clear. Like, you're just going to make your employees more efficient in what they're doing. So that's, fairly well established. Because they're, you know, there's documents that you already have.
Like, relative level of effort to bring those solutions to, to bear. it's not as onerous as it might seem so again I think you've got value and you've got relatively little little level of effort And then the third thing is if you don't get it perfect the first time, These aren't mission critical business functions that if it doesn't work like, you know, you're out of business This is you know stuff if you don't get it right on the first time there's low risk low consequence So I like those use cases.
I think they naturally then lead into more sophisticated use cases You But it's a good entree. It's a good way to get started.
Richie Cotton: I really like the idea of doing things internally just while you're trying to figure things out. Because You are, without doubt, going to have like your first attempt at some kind of bot is going to say something pretty stupid. Better to say that to an employee rather than one of your most important customers uh, definitely.
And in general, do you think that enterprises have different requirements around AI compared to individuals?
Nick Magnuson: Yes and no. I think there's commonality, like, whether I'm sharing my information with an LLM or I'm doing it on behalf of an organization, like, I don't want to share certain information. I don't want to share sensitive information, confidential information. I think the parallel there is, quite clear.
Now, on the other hand organizations have I think a higher burden on this because they're also working with their own end customers and by proxy of doing that they have Information about their customers that potentially, they're effectively the steward of that and to share that with an LLM, I think, creates a higher higher level of burden on the organization that does on the individual.
so I think that that may be the biggest difference is that organizations work on behalf of other customers, and so therefore they carry. Yeah, they carry a high burden on that.
Richie Cotton: Absolutely. So it seems like the big difference is really how much responsibility you have for things like data privacy, data security. So, do you have any advice on how you might go about dealing with that?
Nick Magnuson: Yeah, I mean, I think the biggest things there are putting in a policies and procedures around this. I think every organization at this stage of the game should have documented what their expectations are, both internally and then externally in terms of how they they use generative AI and what sort of conditions surround that around privacy and security.
For those that are looking for a bit of a, guideline on that, the, the OWASP just recently put out a I think a pretty good set of recommendations and vulnerabilities with our language models in particular, that for me would be the blueprint for any organization in terms of how you want to account for those vulnerabilities both from a security and privacy standpoint.
But at the end of the day, most of it comes down to, you can't share private information. And I think that's a, that's pretty common, pretty well understood. for organizations, you certainly don't want to be sharing confidential trade secret, even, you know, IP, like these large language models, They have a tough time unlearning what they've seen.
So, that is a, I think a key thing is you don't want to be sharing any of that. So there's a governance framework that needs to be in place to prevent that. And there's certain techniques you can use and way in which you interact with elements to make sure that that's a bit more secure.
Richie Cotton: just related to this, I think a lot of organizations must have dealt with a lot of these similar issues when they've started moving things into the cloud. So using SAS products, is it the same situation or do you think there are additional problems with large language models?
Nick Magnuson: I think there are additional conditions with large language models that are new to the same set of conditions that with going to the cloud or GDPR or, you know, your sovereignty. And that is that particularly the public LLMs, like, they're fairly black box in terms of, how they were constructed, what they were trained on and the inputs.
And so. if you were to push any of yours, like sensitive information in that, realistically, you don't know exactly how that's going to be used versus if it's being pushed into the cloud. Okay. Well, it's just, oh, it's in the cloud. I can, it's a little bit less transparent if you will, as to what the ramifications for any of that might be
Richie Cotton: Okay, and when you're not quite sure what's going on, you probably need to be a little bit more risk averse in that
Nick Magnuson: Yep, exactly. Yeah. I like to, I like to use the analogy that if you met someone for the first time. You're not going to divulge like all of your very secret information. You're probably going to talk about what the weather everyone talks about the weather, and that's because it's a fairly benign topic.
And I think the approach here could be very similar where, you're working with Janaya for the first time, you got to be very cautious about how you use it until you understand and start to build trust with the systems that that are built on top of this new technology.
Richie Cotton: Okay. Yes. It was like, don't give you a credit card number to someone you just met at the bus stop.
Nick Magnuson: Right.
Richie Cotton: Cool. So, before you were talking about data quality and the importance of having good data in order to get good results from AI. So I'd like to go back to that again. So it does feel a bit like, well, everyone's using the same foundational LLM.
So really the benefits you get, you need better data because everyone's using the same AI. So can you talk to me a bit about how you get higher data quality across your organization?
Nick Magnuson: data is paramount. It's, it's really critical and I think I have, if not a unique, at least a well founded perspective on this. the first 15 in my, 15 years of my career, I was an investor. Specifically I was a quant so a quantitative investor. I was using AI and ML long before data science was even a, term that we all now know.
And I went through the process of using the techniques to, like, find signal in the market and obviously try and take advantage of it. And to make a 15 year story short we went through a very discreet evolution where at first nobody was using it, nobody could trust it, nobody was using machine learning, and then people started using it, they started creating an advantage using it, and then everybody was using it.
And it became so ubiquitous that the math that supported the algorithmic trading and that sort of stuff was really not the differentiator. It became the data. And so organizations were investing heavily in trying to scrape together whatever different data they could so that they had some unique market advantage.
And I think the parallel is the same here. As businesses start to use AI, Very quickly, we will all be using the same foundational models, the same prompt tuning, Whatever the technique may be. And it'll actually come back down to what data do you have that's uniquely different than the competitor across the street.
So, in terms of data quality, there's a couple things that I think are super important. One is, AI loves the variety of data. Like, that's where it excels. It can see patterns that we can't. It can find those you know, those little tidbits of information that the human eye can never detect. So you need to bring a lot of different data, disparate data together and allow AI to kind of tackle it.
That means, like I said before, it could be on prem, could be hybrid, could be cloud. But organizations that are setting themselves up to collect as much data as possible, I think are going to be in an advantage. The second piece of it, of course, you can bring all that data together, but if it's, It's spotty, it's not cleansed, there's no quality, there's no, governance around it.
That can make it virtually useless. So, you've got to invest in data collection practices that make that data standardized and then therefore usable. Cleansing of that data should be standardized and where possible automated so that as the data comes in, it's in a form that people can use.
And then I think the other piece on top of it is transparency, it's because data will come in and there'll be transformations that'll be combined with other, as someone who's using it as a end user to actually build AI applications or AI models on top. You gotta have an understanding of where that data came from.
So lineage, transparency, auditability, those things are also very important because, You need to establish trust, and trust comes from an ability to understand, you know, and understand where that data came from. So, that's a lot, obviously, but that's actually where I think the money is made, is it investing in establishing that data foundation so that when you build AI, you can do it with conviction, you do it with confidence, it's trusted, well governed, etc.
Richie Cotton: You're right. That does sound like a lot. So you need lots of data, lots of different types of data, and you need to make sure it's all correct or suitable for use and well governed and all that kind of stuff. So because that's just a massive amount of tasks, I mean, it's probably an entire podcast episode in itself.
But can you just give me a quick overview of like, where do you get started with this? How do you just make those incremental improvements in data quality or data quantity from where you are now?
Nick Magnuson: Yeah, I think you, you pick out a specific use case. I, I love to start, with something small in scope and make sure that small scope has, you know, The right data, the right connectivity, the right data models that sit on top of it. And as you prove that out, then that becomes a launching point for further investment to make that make that data fabric, if you will of the same standard.
But, you know, if you try and boil the ocean, like, I mean, I went through that list fairly purposely because it is a big undertaking, but that doesn't mean that you can't start with a small slice of it and then through that success, employ that elsewhere.
Richie Cotton: Okay, yeah, so really just pick a use case and then go for it. And just as a bolt to that is there like one specific use case you think is a good place to start? know this is going to depend a lot on the business, but are there any sort of common themes here?
Nick Magnuson: I don't, necessarily think so. I think that's going to depend on, each organization. I certainly would advise, as we do with our customers, start with a use case that will drive some business value. But you already kind of understand that there is a level of quality to the data that's needed to support it.
Right, you don't want to go into a use case where, hey, this would be really cool, but I don't even know if we have the data. I don't even know if the data is, of requisite quality or depth or whatever. So, I think the marriage of a business case that, has some value, and it doesn't have to be like game changing, but it has to have value.
But certainly supported by at least superficially, you know that that data is there. we're in a unique position at Qlik because a lot of that data that we're talking about is data that's already been used for, standard purposes around visualization or reporting or stuff like that.
So it's largely cleansed, it's largely prepared, it's largely known. That's a pretty good starting point for a lot of organizations because You're not trying to go on and fetch new data that you've never seen before. It's data that you're already familiar with.
Richie Cotton: Okay. I mean, that does seem reasonable. It's just like, you know, think a bit about, yeah, business cases before you start diving off on on these new projects. All right. So, I'd like to talk a bit about how you go about using generative AI or AI more generally. So I know there's a lot of people going to, and sort of using these large language models sort of directly from the source.
But then there's also a lot of companies building AI into their products. I mean, we're doing this at data camp. I know you're doing it at Qlik and. A lot of other companies are doing this. So when do you want to use an LLM directly versus have it built into a product?
Nick Magnuson: Yeah, it's a good question. And I think that comes back again to the use case. So, if you're looking for, general responses, generic content, an out of the box solution can do the job. I use it, you know, fairly frequently. My kids use it quite frequently. Like, it's, you know, everybody has cracked open GPTN.
And that's part of the Part of the excitement is everyone knows like there's there's real implication real real opportunity with it if you go to chat GBT and ask a specific business question It's not grounded in your business. It doesn't know anything about your data So it's when you need to get specific that I think you need to work with LLMs directly and that's when all these privacy and security things really, really come into play.
But yeah, so it's, it's more around use cases if you're looking to build something that has, It would require specific knowledge that isn't generally part of the corpus that these models have been trained on. Then you've gotta go and work with with an LM directly and use techniques like RAG or pumped engineering or, what have you, to make sure that the, the model is grounded in the contextual information that makes the solution useful.
Richie Cotton: Yeah, absolutely. That sort of resonates a bit with my own experience. So if I'm trying to write some sort of marketing type copy, then throw it into chat GPT, that's fine. And it's marketing stuff. It's going to be public anyway, so no privacy worries. But then if I'm doing something technical, like I'm coding, I want a separate product. I don't want to necessarily do that within Chattopadhyay itself. And I guess more generally, how do you think about whether organizations should be buying existing AI services versus building
Nick Magnuson: For me, AI is a portfolio of play a hundred percent. Now, when I say portfolio of play, I mean it's. You're not doing one or the other you're doing both the way to get scale out of ai is to have a portfolio solutions that you can leverage across different personas within your organization, across different contexts.
And so it's, it's a portfolio. in house, you know, use cases depending on the organization, depending on how sparse those resources are. I mean, some organizations don't even have data science teams. And that's fine. That's just a recognition that you're going to be looking at other solutions.
So, in house use cases, depending on the resource availability, my recommendation would be to focus on things that require a level of customization. A level of specificity in the inputs that only like those very talented individuals would be able to affect.
And then, for use cases that are very they're very core to the business, so to speak that require a level of precision in their implementation. And where the risks are potentially quite large if you don't get it just right. That to me is like, you want to be, you want to be hands on, you want to be very specific in the delivery of that.
that's not to say that that's the only use case that can drive value. The other variety of use cases, and we have customers that are, deriving multi millions of dollars of savings or efficiency gains just through low hanging fruit use cases that you can get through built in technology that is catered to an analytics team, not a data science team or something like that.
So, I think really depends on the organization. It depends on the use case that you're going after. But I do believe that most organizations should be following a broader portfolio, like it shouldn't be, we're going to this part of the org for all our AI, because that does not scale, that's the one thing I've seen over the last 10 plus years now, that leads to failure.
Um, I have an interesting anecdote, I was talking to a manager at one of our customers the other day. Who was opining on a technology, an AI technology that they had just purchased. And one of his technical resources reported back to him that this AI technology could only do 85 percent of what he could do.
And the manager was like, that's pretty good. So his, his recommendation was, well, that's good. Cause now you can focus on the other 15 percent and make that, you know, even better. And I think that type of mentality is the right one where, hey, if we can use technology to be good. 80 percent better at everything else that we're doing.
That leaves us a lot of resource to focus on the things that, you know, we haven't yet perfected.
Richie Cotton: I like that he's only been outsourced 85%, so it was good. All right. So, does seem like, You need some kind of big AI strategy then just to make sure that all your portfolio comes together, especially if you're using external AI things, you've got some in house projects, a lot of different roles involved.
So first of all, like, where should this strategy come from? Like who should be responsible for it?
Nick Magnuson: I hate to put the burden on this individual, but for me, it's the CEO. It has to be the CEO. And it has to be the CEO because the possibility that you could build an AI strategy from the ground up grassroots style, I don't think is realistic, you'll get an isolated pocket of people doing something successful over here.
But they're not talking to people over here. And so like none of it will hang together at the end of the day. And it won't look like a strategy that. can really drive the level of value that you want out of it. So to me, it's the CEO to me It's it's a push down. It's a driven from the top, the strategy that i've seen that have been That have been successful like you can literally feel it permeate the organization across every level.
Everyone's aligned on it Everyone's excited by it because everyone wants to get involved in this. So, for me, goes from the top for better or worse.
Richie Cotton: And I suppose if your CEO is interested in AI, which hopefully you are, you're all right. If they're not, And you've got sort of access. Is how do you go about sort of persuading senior management or your CEO that they ought to get going with AI?
Nick Magnuson: It's a good question. Now, if you have a CEO that hasn't, caught onto this, then I think that it could be a challenge. You know, one of the things that. For me anyway, this is the second time I've seen this movie, right? I saw the auto ML, the automated machine learning kind of rise up and that took a long time because that technology was a little bit hard to understand, like you've got this data in these algorithms, like.
you needed like four different things. You needed understanding of the math, you needed the ability to code, you needed domain expertise, and you needed to be able to move the data around like a SQL ninja. And like, COs couldn't get their head around that, you and it built some critical mass over time.
Generative AI is different. everyone can crack open JAT GBT and go, Oh boy, you know, like this is a big deal. So I think for a large. These cross sectional CEOs, like, they're already on it because the boards are asking them about it at the end of the day. If you are one of those few that the CEO either hasn't got on board or isn't pushing that from a top down perspective, I think you've got to work on a proof of concept that the CEO can relate to so that they can understand, like, the implications of it and potentially position it as this is what everyone else is starting to do.
And if we're not on board, like we're starting to, put ourselves in a position where we're going to have to play catch up. You know, not to put the fear into the CEO, but that's, reality is everyone right now, like I said before, is trying to figure out how to use this technology and as soon as they do, it's going to create competitive advantage.
So, think it's incumbent upon people to push that into the CEO's lap and help them understand, how they could implement it, how they can take advantage of it.
Richie Cotton: that seems pretty sensible. And perhaps if the CEO is still resistant, then probably dust off your resume and look elsewhere. Okay. So, we've established the CEO has got to be leading the strategic efforts, cheerleading stuff. what else does the C suite need to do in order to make sure that your AI projects are successful?
Nick Magnuson: I think there's two things that I would point to one is at the C level, you've got to make sure that. Everyone else within the organization feels empowered to use this technology to apply to use cases that they're going to know very specifically because they're experts in that part of the organization or the domain but they need to feel empowered.
They need to have the technology. They need to have the resourcing to be able to do that. And then the second thing is, While they may feel empowered, they also can't go rogue and kind of use this without any guardrails. So the other piece that the C suite's got to push is the governance to ensure that, yes, you're empowered to use it, but there's guardrails and there's sort of, security and safety measures and protocols and, Making sure it's used for the right use cases that it's not built on bias or discriminatory data and those types of things.
So that goes back to a governance framework. And I think for most organizations, that governance framework should be fairly centralized. So that that is something that is everyone understands and is bought into. And then there permeates to all the applications across the business. But those two things, you know, in my view, the C suite's got to drive the empowerment and the governance of it.
Richie Cotton: Okay, it does seem to make sense if the governance is a whole company wide thing rather than each individual team having to reinvent governance every time, because you're going to have different rules, that's going to get complicated.
Nick Magnuson: Yeah, you'll have teams of legal professionals that you'll need to hire otherwise.
Richie Cotton: Yeah I guess, no, no one's had just lots of different lawyers for different AI projects. Okay. Uh, It does seem like a lot of different teams are going to have a stake in AI. So you're going to start off like the data engineers through to the analysts and yeah, all sorts of business and project stakeholders, whatever.
So, how can you manage all these different interests in AI across your organization?
Nick Magnuson: Yeah, it can be challenging, especially at scale. I think certain functions within, you know, we just talked about governance, certain functions within AI can be centralized or should at least be considered to be centralized, I should say. And that way, have accountability in a, single part of the organization.
Now, organizations should recognize that, you know, departments and products are how you organize hierarchically. they're going to want to be able to own and craft their own AI. They're going to want to be able to use it for the purposes that suit them best. And that's back to their empowerment. They should be empowered to do that because they're going to know what they need best, better than anyone else, and they're going to know how they want to implement it.
And so, I think in terms of, you know, we think of the governance part of it being centralized, the application of it being decentralized, At the end of the day, all that needs, I think at the end of the day, needs to be reported back up to that c-suite who's helping to drive it so that they can observe the type of ads being used, how well it's being used, what kind of implications is it causing, what, outcomes is it driving and then what sort of governance is in place around it so that they can manage it holistically.
But you, as you, as you just heard, like, I think a lot of it has to be decentralized in order to create that empowerment. While maintaining a central set of concerns around how it's being applied and what sort of guardrails are in place.
Richie Cotton: Yeah. I mean, I guess the thing is normally when you've got a cross functional team or cross functional project going on, then it's always like it's the other team's fault. But if you've got the C suite watching what's going on, then hopefully the different teams are going to behave and not squabble too much.
Nick Magnuson: Well, especially if it's being reported back and so the C suite can see, you know, there's a discrepancy between this team and this team and, there's a dependency here on the other or whatever, you know, you can detect that and hopefully alleviate it. I think again, If the separations of concerns can be done in such a way that the, departments or the products or however the organization, but they should be responsible for applying the AI within a construct that is centrally centrally governed.
Richie Cotton: All right. I'd like to switch a bit and talk about careers. So it just seemed like generative AI is having a huge impact on a lot of data careers. So. I guess, to me, how do you think that existing data roles like data analyst, data scientist, how are they going to change due to the rise of generative AI?
Nick Magnuson: Yeah, there, there's definitely some change in the works. The, one of the things I'll point to off the bat here is, with generative value, we're talking about moving from structured data to unstructured data. And unstructured data presents a lot of different data concerns that are slightly different in terms of their meaning and application with unstructured than with structured.
So, for instance, when we talk about Change data capture, you know, incremental changes in tabular data, pretty easy to understand when we're talking about Changes in data when it's in textual form. How do you manage that? That's a whole new concern How do you ensure data quality again? That's a whole new concern So I think that will evolve into Levels of expertise around working with unstructured data that we haven't seen in the past the other thing that I would point at is, by most accounts, the amount of unstructured data is at least twice as much, in some cases, nine times as large as the amount of structured data that we have.
And so organizations have been clamoring for years to try and make the most use of the structured data they have and probably only getting, you know, this much out of it. And now we have this whole pile of unstructured data. So there's a real big opportunity, a real big challenge on the other side of that same coin, but, bringing these worlds together is something unique.
I think from a career standpoint, you know, there'll be people that become more experts in working with structured data. if anyone listening here has worked with large language models and worked on prompting, it is super painful. it is a trick into its own trade. the prompts are super sensitive.
If you swap out the foundational model, the same prompts don't work the same way. So prompt engineers, there's already, you know, job listings for that. That's become a new thing. I think the role of the data scientist will evolve. still be data scientists who are in the, you know, in the data working, building solutions, certainly.
But I think a lot of data scientists will be looked at as more as a higher level concern, where they're not concerned about building solutions, but they're concerned about managing and governing solutions at scale. So that puts them into a a role where they're approving other work that's being done by a larger cross section of the organization.
Ensuring the quality of those models keeping it to a level of standard that the organization is set. think the data engineer probably moves into a more of a engineering type of role. Maybe they're prompt engineers, maybe they're the ones that are helping to build out those solutions.
And the other role I think probably changes over time is the data steward. You know, you've got this whole new world of unstructured data that they're going to be responsible for from a whole life cycle standpoint. so their, their job description will be rewritten if it hasn't already.
Richie Cotton: that's really interesting that like the sort of consistent theme throughout that is that data science isn't just about numbers anymore. It's really about all these unstructured data types of text and images and all that sort of stuff. And that actually reminds me, got asked a question recently that I wasn't quite sure how to answer.
So, lot of people, like traditionally you go, you go into data science, you've got a sort of STEM background, you know, you have science, technology, engineering, maths, and now if everything's going to a natural language interface, you need to be good at sentences as well. So does this mean that if you've got like an English degree background or some other sort of humanities, liberal arts sort of background, does that mean that you're then, that's now a viable pathway into data science?
Nick Magnuson: I think so. I have not heard that before, but that makes a lot of sense because we're talking about prose, right? We're talking about how things are worded. And the specificity of that wording has a lot of implication on how the LLM interprets it. And so, I could completely see the uh, Transcribed Ubiquitous liberal arts degree that no one thought was any value anymore being of a, intense value now, particularly because of the way in which these, these models are so sensitive to the way in which.
You express intent. If you express intent the right way, the models work. they can do a lot of really powerful things. But once you're off by just a little bit, or interpret it slightly differently, you're back to square one. So, I do actually think there's a role for that to come back into the technology spaces.
People that are very well versed on specific wording, word choice phraseology, those types of things, so that it communicates well to a AI, basically. You're not talking to a human anymore, you're talking to AI.
Richie Cotton: Definitely. Yeah. We've just upended the university system now, I think. Heh, heh, heh, heh. I suppose, well, I mean, related to that, I suppose philosophy degrees are now more important as well with everyone worrying about AI ethics. So, yeah, there's definitely a turnaround there. Alright, so, we've sidetracked a bit, but you were talking about how some of the existing roles will change.
So you mentioned data analysts, data scientists data stewards. Are there any new roles that you think will come about because of this?
Nick Magnuson: I think there'll be new roles I think there'll be new roles around developers and how they use large language models. I've already talked a little bit about the, the Prompt Engineer. To me, the Prompt Engineer is a byproduct of this new technology, just as, Ml ops engineers were a byproduct of automated machine learning when that came online however, I don't know that necessarily organizations are going to go out and hire net new talent for that what I do think is you're going to have a request or a requirement for People in tangential seats to start to move into that there.
I think that's the most logical means to fill those needs That being said there would be certain individuals and in programs that start to or already have built into this They're that this type of skill set into their programs and you'll be seeing those types of roles being filled by people who have kind of grown up with that as their banner to carry.
But I don't think you get the scale. So I think there's going to be a lot of transition as I was talking about of existing resources that can pick up this new load.
Richie Cotton: Okay. Yeah. So possibility of like some new roles is probably like not completely certain exactly what they're going to be. But we expect some, some new kinds of jobs going on. So I'd also like to ask about like the flip side to this is how can generative AI help people learn these data skills?
So I mean, at Datacamp, for example, we're big fans of Everyone being a little bit data literate. So how do you think generative AI can help?
Nick Magnuson: Well, yeah, it goes, back to some of those use cases I referred to as internal. Right, so if you're looking to reskill or upskill or, or just going to recraft your own skill set AI is a very efficient way to do that in my opinion we're frankly using it internally at Qlik to help some of our, our own initiatives because it's way more efficient to create the level of competency in a given area.
And so, I think generative AI has a role to play in that in terms of helping professionals become better equipped to handle this new reality. like I said, I encourage those internal use cases because I think there's very little downside to investing in those areas.
Richie Cotton: And for people who are interested in a career in AI, what skills should they be learning right now?
Nick Magnuson: Because this is all relatively new, especially degenerative AI stuff, We're just about a year into when ChatGBT was, was released. So, again, I think everyone's playing a little bit of. Catch up is the right word, but everyone's catching up. So I don't really know that that's the right term to use, but everyone's trying to figure it out.
And if you're looking for a career in this area, I mean, I would, I would get in and start learning everything you can about a large language models, the different techniques around them, the different types of models, what's good about each, what's not so good about each. How do you work with small models versus large models?
What is, what is a small model these days? That type of expertise, I think, is, going to be useful for almost every organization. And so, I would start there. that'll bring about a level of competency in an area that very few have it today. Which will make yourself valuable, make yourself useful.
Now, I think over time, there'll be technologies that come online that continue as they always do, lower the barrier, make it easier for the average Joe to come in and get value out of working with the foundational model. So at that point, I don't know that that expertise becomes incrementally as valuable as it is today.
However, at that point, it'll be more about people who can, that can see an opportunity, that can see how a generative AI solution can transform a particular part of the business And they can connect all those dots to make it happen. That's a skill set that frankly it just takes time to build.
It takes time to build experiences, the, the core set there.
Richie Cotton: Okay. So there's really like a lot to go on, like it's some technical skills and then also like understanding how things are joined together as that requires your business skills as well. So yeah pretty broad skillset. There's probably a lot of different ways into this from different directions, I think.
Nick Magnuson: Yeah, I would, I would agree with that too.
Richie Cotton: All right. Before we wrap up I'd like to know, what are you working on that Qlik that you're excited about at the moment?
Nick Magnuson: Yeah, well, just about everything we talked about you know, the, Qlik is in a unique position, right? We've got a strong product line around data, a strong product line around analytics, and when you think about the application of AI, you need both the data and then you need the ability to action anything that comes out of data.
Yeah. So I'm excited by that because we're in a unique position to help our customers in the market in general make use of this technology. the intersection of structured and unstructured data to me is, really interesting. And we're doing some very, I think, innovative and interesting things in there to help train.
exploit the opportunities that those two seemingly isolated sets of data can provide. And foundational models are at the heart of that generative bias at the heart of that. So, I'm particularly excited about all that stuff because I think it can be transformative.
And I think Qlik is in a position that uniquely can help customers avail themselves of that data in a way in which they've, never been able to explore before. So I think At the day, we're at the very tip of that iceberg. And I think there's a lot of really interesting things to come from it.
Richie Cotton: Absolutely. I definitely agree. It's exciting times, lots more interesting stuff to come. So do you have any final advice for any organizations wanting to adopt AI?
Nick Magnuson: Yeah, I do. I think, you know, we talk a lot about generative AI in this, forum here. AI is like the topic du jour and has been for a good period of time here. And that's great. Cause it, it is transformative, but I think as I indicated, a lot of people are figuring out how to use it.
While you have all this other AI that no one's really talking about traditionally, AI that's been around for a long time that, works with structured data, supervised learning, predictive modeling, that stuff has immense value and you can make use of it today, those use cases are better established.
Uh, You've got a lot of documentation on it. So one thing I would encourage is, all AI matters. That's one of the things we like to say at Qlik is all AI matters and generative AI has brought up the, level of the conversation on AI. But in some cases, it's kind of blinded us to the other applications of it.
So, I would encourage organizations to think about AI holistically, not just with regard to generative. In fact, some people now, I catch them using AI when they really mean generative AI. And I have to remind them, like, there's this whole other class of AI that we, we know we can use and can drive value with.
So, the one message I would leave given the context of this, this discussion is, don't forget about the other AI, the traditional AI.
Richie Cotton: remember, please remember logistic regression. It's still quite useful. I
Nick Magnuson: Yeah.
Richie Cotton: it. All right. Thank you very much, Nick, for your time. That was really informative.
Nick Magnuson: Yeah. I appreciate it, Richie. Thank you.
You’re invited! Join us for Radar: AI Edition
DataCamp Team
2 min
The Art of Prompt Engineering with Alex Banks, Founder and Educator, Sunday Signal
The Future of Programming with Kyle Daigle, COO at GitHub
A Comprehensive Guide to Working with the Mistral Large Model
Josep Ferrer
12 min
Serving an LLM Application as an API Endpoint using FastAPI in Python
How to Improve RAG Performance: 5 Key Techniques with Examples
Eugenia Anello