HR Analytics: Predicting Employee Churn in Python
In this course you'll learn how to apply machine learning in the HR domain.
Commencer Le Cours Gratuitement4 heures14 vidéos44 exercices8 195 apprenantsDéclaration de réalisation
Créez votre compte gratuit
ou
En continuant, vous acceptez nos Conditions d'utilisation, notre Politique de confidentialité et le fait que vos données sont stockées aux États-Unis.Formation de 2 personnes ou plus ?
Essayer DataCamp for BusinessApprécié par les apprenants de milliers d'entreprises
Description du cours
Among all of the business domains, HR is still the least disrupted. However, the latest developments in data collection and analysis tools and technologies allow for data driven decision-making in all dimensions, including HR. This course will provide a solid basis for dealing with employee data and developing a predictive model to analyze employee turnover.
Formation de 2 personnes ou plus ?
Donnez à votre équipe l’accès à la plateforme DataCamp complète, y compris toutes les fonctionnalités.- 1
Introduction to HR Analytics
GratuitIn this chapter you will learn about the problems addressed by HR analytics, as well as will explore a sample HR dataset that will further be analyzed. You will describe and visualize some of the key variables, transform and manipulate the dataset to make it ready for analytics.
- 2
Predicting employee turnover
This chapter introduces one of the most popular classification techniques: the Decision Tree. You will use it to develop an algorithm that predicts employee turnover.
Splitting the data50 xpSeparating Target and Features100 xpSpliting employee data100 xpIntroduction to Decision Tree classification50 xpComputing Gini index100 xpSplitting the tree100 xpPredicting employee churn using decision trees50 xpFitting the tree to employee data100 xpChecking the accuracy of prediction100 xpInterpretation of the decision tree50 xpExporting the tree100 xpInterpretation of results50 xp - 3
Evaluating the turnover prediction model
Here, you will learn how to evaluate a model and understand how "good" it is. You will compare different trees to choose the best among them.
Tuning employee turnover classifier50 xpPruning the tree100 xpLimiting the sample size100 xpEvaluating the model50 xpInterpreting accuracy metrics50 xpCalculating accuracy metrics: precision100 xpCalculating accuracy metrics: recall100 xpTargeting both leavers and stayers50 xpCalculating the ROC/AUC score100 xpClass imbalance50 xpBalancing classes100 xpComparison of Employee attrition models100 xp - 4
Choosing the best turnover prediction model
In this final chapter, you will learn how to use cross-validation to avoid overfitting the training data. You will also learn how to know which features are impactful, and which are negligible. Finally, you will use these newly acquired skills to build a better performing Decision Tree!
Hyperparameter tuning50 xpCross-validation using sklearn100 xpSetting up GridSearch parameters100 xpImplementing GridSearch100 xpImportant features for predicting attrition50 xpInterpreting importance50 xpSorting important features100 xpSelecting important features100 xpDevelop and test the best model100 xpFinal thoughts50 xp
Formation de 2 personnes ou plus ?
Donnez à votre équipe l’accès à la plateforme DataCamp complète, y compris toutes les fonctionnalités.ensembles de données
Employee turnover datacollaborateurs
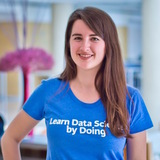
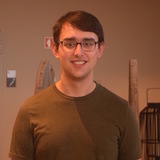
prérequis
Intermediate PythonQu’est-ce que les autres apprenants ont à dire ?
Inscrivez-vous 15 millions d’apprenants et commencer HR Analytics: Predicting Employee Churn in Python Aujourd’hui!
Créez votre compte gratuit
ou
En continuant, vous acceptez nos Conditions d'utilisation, notre Politique de confidentialité et le fait que vos données sont stockées aux États-Unis.