Performing Experiments in Python
Learn about experimental design, and how to explore your data to ask and answer meaningful questions.
Commencer Le Cours Gratuitement4 heures16 vidéos53 exercices7 717 apprenantsDéclaration de réalisation
Créez votre compte gratuit
ou
En continuant, vous acceptez nos Conditions d'utilisation, notre Politique de confidentialité et le fait que vos données sont stockées aux États-Unis.Formation de 2 personnes ou plus ?
Essayer DataCamp for BusinessApprécié par les apprenants de milliers d'entreprises
Description du cours
Data is all around us and can help us to understand many things. Making a pretty graph is great, but how can we tell the difference between a few outliers on a graph and a real, reliable effect? Is a trend that we see on a graph a reliable result or just random chance playing tricks? In this course, you will learn how to interrogate datasets in a rigorous way, giving clear answers to your questions. You will learn a range of statistical tests, how to apply them, how to understand their results, and how to deal with their shortcomings. Along the way, you will explore Olympic athlete data and the differences between populations of continents.
Formation de 2 personnes ou plus ?
Donnez à votre équipe l’accès à la plateforme DataCamp complète, y compris toutes les fonctionnalités.- 1
The Basics of Statistical Hypothesis Testing
GratuitIn this chapter, you will learn how to explore your data and ask meaningful questions. Then, you will discover how to answer these question by using your first statistical hypothesis tests: the t-test, the Chi-Square test, the Fisher exact test, and the Pearson correlation test.
- 2
Design Considerations in Experimental Design
In this chapter, you will learn how to examine and multiple factors at once, controlling for the effect of confounding variables and examining interactions between variables. You will learn how to use randomization and blocking to build robust tests and how to use the powerful ANOVA method.
Confounding variables50 xpExploring confounding variables100 xpFinding confounding variables100 xpBlocking and randomization50 xpRandom sampling100 xpBlocking100 xpPaired t-test100 xpANOVA50 xpOne-way ANOVA100 xpTwo-way ANOVA100 xpInteractive effects50 xpTwo-way ANOVA with interactive effects100 xpChoosing an appropriate test100 xp - 3
Sample size, Power analysis, and Effect size
In this chapter, you will focus on ways to avoid drawing false conclusions, whether false positives (type I errors) or false negatives (type II errors). Central to avoiding false negatives is understanding the interplay between sample size, power analysis, and effect size.
Type I errors50 xpBonferroni correction100 xpŠídák correction100 xpSample size50 xpExploring sample size100 xpSample size for a t-test100 xpEffect size50 xpEffect size for a t-test100 xpComputing Cohen's d100 xpEffect size for a Fisher exact test100 xpEffect sizes for Pearson correlation100 xpPower50 xpPower analysis for a t-test100 xpInterpreting power analyses50 xp - 4
Testing Normality: Parametric and Non-parametric Tests
In this final chapter, you will examine the assumptions underlying statistical tests and learn about how that influences your experimental design. This will include learning whether a variable follows a normal distribution and when you should use non-parametric statistical tests like the Wilcoxon rank-sum test and the Spearman correlation test.
Assumptions and normal distributions50 xpExploring distributions with summary stats100 xpQ-Q plot100 xpTesting for normality50 xpShapiro-Wilk test100 xpChoosing tests and normality50 xpNon-parametric tests: Wilcoxon rank-sum test50 xpWilcoxon rank-sum test100 xpWilcoxon signed-rank test100 xpParametric vs non-parametric tests100 xpMore non-parametric tests: Spearman correlation50 xpSpearman correlation100 xpChoosing the correct correlation test100 xpSummary50 xp
Formation de 2 personnes ou plus ?
Donnez à votre équipe l’accès à la plateforme DataCamp complète, y compris toutes les fonctionnalités.collaborateurs
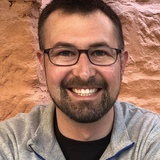
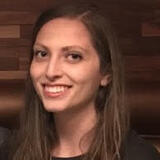
prérequis
Foundations of Probability in PythonQu’est-ce que les autres apprenants ont à dire ?
Inscrivez-vous 15 millions d’apprenants et commencer Performing Experiments in Python Aujourd’hui!
Créez votre compte gratuit
ou
En continuant, vous acceptez nos Conditions d'utilisation, notre Politique de confidentialité et le fait que vos données sont stockées aux États-Unis.