Winning a Kaggle Competition in Python
Learn how to approach and win competitions on Kaggle.
Commencer Le Cours Gratuitement4 heures16 vidéos52 exercices18 454 apprenantsDéclaration de réalisation
Créez votre compte gratuit
ou
En continuant, vous acceptez nos Conditions d'utilisation, notre Politique de confidentialité et le fait que vos données sont stockées aux États-Unis.Formation de 2 personnes ou plus ?
Essayer DataCamp for BusinessApprécié par les apprenants de milliers d'entreprises
Description du cours
Kaggle is the most famous platform for Data Science competitions. Taking part in such competitions allows you to work with real-world datasets, explore various machine learning problems, compete with other participants and, finally, get invaluable hands-on experience. In this course, you will learn how to approach and structure any Data Science competition. You will be able to select the correct local validation scheme and to avoid overfitting. Moreover, you will master advanced feature engineering together with model ensembling approaches. All these techniques will be practiced on Kaggle competitions datasets.
Formation de 2 personnes ou plus ?
Donnez à votre équipe l’accès à la plateforme DataCamp complète, y compris toutes les fonctionnalités.Dans les titres suivants
Scientifique en apprentissage automatique en Python
Aller à la piste- 1
Kaggle competitions process
GratuitIn this first chapter, you will get exposure to the Kaggle competition process. You will train a model and prepare a csv file ready for submission. You will learn the difference between Public and Private test splits, and how to prevent overfitting.
Competitions overview50 xpExplore train data100 xpExplore test data100 xpPrepare your first submission50 xpDetermine a problem type50 xpTrain a simple model100 xpPrepare a submission100 xpPublic vs Private leaderboard50 xpWhat model is overfitting?50 xpTrain XGBoost models100 xpExplore overfitting XGBoost100 xp - 2
Dive into the Competition
Now that you know the basics of Kaggle competitions, you will learn how to study the specific problem at hand. You will practice EDA and get to establish correct local validation strategies. You will also learn about data leakage.
Understand the problem50 xpUnderstand the problem type50 xpDefine a competition metric100 xpInitial EDA50 xpEDA statistics100 xpEDA plots I100 xpEDA plots II100 xpLocal validation50 xpK-fold cross-validation100 xpStratified K-fold100 xpValidation usage50 xpTime K-fold100 xpOverall validation score100 xp - 3
Feature Engineering
You will now get exposure to different types of features. You will modify existing features and create new ones. Also, you will treat the missing data accordingly.
Feature engineering50 xpArithmetical features100 xpDate features100 xpCategorical features50 xpLabel encoding100 xpOne-Hot encoding100 xpTarget encoding50 xpMean target encoding100 xpK-fold cross-validation100 xpBeyond binary classification100 xpMissing data50 xpFind missing data100 xpImpute missing data100 xp - 4
Modeling
Time to bring everything together and build some models! In this last chapter, you will build a base model before tuning some hyperparameters and improving your results with ensembles. You will then get some final tips and tricks to help you compete more efficiently.
Baseline model50 xpReplicate validation score100 xpBaseline based on the date100 xpBaseline based on the gradient boosting100 xpHyperparameter tuning50 xpGrid search100 xp2D grid search100 xpModel ensembling50 xpModel blending100 xpModel stacking I100 xpModel stacking II100 xpFinal tips50 xpTesting Kaggle forum ideas100 xpSelect final submissions50 xpFinal thoughts50 xp
Formation de 2 personnes ou plus ?
Donnez à votre équipe l’accès à la plateforme DataCamp complète, y compris toutes les fonctionnalités.Dans les titres suivants
Scientifique en apprentissage automatique en Python
Aller à la pisteensembles de données
Demand forecasting (train)Demand forecasting (test)House prices (train)House prices (test)Taxi rides (train)Taxi rides (test)collaborateurs
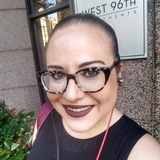
Qu’est-ce que les autres apprenants ont à dire ?
Inscrivez-vous 15 millions d’apprenants et commencer Winning a Kaggle Competition in Python Aujourd’hui!
Créez votre compte gratuit
ou
En continuant, vous acceptez nos Conditions d'utilisation, notre Politique de confidentialité et le fait que vos données sont stockées aux États-Unis.