Dimensionality Reduction in R
Learn dimensionality reduction techniques in R and master feature selection and extraction for your own data and models.
Kurs Kostenlos Starten4 Stunden16 Videos56 Übungen
Kostenloses Konto erstellen
oder
Durch Klick auf die Schaltfläche akzeptierst du unsere Nutzungsbedingungen, unsere Datenschutzrichtlinie und die Speicherung deiner Daten in den USA.Trainierst du 2 oder mehr?
Versuchen DataCamp for BusinessBeliebt bei Lernenden in Tausenden Unternehmen
Kursbeschreibung
Do you ever work with datasets with an overwhelming number of features? Do you need all those features? Which ones are the most important? In this course, you will learn dimensionality reduction techniques that will help you simplify your data and the models that you build with your data while maintaining the information in the original data and good predictive performance.
We live in the information age—an era of information overload. The art of extracting essential information from data is a marketable skill. Models train faster on reduced data. In production, smaller models mean faster response time. Perhaps most important, smaller data and models are often easier to understand. Dimensionality reduction is your Occam’s razor in data science.
The difference between feature selection and feature extraction! Using R, you will learn how to identify and remove features with low or redundant information, keeping the features with the most information. That’s feature selection. You will also learn how to extract combinations of features as condensed components that contain maximal information. That’s feature extraction!
But most importantly, using R’s new tidymodel package, you will use real-world data to build models with fewer features without sacrificing significant performance.
Why learn dimensionality reduction?
We live in the information age—an era of information overload. The art of extracting essential information from data is a marketable skill. Models train faster on reduced data. In production, smaller models mean faster response time. Perhaps most important, smaller data and models are often easier to understand. Dimensionality reduction is your Occam’s razor in data science.
What will you learn in this course?
The difference between feature selection and feature extraction! Using R, you will learn how to identify and remove features with low or redundant information, keeping the features with the most information. That’s feature selection. You will also learn how to extract combinations of features as condensed components that contain maximal information. That’s feature extraction!
But most importantly, using R’s new tidymodel package, you will use real-world data to build models with fewer features without sacrificing significant performance.
Trainierst du 2 oder mehr?
Verschaffen Sie Ihrem Team Zugriff auf die vollständige DataCamp-Plattform, einschließlich aller Funktionen.In den folgenden Tracks
Machine Learning Scientist mit R
Gehe zu Track- 1
Foundations of Dimensionality Reduction
KostenlosPrepare to simplify large data sets! You will learn about information, how to assess feature importance, and practice identifying low-information features. By the end of the chapter, you will understand the difference between feature selection and feature extraction—the two approaches to dimensionality reduction.
Introduction to dimensionality reduction50 xpDimensionality and feature information100 xpMutual information features100 xpInformation and feature importance50 xpCalculating root entropy100 xpCalculating child entropies100 xpCalculating information gain of color100 xpThe Importance of Dimensionality Reduction in Data and Model Building50 xpCalculate possible combinations100 xpCurse of dimensionality, overfitting, and bias100 xp - 2
Feature Selection for Feature Importance
Learn how to identify information-rich and information-poor features missing value ratios, variance, and correlation. Then you'll discover how to build tidymodel recipes to select features using these information indicators.
Feature selection vs. feature extraction50 xpCreate a zero-variance filter100 xpCreate a missing values filter100 xpFeature selection with the combined filter100 xpSelecting based on missing values50 xpCreate a missing value ratio filter100 xpApply a missing value ratio filter100 xpCreate a missing values recipe100 xpSelecting based on variance50 xpCreate a low-variance filter100 xpCreate a low-variance recipe100 xpSelecting based on correlation with other features50 xpIdentify highly correlated features100 xpSelect correlated feature to remove50 xpCreate a high-correlation recipe100 xp - 3
Feature Selection for Model Performance
Chapter three introduces the difference between unsupervised and supervised feature selection approaches. You'll review how to use tidymodels workflows to build models. Then, you'll perform supervised feature selection using lasso regression and random forest models.
Supervised feature selection50 xpSupervised vs. unsupervised feature selection100 xpDecision tree feature selection type50 xpModel Building and Evaluation with tidymodels50 xpSplit out the train and test sets100 xpCreate a recipe-model workflow100 xpFit, explore, and evaluate the model100 xpLasso Regression50 xpScale the data for lasso regression100 xpExplore lasso regression penalty values100 xpTune the penalty hyperparameter100 xpFit the best model100 xpRandom forest models50 xpCreate full random forest model100 xpReduce data using feature importances100 xpCreate reduced random forest100 xp - 4
Feature Extraction and Model Performance
In this final chapter, you'll gain a strong intuition of feature extraction by understanding how principal components extract and combine the most important information from different features. Then learn about and apply three types of feature extraction — principal component analysis (PCA), t-SNE, and UMAP. Discover how you can use these feature extraction methods as a preprocessing step in the tidymodels model-building process.
Foundations of feature extraction - principal components50 xpUnderstanding principal components100 xpNaming principal components50 xpPrincipal Component Analysis (PCA)50 xpPCA: variance explained50 xpMapping features to principal components100 xpPCA in tidymodels100 xpt-Distributed Stochastic Neighborhood Embedding (t-SNE)50 xpSeparating house prices with PCA100 xpSeparating house prices with t-SNE100 xpUniform Manifold Approximation and Projection (UMAP)50 xpSeparating house prices with UMAP100 xpUMAP reduction in a decision tree model100 xpEvaluate the UMAP decision tree model100 xpWrap up50 xp
Trainierst du 2 oder mehr?
Verschaffen Sie Ihrem Team Zugriff auf die vollständige DataCamp-Plattform, einschließlich aller Funktionen.In den folgenden Tracks
Machine Learning Scientist mit R
Gehe zu TrackMitwirkende
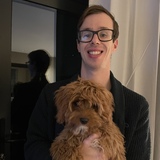
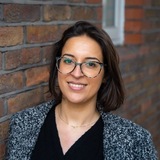
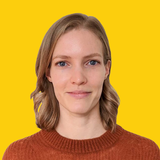
Voraussetzungen
Modeling with tidymodels in RWas sagen andere Lernende?
Melden Sie sich an 15 Millionen Lernende und starten Sie Dimensionality Reduction in R Heute!
Kostenloses Konto erstellen
oder
Durch Klick auf die Schaltfläche akzeptierst du unsere Nutzungsbedingungen, unsere Datenschutzrichtlinie und die Speicherung deiner Daten in den USA.