Financial Forecasting in Python
Step into the role of CFO and learn how to advise a board of directors on key metrics while building a financial forecast.
Comece O Curso Gratuitamente4 horas12 vídeos49 exercícios
Crie sua conta gratuita
ou
Ao continuar, você aceita nossos Termos de Uso, nossa Política de Privacidade e que seus dados são armazenados nos EUA.Treinar 2 ou mais pessoas?Experimente o DataCamp For Business
Amado por alunos de milhares de empresas
Descrição do Curso
In Financial Forecasting in Python, you will step into the role of CFO and learn how to advise a board of directors on key metrics while building a financial forecast, the basics of income statements and balance sheets, and cleaning messy financial data. During the course, you will examine real-life datasets from Netflix, Tesla, and Ford, using the pandas package. Following the course, you will be able to calculate financial metrics, work with assumptions and variances, and build your own forecast in Python!
Para Empresas
Treinar 2 ou mais pessoas?
Obtenha acesso à biblioteca completa do DataCamp, com relatórios, atribuições, projetos e muito mais centralizados- 1
Income statements
GratuitoIn this chapter, we will learn the basics of financial statements, with a specific focus on the income statement, which provides details on our sales, costs, and profits. We will learn how to calculate profitability metrics and finish off what we have learned by building our profit forecast for Tesla!
Introduction to financial statements50 xpCalculating gross profit100 xpCalculating net profit100 xpElements within net profit & gross profit50 xpCalculating sales & Cost of Goods Sold (COGS)50 xpCalculating sales100 xpForecasting sales with a discount100 xpCalculating COGS100 xpCalculating the break-even point100 xpWorking with raw forecast datasets50 xpTesla income statement100 xpForecasting profit for Tesla100 xp - 2
Balance sheet and forecast ratios
In this chapter, we will learn a bit more about the balance sheet, covering assets and liabilities and specific ratios to help evaluate the financial health and efficiency of a company, as well as how these ratios can assist us in building a great forecast.
Introduction to the balance sheet50 xpCalculating accounts receivable (debtors)100 xpBad debts100 xpCalculating accounts payable (creditors)100 xpUnderstanding accounts payable and receivable50 xpBalance sheet efficiency ratios - Part 150 xpDebtor days ratio100 xpDays payable outstanding100 xpBalance sheet efficiency ratios - Part 250 xpDays in inventory and asset turnover ratio100 xpUnderstanding ratios50 xpCalculating balance sheet ratios for Ford100 xpForecasting the balance sheet for Ford100 xp - 3
Formatting raw data, managing dates and financial periods
We have gotten a basic understanding of income statements and balance sheets. However, consolidating data for forecasting is complex, so in this chapter, we will look at some basic tools to help solve some of the complexities specifically relating to finance - working with dates and different financial periods, and formatting our raw data into the correct format for financial forecasting.
Financial periods and how to work with them50 xpConverting quarters into months100 xpMerging months into quarters100 xpThe datetime library50 xpWorking with the datetime library50 xpConverting date formats - simple100 xpConverting date formats - explicit100 xpTips and tricks when working with datasets50 xpWorking with datasets - month totals100 xpWorking with datasets - combining datasets100 xpWorking with datasets - exporting data50 xp - 4
Assumptions and variances in forecasts
In this chapter, we will be exploring two more aspects to creating a good forecast. First, we will look at assumptions, what drives them and what happens when an assumption changes? Next, we will look at variances, as a forecast is built at one point in time, but what happens when the actual results do not correspond to our forecast? We need to build a sensitive forecast that can be sensitive to changes in both assumptions and take into account variances, and this is what we will explore in this chapter.
Building sensitive forecast models50 xpWeighted probability100 xpMarket sentiment100 xpDependencies and sensitivity50 xpAssigning dependencies for sales and COGS100 xpBuilding a sensitivity analysis for gross profit100 xpAssigning dependencies for expenses100 xpBuild a sensitivity analysis for the net profit100 xpWorking with variances in the forecast50 xpBuilding an alternate forecast100 xpBuilding a gap analysis between forecasts100 xpSetting dependencies for Netflix100 xpCalculating an alternative forecast for Netflix100 xp
Para Empresas
Treinar 2 ou mais pessoas?
Obtenha acesso à biblioteca completa do DataCamp, com relatórios, atribuições, projetos e muito mais centralizadoscolaboradores
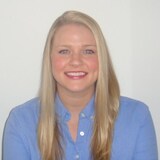
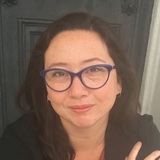
Victoria Clark
Ver MaisChartered Global Management Accountant at CIMA
O que os outros alunos têm a dizer?
Junte-se a mais de 14 milhões de alunos e comece Financial Forecasting in Python hoje mesmo!
Crie sua conta gratuita
ou
Ao continuar, você aceita nossos Termos de Uso, nossa Política de Privacidade e que seus dados são armazenados nos EUA.