Introduction to Anomaly Detection in R
Learn statistical tests for identifying outliers and how to use sophisticated anomaly scoring algorithms.
Comece O Curso Gratuitamente4 horas13 vídeos47 exercícios6.956 aprendizesDeclaração de Realização
Crie sua conta gratuita
ou
Ao continuar, você aceita nossos Termos de Uso, nossa Política de Privacidade e que seus dados são armazenados nos EUA.Treinar 2 ou mais pessoas?
Tentar DataCamp for BusinessAmado por alunos de milhares de empresas
Descrição do Curso
Are you concerned about inaccurate or suspicious records in your data, but not sure where to start? An anomaly detection algorithm could help! Anomaly detection is a collection of techniques designed to identify unusual data points, and are crucial for detecting fraud and for protecting computer networks from malicious activity. In this course, you'll explore statistical tests for identifying outliers, and learn to use sophisticated anomaly scoring algorithms like the local outlier factor and isolation forest. You'll apply anomaly detection algorithms to identify unusual wines in the UCI Wine quality dataset and also to detect cases of thyroid disease from abnormal hormone measurements.
Para Empresas
Treinar 2 ou mais pessoas?
Obtenha acesso à biblioteca completa do DataCamp, com relatórios, atribuições, projetos e muito mais centralizados- 1
Statistical outlier detection
GratuitoIn this chapter, you'll learn how numerical and graphical summaries can be used to informally assess whether data contain unusual points. You'll use a statistical procedure called Grubbs' test to check whether a point is an outlier, and learn about the Seasonal-Hybrid ESD algorithm, which can help identify outliers when the data are a time series.
What do we mean when we talk about anomalies?50 xpRecognizing anomaly types50 xpExploring the river nitrate data100 xpTesting the extremes with Grubbs' test50 xpVisual check of normality100 xpGrubbs' test100 xpHunting multiple outliers using Grubbs' test100 xpAnomalies in time series50 xpVisual assessment of seasonality100 xpSeasonal Hybrid ESD algorithm100 xpInterpreting Seasonal-Hybrid ESD output100 xpSeasonal-Hybrid ESD versus Grubbs' test50 xp - 2
Distance and density based anomaly detection
In this chapter, you'll learn how to calculate the k-nearest neighbors distance and the local outlier factor, which are used to construct continuous anomaly scores for each data point when the data have multiple features. You'll learn the difference between local and global anomalies and how the two algorithms can help in each case.
k-nearest neighbors distance score50 xpExploring wine100 xpkNN distance matrix100 xpkNN distance score100 xpVisualizing kNN distance50 xpStandardizing features100 xpAppending the kNN score100 xpVisualizing kNN distance score100 xpLocal outlier factor50 xpLOF calculation100 xpLOF visualization100 xpLOF vs kNN100 xp - 3
Isolation forest
k-nearest neighbors distance and local outlier factor use the distance or relative density of the nearest neighbors to score each point. In this chapter, you'll explore an alternative tree-based approach called an isolation forest, which is a fast and robust method of detecting anomalies that measures how easily points can be separated by randomly splitting the data into smaller and smaller regions.
- 4
Comparing performance
You've now been introduced to a few different algorithms for anomaly scoring. In this final chapter, you'll learn to compare the detection performance of the algorithms in instances where labeled anomalies are available. You'll learn to calculate and interpret the precision and recall statistics for an anomaly score, and how to adapt the algorithms so they can accommodate data with categorical features.
Labeled anomalies50 xpThyroid data100 xpVisualizing thyroid disease100 xpAnomaly score100 xpMeasuring performance50 xpBinarized scores100 xpCross-tabulate binary scores100 xpThyroid precision and recall100 xpWorking with categorical features50 xpConverting character to factor100 xpIsolation forest with factors100 xpLOF with factors100 xpWrap-up50 xp
Para Empresas
Treinar 2 ou mais pessoas?
Obtenha acesso à biblioteca completa do DataCamp, com relatórios, atribuições, projetos e muito mais centralizadoscolaboradores
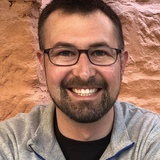
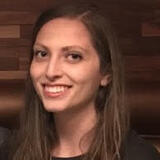
pré-requisitos
Intermediate RDataCamp Content Creator
Ver MaisCourse Instructor
O que os outros alunos têm a dizer?
Junte-se a mais de 15 milhões de alunos e comece Introduction to Anomaly Detection in R hoje mesmo!
Crie sua conta gratuita
ou
Ao continuar, você aceita nossos Termos de Uso, nossa Política de Privacidade e que seus dados são armazenados nos EUA.