Introduction to Predictive Analytics in Python
In this course you'll learn to use and present logistic regression models for making predictions.
Comece O Curso Gratuitamente4 horas14 vídeos52 exercícios
Crie sua conta gratuita
ou
Ao continuar, você aceita nossos Termos de Uso, nossa Política de Privacidade e que seus dados são armazenados nos EUA.Treinar 2 ou mais pessoas?Experimente o DataCamp For Business
Amado por alunos de milhares de empresas
Descrição do Curso
In this course, you will learn how to build a logistic regression model with meaningful variables. You will also learn how to use this model to make predictions and how to present it and its performance to business stakeholders.
Para Empresas
Treinar 2 ou mais pessoas?
Obtenha acesso à biblioteca completa do DataCamp, com relatórios, atribuições, projetos e muito mais centralizados- 1
Building Logistic Regression Models
GratuitoIn this Chapter, you'll learn the basics of logistic regression: how can you predict a binary target with continuous variables and, how should you interpret this model and use it to make predictions for new examples?
Introduction and base table structure50 xpStructure of the base table50 xpExploring the base table100 xpExploring the predictive variables100 xpLogistic regression50 xpInterpretation of coefficients50 xpBuilding a logistic regression model100 xpShowing the coefficients and intercept100 xpUsing the logistic regression model50 xpMaking predictions100 xpDonor that is most likely to donate100 xp - 2
Forward stepwise variable selection for logistic regression
In this chapter you'll learn why variable selection is crucial for building a useful model. You'll also learn how to implement forward stepwise variable selection for logistic regression and how to decide on the number of variables to include in your final model.
Motivation for variable selection50 xpWhich model is best?50 xpCalculating AUC100 xpUsing different sets of variables100 xpForward stepwise variable selection50 xpSelecting the next best variable100 xpFinding the order of variables100 xpCorrelated variables100 xpDeciding on the number of variables50 xpPartitioning100 xpEvaluating a model on test and train100 xpBuilding the AUC curves100 xpDeciding the cut-off50 xp - 3
Explaining model performance to business
Now that you know how to build a good model, you should convince stakeholders to use it by creating appropriate graphs. You will learn how to construct and interpret the cumulative gains curve and lift graph.
The cumulative gains curve50 xpInterpreting the cumulative gains curve50 xpConstructing the cumulative gains curve100 xpA random model100 xpThe lift curve50 xpInterpreting the lift curve50 xpConstructing the lift curve100 xpA perfect model100 xpGuiding business to better decisions50 xpTargeting using cumulative gains curve50 xpBusiness case using lift curve100 xpBusiness case using cumulative gains curve100 xp - 4
Interpreting and explaining models
In a business context, it is often important to explain the intuition behind the model you built. Indeed, if the model and its variables do not make sense, the model might not be used. In this chapter you'll learn how to explain the relationship between the variables in the model and the target by means of predictor insight graphs.
Predictor insight graphs50 xpInterpretation of predictor insight graphs50 xpRetrieving information from the predictor insight table100 xpDiscretization of continuous variables50 xpDiscretization of a certain variable100 xpDiscretizing all variables100 xpMaking clean cuts100 xpPreparing the predictor insight graph table50 xpCalculating average incidences100 xpConstructing the predictor insight graph table100 xpGrouping all predictor insight graph tables100 xpPlotting the predictor insight graph50 xpPlotting the incidences100 xpPlotting the group sizes100 xpPutting it all together100 xpSummary50 xp
Para Empresas
Treinar 2 ou mais pessoas?
Obtenha acesso à biblioteca completa do DataCamp, com relatórios, atribuições, projetos e muito mais centralizadosconjuntos de dados
Example basetablecolaboradores
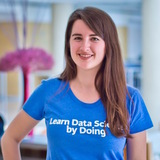
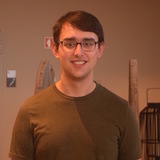
pré-requisitos
Intermediate PythonNele Verbiest
Ver MaisData Scientist at Python Predictions
O que os outros alunos têm a dizer?
Junte-se a mais de 14 milhões de alunos e comece Introduction to Predictive Analytics in Python hoje mesmo!
Crie sua conta gratuita
ou
Ao continuar, você aceita nossos Termos de Uso, nossa Política de Privacidade e que seus dados são armazenados nos EUA.