Dealing With Missing Data in R
Make it easy to visualize, explore, and impute missing data with naniar, a tidyverse friendly approach to missing data.
Comienza El Curso Gratis4 horas14 vídeos52 ejercicios15.173 aprendicesDeclaración de cumplimiento
Crea Tu Cuenta Gratuita
o
Al continuar, acepta nuestros Términos de uso, nuestra Política de privacidad y que sus datos se almacenan en los EE. UU.¿Entrenar a 2 o más personas?
Probar DataCamp for BusinessPreferido por estudiantes en miles de empresas
Descripción del curso
Missing data is part of any real world data analysis. It can crop up in unexpected places, making analyses challenging to understand. In this course, you will learn how to use tidyverse tools and the naniar R package to visualize missing values. You'll tidy missing values so they can be used in analysis and explore missing values to find bias in the data. Lastly, you'll reveal other underlying patterns of missingness. You will also learn how to "fill in the blanks" of missing values with imputation models, and how to visualize, assess, and make decisions based on these imputed datasets.
¿Entrenar a 2 o más personas?
Obtén a tu equipo acceso a la plataforma DataCamp completa, incluidas todas las funciones.En las siguientes pistas
Caja de herramientas intermedia de Tidyverse
Ir a la pista- 1
Why care about missing data?
GratuitoChapter 1 introduces you to missing data, explaining what missing values are, their behavior in R, how to detect them, and how to count them. We then introduce missing data summaries and how to summarise missingness across cases, variables, and how to explore across groups within the data. Finally, we discuss missing data visualizations, how to produce overview visualizations for the entire dataset and over variables, cases, and other summaries, and how to explore these across groups.
Introduction to missing data50 xpUsing and finding missing values100 xpHow many missing values are there?100 xpWorking with missing values50 xpWhy care about missing values?50 xpSummarizing missingness100 xpTabulating Missingness100 xpOther summaries of missingness100 xpHow do we visualize missing values?50 xpYour first missing data visualizations100 xpVisualizing missing cases and variables100 xpVisualizing missingness patterns100 xp - 2
Wrangling and tidying up missing values
In chapter two, you will learn how to uncover hidden missing values like "missing" or "N/A" and replace them with `NA`. You will learn how to efficiently handle implicit missing values - those values implied to be missing, but not explicitly listed. We also cover how to explore missing data dependence, discussing Missing Completely at Random (MCAR), Missing At Random (MAR), Missing Not At Random (MNAR), and what they mean for your data analysis.
Searching for and replacing missing values50 xpUsing miss_scan_count100 xpUsing replace_with_na100 xpUsing replace_with_na scoped variants100 xpFilling down missing values50 xpFix implicit missings using complete()100 xpFix explicit missings using fill()100 xpUsing complete() and fill() together100 xpMissing Data dependence50 xpDifferences between MCAR and MAR50 xpExploring missingness dependence100 xpFurther exploring missingness dependence50 xp - 3
Testing missing relationships
In this chapter, you will learn about workflows for working with missing data. We introduce special data structures, the shadow matrix, and nabular data, and demonstrate how to use them in workflows for exploring missing data so that you can link summaries of missingness back to values in the data. You will learn how to use ggplot to explore and visualize how values changes as other variables go missing. Finally, you learn how to visualize missingness across two variables, and how and why to visualize missings in a scatterplot.
Tools to explore missing data dependence50 xpCreating shadow matrix data100 xpPerforming grouped summaries of missingness100 xpFurther exploring more combinations of missingness100 xpVisualizing missingness across one variable50 xpNabular data and filling by missingness100 xpNabular data and summarising by missingness100 xpExplore variation by missingness: box plots100 xpVisualizing missingness across two variables50 xpExploring missing data with scatter plots100 xpUsing facets to explore missingness100 xpFaceting to explore missingness (multiple plots)100 xp - 4
Connecting the dots (Imputation)
In this chapter, you will learn about filling in the missing values in your data, which is called imputation. You will learn how to impute and track missing values, and what the good and bad features of imputations are so that you can explore, visualise, and evaluate the imputed data against the original values. You will learn how to use, evaluate, and compare different imputation models, and explore how different imputation models affect the inferences you can draw from the models.
Filling in the blanks50 xpImpute data below range with nabular data100 xpVisualize imputed values in a scatter plot100 xpCreate histogram of imputed data100 xpWhat makes a good imputation50 xpEvaluating bad imputations100 xpEvaluating imputations: The scale100 xpEvaluating imputations: Across many variables100 xpPerforming imputations50 xpUsing simputation to impute data100 xpEvaluating and comparing imputations100 xpEvaluating imputations (many models & variables)100 xpEvaluating imputations and models50 xpCombining and comparing many imputation models100 xpEvaluating the different parameters in the model100 xpFinal Lesson50 xp
¿Entrenar a 2 o más personas?
Obtén a tu equipo acceso a la plataforma DataCamp completa, incluidas todas las funciones.En las siguientes pistas
Caja de herramientas intermedia de Tidyverse
Ir a la pistacolaboradores
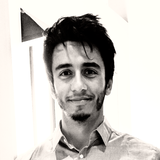
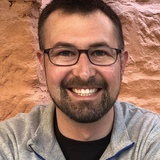
¿Qué tienen que decir otros alumnos?
¡Únete a 15 millones de estudiantes y empieza Dealing With Missing Data in R hoy mismo!
Crea Tu Cuenta Gratuita
o
Al continuar, acepta nuestros Términos de uso, nuestra Política de privacidad y que sus datos se almacenan en los EE. UU.