Natural Language Processing with spaCy
Master the core operations of spaCy and train models for natural language processing. Extract information from unstructured data and match patterns.
Comience El Curso Gratis4 Horas15 Videos53 Ejercicios
Crea Tu Cuenta Gratuita
o
Al continuar, acepta nuestros Términos de uso, nuestra Política de privacidad y que sus datos se almacenan en los EE. UU.¿Entrenar a 2 o más personas?Pruebe DataCamp para empresas
Preferido por estudiantes en miles de empresas
Descripción del curso
Meet spaCy, an Industry-Standard for NLP
In this course, you will learn how to use spaCy, a fast-growing industry-standard library, to perform various natural language processing tasks such as tokenization, sentence segmentation, parsing, and named entity recognition. spaCy can provide powerful, easy-to-use, and production-ready features across a wide range of natural language processing tasks.Learn the Core Operations of spaCy
You will start by learning the core operations of spaCy and how to use them to parse text and extract information from unstructured data. Then, you will work with spaCy’s classes, such as Doc, Span, and Token, and learn how to use different spaCy components for calculating word vectors and predicting semantic similarity.Train spaCy Models and Learn About Pattern Matching
You will practice writing simple and complex matching patterns to extract given terms and phrases using EntityRuler, Matcher, and PhraseMatcher from unstructured data. You will also learn how to create custom pipeline components and create training/evaluation data. From there, you will dive into training spaCy models and how to use them for inference. Throughout the course, you will work on real-world examples and solidify your understanding of using spaCy in your own NLP projects.Empresas
¿Entrenar a 2 o más personas?
Obtenga acceso de su equipo a la biblioteca completa de DataCamp, con informes centralizados, tareas, proyectos y másEn las siguientes pistas
Científico de Machine Learning con Python
Ir a la pistaProcesamiento del Lenguaje Natural en Python
Ir a la pista- 1
Introduction to NLP and spaCy
GratuitoThis chapter will introduce you to NLP, some of its use cases such as named-entity recognition and AI-powered chatbots. You’ll learn how to use the powerful spaCy library to perform various natural language processing tasks such as tokenization, sentence segmentation, POS tagging, and named entity recognition.
Natural Language Processing (NLP) basics50 xpDoc container in spaCy100 xpNER use case50 xpTokenization with spaCy100 xpspaCy basics50 xpRunning a spaCy pipeline100 xpLemmatization with spaCy100 xpSentence segmentation with spaCy100 xpLinguistic features in spaCy50 xpPOS tagging with spaCy100 xpNER with spaCy100 xpText processing with spaCy100 xp - 2
spaCy Linguistic Annotations and Word Vectors
Learn about linguistic features, word vectors, semantic similarity, analogies, and word vector operations. In this chapter you’ll discover how to use spaCy to extract word vectors, categorize texts that are relevant to a given topic and find semantically similar terms to given words from a corpus or from a spaCy model vocabulary.
Linguistic features50 xpLinguistic annotations in spaCy100 xpWord-sense disambiguation with spaCy100 xpDependency parsing with spaCy100 xpIntroduction to word vectors50 xpspaCy vocabulary100 xpWord vectors in spaCy vocabulary100 xpWord vectors and spaCy50 xpAnalogies and vector operations100 xpWord vectors projection100 xpSimilar words in a vocabulary100 xpMeasuring semantic similarity with spaCy50 xpDoc similarity with spaCy100 xpSpan similarity with spaCy100 xpSemantic similarity for categorizing text100 xp - 3
Data Analysis with spaCy
Get familiar with spaCy pipeline components, how to add a pipeline component, and analyze the NLP pipeline. You will also learn about multiple approaches for rule-based information extraction using EntityRuler, Matcher, and PhraseMatcher classes in spaCy and RegEx Python package.
spaCy pipelines50 xpAdding pipes in spaCy100 xpAnalyzing pipelines in spaCy100 xpspaCy EntityRuler50 xpEntityRuler with blank spaCy model100 xpEntityRuler for NER100 xpEntityRuler with multi-patterns in spaCy100 xpRegEx with spaCy50 xpRegEx in Python100 xpRegEx with EntityRuler in spaCy100 xpspaCy Matcher and PhraseMatcher50 xpMatching a single term in spaCy100 xpPhraseMatcher in spaCy100 xpMatching with extended syntax in spaCy100 xp - 4
Customizing spaCy Models
Explore multiple real-world use cases where spaCy models may fail and learn how to train them further to improve model performance. You’ll be introduced to spaCy training steps and understand how to train an existing spaCy model or from scratch, and evaluate the model at the inference time.
Customizing spaCy models50 xpTraining spaCy models50 xpModel performance on your data100 xpspaCy training data format50 xpTraining steps100 xpAnnotation and preparing training data100 xpCompatible training data100 xpTraining with spaCy50 xpTraining preparation steps100 xpTrain an existing NER model100 xpTraining a spaCy model from scratch100 xpWrap-up50 xp
Empresas
¿Entrenar a 2 o más personas?
Obtenga acceso de su equipo a la biblioteca completa de DataCamp, con informes centralizados, tareas, proyectos y másEn las siguientes pistas
Científico de Machine Learning con Python
Ir a la pistaProcesamiento del Lenguaje Natural en Python
Ir a la pistaSets De Datos
corona.jsonColaboradores
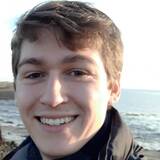
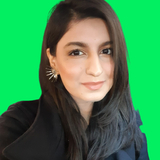
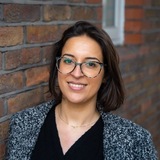
Azadeh Mobasher
Ver MasPrincipal Data Scientist
¿Qué tienen que decir otros alumnos?
¡Únete a 13 millones de estudiantes y empieza Natural Language Processing with spaCy hoy mismo!
Crea Tu Cuenta Gratuita
o
Al continuar, acepta nuestros Términos de uso, nuestra Política de privacidad y que sus datos se almacenan en los EE. UU.