Fraud Detection in R
Learn to detect fraud with analytics in R.
Comienza El Curso Gratis4 horas16 vídeos49 ejercicios6978 aprendicesDeclaración de cumplimiento
Crea Tu Cuenta Gratuita
o
Al continuar, acepta nuestros Términos de uso, nuestra Política de privacidad y que sus datos se almacenan en los EE. UU.¿Entrenar a 2 o más personas?
Probar DataCamp for BusinessPreferido por estudiantes en miles de empresas
Descripción del curso
The Association of Certified Fraud Examiners estimates that fraud costs organizations worldwide $3.7 trillion a year and that a typical company loses five percent of annual revenue due to fraud. Fraud attempts are expected to even increase further in future, making fraud detection highly necessary in most industries. This course will show how learning fraud patterns from historical data can be used to fight fraud. Some techniques from robust statistics and digit analysis are presented to detect unusual observations that are likely associated with fraud. Two main challenges when building a supervised tool for fraud detection are the imbalance or skewness of the data and the various costs for different types of misclassification. We present techniques to solve these issues and focus on artificial and real datasets from a wide variety of fraud applications.
¿Entrenar a 2 o más personas?
Obtén a tu equipo acceso a la plataforma DataCamp completa, incluidas todas las funciones.- 1
Introduction & Motivation
GratuitoThis chapter will first give a formal definition of fraud. You will then learn how to detect anomalies in the type of payment methods used or the time these payments are made to flag suspicious transactions.
Introduction & Motivation50 xpImbalanced class distribution100 xpCost of not detecting fraud100 xpTime features50 xpCircular histogram100 xpSuspicious timestamps100 xpFrequency features50 xpFrequency feature for one account100 xpFrequency feature for multiple accounts100 xpRecency features50 xpRecency feature100 xpComparing frequency & recency100 xp - 2
Social network analytics
In the second chapter, you will learn how to use networks to fight fraud. You will visualize networks and use a sociology concept called homophily to detect fraudulent transactions and catch fraudsters.
Social network analytics50 xpAnalyzing a network100 xpOverlapping edges100 xpFraud and social network analysis50 xpLooking for homophily in a network100 xpVisualizing node attributes100 xpSocial network based inference50 xpRelational vs non-relational models50 xpRelational neighbor classifier100 xpSocial network metrics50 xpDegree, closeness & betweenness100 xpAdding network features100 xp - 3
Imbalanced class distributions
Fortunately, fraud occurrences are rare. However, this means that you're working with imbalanced data, which if left as is will bias your detection models. In this chapter, you will tackle imbalance using over and under-sampling methods.
Dealing with imbalanced datasets50 xpHow to deal with class imbalance?50 xpVisualizing patterns in the data100 xpRandom over-sampling100 xpRandom under-sampling50 xpShrinking the majority group100 xpCombining ROS & RUS100 xpSynthetic Over-sampling50 xpHave you met SMOTE?50 xpSMOTE100 xpFrom dataset to detection model50 xpBuild your own detection model100 xpTrue cost of fraud detection100 xp - 4
Digit analysis and robust statistics
In this final chapter, you will learn about a surprising mathematical law used to detect suspicious occurrences. You will then use robust statistics to make your models even more bulletproof.
Digit analysis using Benford's law50 xpBenford's Law for first digit100 xpConformity of census data100 xpBenford's Law for fraud detection50 xpConformity to Benford's Law50 xpFire insurance claims100 xpPayments data set100 xpDetecting univariate outliers50 xpComputing robust z-scores100 xpBoxplot100 xpDetecting multivariate outliers50 xpMultivariate outlier detection100 xp
¿Entrenar a 2 o más personas?
Obtén a tu equipo acceso a la plataforma DataCamp completa, incluidas todas las funciones.colaboradores
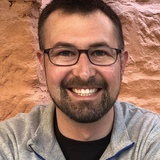
¿Qué tienen que decir otros alumnos?
¡Únete a 15 millones de estudiantes y empieza Fraud Detection in R hoy mismo!
Crea Tu Cuenta Gratuita
o
Al continuar, acepta nuestros Términos de uso, nuestra Política de privacidad y que sus datos se almacenan en los EE. UU.